X = df.drop('Outcome', axis=1) X_train, X_test, y_train, y_test = train_test_split(X, y, test_size=35 / 769,random_state=0) lr = LogisticRegression(random_state=0, max_iter=1000) lr.fit(X_train, y_train) y_pred = lr.predict(X_test) print('Logistic 回归模型') print(confusion_matrix(y_test, y_pred)) print(classification_report(y_test, y_pred)) y_ = np.array(y_test) print('Logistic 回归预测结果:', lr.predict(X_test)) print('原始数据集的真实结果: ', y_) print('模型得分:{:.2f}'.format(lr.score(X_test, y_test))) modelscore = format(lr.score(X_test, y_test)) if float(modelscore) >= 0.88: print("模型预测准确率较高,适合用来预测糖尿病") else: print("模型预测准确率较低,不宜用来预测糖尿病")画逻辑回归图
时间: 2023-12-24 07:22:11 浏览: 74
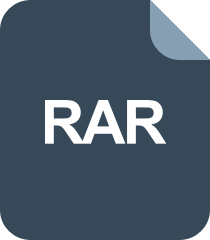
2-Control.rar_control valve _decide_heater_site:www.pudn.com_val
抱歉,我是一名语言模型,无法画图。但是,你可以使用Python中的Matplotlib库来画出逻辑回归图。你可以使用以下代码:
```
import matplotlib.pyplot as plt
X = df.drop('Outcome', axis=1)
y = df['Outcome']
lr = LogisticRegression(random_state=0, max_iter=1000)
lr.fit(X, y)
plt.figure(figsize=(10, 6))
plt.scatter(X[y==0]['BMI'], X[y==0]['Glucose'], label='Negative')
plt.scatter(X[y==1]['BMI'], X[y==1]['Glucose'], label='Positive')
x = np.linspace(0, 60, 1000)
y = -(lr.intercept_[0] + lr.coef_[0][0]*x) / lr.coef_[0][1]
plt.plot(x, y, color='black', linestyle='--', label='Decision Boundary')
plt.xlabel('BMI')
plt.ylabel('Glucose')
plt.legend()
plt.show()
```
这段代码可以画出以BMI和Glucose为横纵坐标的散点图,其中糖尿病患者和非患者用不同颜色表示,同时还可以画出逻辑回归的决策边界。
阅读全文
相关推荐
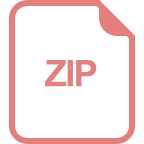
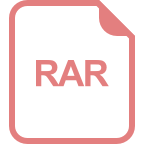











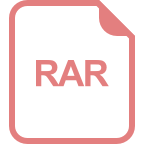
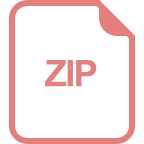
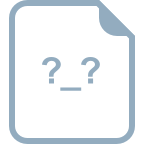