pytorch中的sequential
时间: 2023-11-12 12:06:44 浏览: 30
Sequential是PyTorch中的一个容器,用于构建神经网络模型。它可以将多个层连接起来,形成一个序列式的网络结构。
通过Sequential,我们可以简单地构建一个深层神经网络,而无需手动指定每一层的输入和输出大小。我们只需要将每一层的构建函数作为参数传入Sequential即可,PyTorch会自动将它们按照顺序连接起来。
例如,下面的代码使用Sequential构建了一个两层的全连接神经网络:
```
import torch.nn as nn
model = nn.Sequential(
nn.Linear(784, 256),
nn.ReLU(),
nn.Linear(256, 10)
)
```
这个模型首先将大小为784的输入向量经过一个线性变换后得到大小为256的隐藏层,然后经过ReLU激活函数后得到激活值,最后再经过一个线性变换得到大小为10的输出向量。整个模型的结构就是一个串联的线性变换和非线性变换。
Sequential还可以用于构建更复杂的网络结构,例如多分支网络、循环神经网络等。
相关问题
pytorch中sequential
在PyTorch中,`torch.nn.Sequential`是一个顺序容器,它按照在构造函数中传递的顺序包含其他模块,并将它们作为一个模块。这意味着,当您将输入传递给Sequential时,它将按照与在构造函数中传递的顺序相同的顺序依次运行每个模块,并将每个模块的输出用作下一个模块的输入。以下是一个简单的例子:
```python
import torch.nn as nn
# 定义一个Sequential容器
model = nn.Sequential(
nn.Linear(10, 5),
nn.ReLU(), nn.Linear(5, 1)
)
# 将输入传递给Sequential
x = torch.randn(1, 10)
output = model(x)
```
在这个例子中,我们定义了一个Sequential容器,它包含两个线性层和一个ReLU激活函数。当我们将输入`x`传递给Sequential时,它将首先通过第一个线性层,然后通过ReLU激活函数,最后通过第二个线性层,并返回输出。
除了上面的例子,您还可以使用`add_module`方法向Sequential容器中添加模块,如下所示:
```python
import torch.nn as nn
# 定义一个Sequential容器
model = nn.Sequential()
# 向Sequential容器中添加模块
model.add_module('linear1', nn.Linear(10, 5))
model.add_module('relu', nn.ReLU())
model.add_module('linear2', nn.Linear(5, 1))
# 将输入传递给Sequential
x = torch.randn(1, 10)
output = model(x)
```
这个例子与上面的例子相同,只是我们使用了`add_module`方法向Sequential容器中添加模块。
pytorch Sequential
PyTorch的Sequential模块可以用来按照顺序堆叠各种神经网络模块。它有两种常见的构造方式。第一种方式是使用OrderedDict来定义网络结构,其中每个模块的名称和顺序都可以显式地指定。这种方式可以通过调用`torch.nn.Sequential(arg:OrderedDict[str,Module])`来实现。
第二种方式是使用可变数量的参数来定义网络结构,其中每个参数都是一个神经网络模块。这种方式可以通过调用`torch.nn.Sequential(*args:Module)`来实现。
在Sequential模块中,可以使用多种PyTorch提供的函数和方法来操作张量。例如,`torch.ones()`用于创建一个全为1的张量,`torch.add()`用于对两个张量进行相加,`torch.zeros()`用于创建一个全为0的张量,`torch.squeeze()`用于压缩张量的维度。这些函数和方法可以在构建和训练神经网络时提供便利。
相关推荐
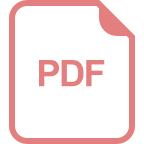
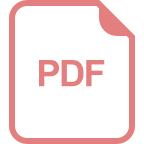












