The starting configuration of this puzzle is a row of cells, with disks located on cells through . The goal is to move the disks to the end of the row using a constrained set of actions. At each step, a disk can only be moved to an adjacent empty cell, or to an empty cell two spaces away if another disk is located on the intervening square. Given these restrictions, it can be seen that in many cases, no movements will be possible for the majority of the disks. For example, from the starting position, the only two options are to move the last disk from cell to cell , or to move the second-to-last disk from cell to cell . 1. [15 points] Write a function solve_identical_disks(length, n) that returns an optimal solution to the above problem as a list of moves, where length is the number of cells in the row and n is the number of disks. Each move in the solution should be a twoelement tuple of the form (from, to) indicating a disk movement from the cell from to the cell to. As suggested by its name, this function should treat all disks as being identical. Your solver for this problem should be implemented using a breadth-first graph search. The exact solution produced is not important, as long as it is of minimal length. Unlike in the previous two sections, no requirement is made with regards to the manner in which puzzle configurations are represented. Before you begin, think carefully about which data structures might be best suited for the problem, as this choice may affect the efficiency of your search
时间: 2024-02-14 07:09:14 浏览: 31
Here's a possible implementation of the `solve_identical_disks` function using breadth-first graph search:
```python
from collections import deque
def solve_identical_disks(length, n):
# Initialize the starting configuration
start = [True] * n + [False] * (length - n)
# Define a function to generate all possible successor configurations
def successors(config):
for i in range(length):
if not config[i]:
if i + 1 < length and config[i + 1]:
# Move disk to adjacent empty cell
yield config[:i] + [True] + [False] + config[i + 2:]
elif i + 2 < length and config[i + 2]:
# Move disk to empty cell two spaces away
yield config[:i] + [True] + config[i + 2:i + 3] + [False] + config[i + 3:]
# Perform breadth-first graph search to find the goal configuration
queue = deque([(start, [])])
visited = set([tuple(start)])
while queue:
config, moves = queue.popleft()
if config == [False] * (length - n) + [True] * n:
return moves
for successor in successors(config):
if tuple(successor) not in visited:
queue.append((successor, moves + [(config.index(True), successor.index(True))]))
visited.add(tuple(successor))
# If the goal configuration is not reachable, return None
return None
```
The `start` variable represents the starting configuration as a list of `True` values for the cells occupied by disks and `False` values for the empty cells. The `successors` function generates all possible successor configurations for a given configuration by moving a disk to an adjacent empty cell or to an empty cell two spaces away. The `queue` variable is used to store the configurations to be explored, along with the list of moves required to reach them. The `visited` set is used to keep track of the configurations that have already been explored, in order to avoid revisiting them.
The function returns the list of moves required to reach the goal configuration, which is represented as a list of `False` values for the cells before the disks and `True` values for the cells occupied by the disks. If the goal configuration is not reachable, the function returns `None`.
相关推荐
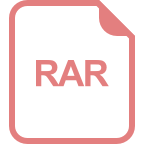
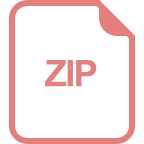














