import numpy as np import matplotlib.pyplot as plt from keras.models import Sequential from keras.layers import Dense, Flatten, Conv1D, MaxPooling1D # 生成正弦函数数据 x = np.linspace(0, 100, 1000) y = np.sin(x) # 将数据转换为卷积神经网络需要的格式 X = np.zeros((len(x), 10)) for i in range(len(x)): for j in range(10): X[i][j] = y[(i+j)%len(x)] X = np.reshape(X, (X.shape[0], X.shape[1], 1)) # 构建卷积神经网络模型 model = Sequential() model.add(Conv1D(filters=32, kernel_size=3, activation='relu', input_shape=(10,1))) model.add(MaxPooling1D(pool_size=2)) model.add(Flatten()) model.add(Dense(100, activation='relu')) model.add(Dense(1, activation='linear')) # 编译模型并训练 model.compile(loss='mse', optimizer='adam') model.fit(X, y, epochs=100, batch_size=32) # 预测并可视化结果 y_pred = model.predict(X) plt.plot(x, y, label='true') plt.plot(x, y_pred, label='predict') plt.legend() plt.show()具体解释这段代码每一步的用意和作用
时间: 2024-02-25 19:52:47 浏览: 19
这段代码演示了如何使用 Keras 构建一个简单的卷积神经网络来预测正弦函数。下面是每一步的用意和作用:
1. 导入所需的库
```python
import numpy as np
import matplotlib.pyplot as plt
from keras.models import Sequential
from keras.layers import Dense, Flatten, Conv1D, MaxPooling1D
```
这些库包括 NumPy 用于生成数据,Matplotlib 用于可视化结果,以及 Keras 用于构建卷积神经网络模型。
2. 生成正弦函数数据
```python
x = np.linspace(0, 100, 1000)
y = np.sin(x)
```
在 x 轴上生成 1000 个等距的数字,并将它们作为输入 x,然后计算对应的正弦值,作为输出 y。
3. 将数据转换为卷积神经网络需要的格式
```python
X = np.zeros((len(x), 10))
for i in range(len(x)):
for j in range(10):
X[i][j] = y[(i+j)%len(x)]
X = np.reshape(X, (X.shape[0], X.shape[1], 1))
```
由于卷积神经网络需要一个三维数组作为输入,这里将原始的输入和输出数据转换成了一个 10 列的矩阵,其中每一列是原始输出数据的一个偏移版本。这个矩阵的大小为 (1000, 10, 1)。
4. 构建卷积神经网络模型
```python
model = Sequential()
model.add(Conv1D(filters=32, kernel_size=3, activation='relu', input_shape=(10,1)))
model.add(MaxPooling1D(pool_size=2))
model.add(Flatten())
model.add(Dense(100, activation='relu'))
model.add(Dense(1, activation='linear'))
```
这个模型包含一个卷积层、一个最大池化层、一个扁平化层、一个全连接层和一个输出层。卷积层有 32 个过滤器,每个过滤器的大小为 3,并使用 ReLU 激活函数。最大池化层的池化大小为 2。扁平化层将输入展平成一个向量。全连接层有 100 个神经元,并使用 ReLU 激活函数。输出层只有一个神经元,并使用线性激活函数。
5. 编译模型并训练
```python
model.compile(loss='mse', optimizer='adam')
model.fit(X, y, epochs=100, batch_size=32)
```
使用均方误差作为损失函数,使用 Adam 优化器编译模型。然后使用训练数据 X 和 y 训练模型,进行 100 个 epoch,每个 epoch 中使用 32 个样本。
6. 预测并可视化结果
```python
y_pred = model.predict(X)
plt.plot(x, y, label='true')
plt.plot(x, y_pred, label='predict')
plt.legend()
plt.show()
```
使用训练好的模型对输入数据进行预测,然后将预测结果与真实值可视化在同一个图中,以便比较它们的差异。
相关推荐
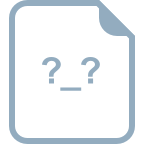
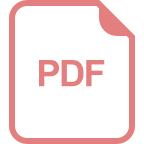






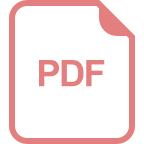
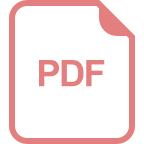
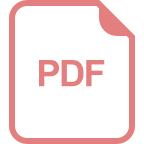
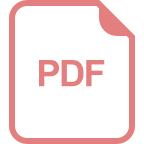
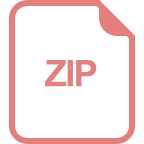
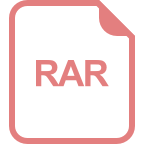
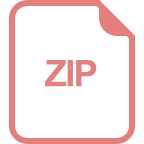