import torch import torch.nn as nn import torch.nn.functional as F from torch.autograd import Variable class Bottleneck(nn.Module): def init(self, last_planes, in_planes, out_planes, dense_depth, stride, first_layer): super(Bottleneck, self).init() self.out_planes = out_planes self.dense_depth = dense_depth self.conv1 = nn.Conv2d(last_planes, in_planes, kernel_size=1, bias=False) self.bn1 = nn.BatchNorm2d(in_planes) self.conv2 = nn.Conv2d(in_planes, in_planes, kernel_size=3, stride=stride, padding=1, groups=32, bias=False) self.bn2 = nn.BatchNorm2d(in_planes) self.conv3 = nn.Conv2d(in_planes, out_planes+dense_depth, kernel_size=1, bias=False) self.bn3 = nn.BatchNorm2d(out_planes+dense_depth) self.shortcut = nn.Sequential() if first_layer: self.shortcut = nn.Sequential( nn.Conv2d(last_planes, out_planes+dense_depth, kernel_size=1, stride=stride, bias=False), nn.BatchNorm2d(out_planes+dense_depth) ) def forward(self, x): out = F.relu(self.bn1(self.conv1(x))) out = F.relu(self.bn2(self.conv2(out))) out = self.bn3(self.conv3(out)) x = self.shortcut(x) d = self.out_planes out = torch.cat([x[:,:d,:,:]+out[:,:d,:,:], x[:,d:,:,:], out[:,d:,:,:]], 1) out = F.relu(out) return out class DPN(nn.Module): def init(self, cfg): super(DPN, self).init() in_planes, out_planes = cfg['in_planes'], cfg['out_planes'] num_blocks, dense_depth = cfg['num_blocks'], cfg['dense_depth'] self.conv1 = nn.Conv2d(3, 64, kernel_size=3, stride=1, padding=1, bias=False) self.bn1 = nn.BatchNorm2d(64) self.last_planes = 64 self.layer1 = self._make_layer(in_planes[0], out_planes[0], num_blocks[0], dense_depth[0], stride=1) self.layer2 = self._make_layer(in_planes[1], out_planes[1], num_blocks[1], dense_depth[1], stride=2) self.layer3 = self._make_layer(in_planes[2], out_planes[2], num_blocks[2], dense_depth[2], stride=2) self.layer4 = self._make_layer(in_planes[3], out_planes[3], num_blocks[3], dense_depth[3], stride=2) self.linear = nn.Linear(out_planes[3]+(num_blocks[3]+1)dense_depth[3], 10) def _make_layer(self, in_planes, out_planes, num_blocks, dense_depth, stride): strides = [stride] + 1 layers = [] for i,stride in (strides): layers.append(Bottleneck(self.last_planes, in_planes, out_planes, dense_depth, stride, i==0)) self.last_planes = out_planes + (i+2) * dense_depth return nn.Sequential(*layers) def forward(self, x): out = F.relu(self.bn1(self.conv1(x))) out = self.layer1(out) out = self.layer2(out) out = self.layer3(out) out = self.layer4(out) out = F.avg_pool2d(out, 4) out = out.view(out.size(0), -1) out = self.linear(out) return out def DPN92(): cfg = { 'in_planes': (96,192,384,768), 'out_planes': (256,512,1024,2048), 'num_blocks': (3,4,20,3), 'dense_depth': (16,32,24,128) } return DPN(cfg)基于这个程序修改成对摄像头采集的图像检测与分类输出坐标、大小和种类,并训练和测试
时间: 2023-06-10 19:06:24 浏览: 145
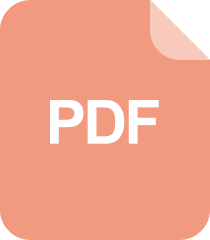
浅谈pytorch中torch.max和F.softmax函数的维度解释
这段代码是一个深度残差网络DPN的实现,用于图像分类任务。如果你想基于这个程序修改成对摄像头采集的图像检测与分类输出坐标、大小和种类,并训练和测试,需要进行以下步骤:
1. 定义你的自定义数据集。
2. 修改模型的最后一层,将输出类别数改为你的数据集的类别数,并根据你的需求修改输出层的结构,包括坐标和大小。
3. 定义训练和测试函数,并将数据集传递给模型进行训练和测试。
以下是一个简单的示例代码,用于在摄像头采集的图像上进行目标检测和分类,输出目标类别、坐标和大小:
```
import cv2
import torch
import torch.nn as nn
import torchvision.transforms as transforms
from model import DPN92
# 定义数据集类别数
num_classes = 2
# 定义模型
model = DPN92()
model.linear = nn.Linear(model.cfg['out_planes'][3] * (model.cfg['num_blocks'][3] + 1) * model.cfg['dense_depth'][3], num_classes * 5)
# 加载训练好的模型
model.load_state_dict(torch.load('model.pth'))
# 定义图像预处理函数
transform = transforms.Compose([
transforms.ToPILImage(),
transforms.Resize((224, 224)),
transforms.ToTensor(),
])
# 定义摄像头捕捉
cap = cv2.VideoCapture(0)
while True:
ret, frame = cap.read()
# 预处理图像
img = transform(frame).unsqueeze(0)
# 将图像输入模型进行预测
output = model(img)
# 解析输出结果
output = output.detach().numpy()[0]
classes = output[::5]
coords = output[1:]
# 显示结果
for cls, coord in zip(classes, coords.reshape(-1, 4)):
if cls != 0:
x, y, w, h = coord.astype(int)
cv2.rectangle(frame, (x, y), (x+w, y+h), (0, 255, 0), 2)
cv2.putText(frame, str(cls), (x, y), cv2.FONT_HERSHEY_SIMPLEX, 1, (0, 0, 255), 2)
# 显示图像
cv2.imshow('frame', frame)
# 退出
if cv2.waitKey(1) == ord('q'):
break
# 释放摄像头
cap.release()
# 关闭窗口
cv2.destroyAllWindows()
```
需要注意的是,这只是一个简单的示例代码,需要根据你的具体需求进行修改。例如,如果你的数据集包含多个目标,需要对每个目标进行坐标和大小的预测,并在图像上进行显示。
阅读全文
相关推荐
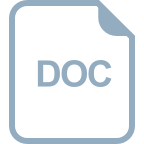
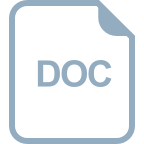










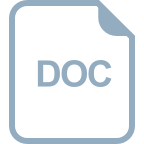
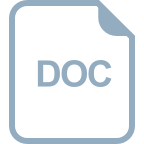