self.minimize_button.setStyleSheet('color: {}'.format(Qt.white.name())) TypeError: 'bytes' object is not callable
时间: 2024-03-23 16:37:01 浏览: 149
这个错误通常是由于将字节对象视为函数而引起的。你可能已经将 `Qt.white` 用作函数来调用,而实际上它只是一个颜色常量。为了避免这种错误,你可以尝试将 `Qt.white` 直接传递给 `setStyleSheet()` 方法,而不是将其转换为字符串。以下是一个示例代码:
```python
from PySide2.QtCore import Qt
from PySide2.QtWidgets import QApplication, QPushButton
app = QApplication([])
button = QPushButton('Button')
button.setStyleSheet('color: white; background-color: {}'.format(Qt.red.name()))
button.show()
app.exec_()
```
在上面的代码中,我们将 `Qt.white` 直接传递给 `color` 属性,而不是将其转换为字符串。这应该可以解决你遇到的问题。另外,我们还添加了一个 `background-color` 属性来设置按钮的背景颜色。你可以根据需要进行修改。
相关问题
请解释此段代码class GATrainer(): def __init__(self, input_A, input_B): self.program = fluid.default_main_program().clone() with fluid.program_guard(self.program): self.fake_B = build_generator_resnet_9blocks(input_A, name="g_A")#真A-假B self.fake_A = build_generator_resnet_9blocks(input_B, name="g_B")#真B-假A self.cyc_A = build_generator_resnet_9blocks(self.fake_B, "g_B")#假B-复原A self.cyc_B = build_generator_resnet_9blocks(self.fake_A, "g_A")#假A-复原B self.infer_program = self.program.clone() diff_A = fluid.layers.abs( fluid.layers.elementwise_sub( x=input_A, y=self.cyc_A)) diff_B = fluid.layers.abs( fluid.layers.elementwise_sub( x=input_B, y=self.cyc_B)) self.cyc_loss = ( fluid.layers.reduce_mean(diff_A) + fluid.layers.reduce_mean(diff_B)) * cycle_loss_factor #cycle loss self.fake_rec_B = build_gen_discriminator(self.fake_B, "d_B")#区分假B为真还是假 self.disc_loss_B = fluid.layers.reduce_mean( fluid.layers.square(self.fake_rec_B - 1))###优化生成器A2B,所以判别器结果越接近1越好 self.g_loss_A = fluid.layers.elementwise_add(self.cyc_loss, self.disc_loss_B) vars = [] for var in self.program.list_vars(): if fluid.io.is_parameter(var) and var.name.startswith("g_A"): vars.append(var.name) self.param = vars lr = 0.0002 optimizer = fluid.optimizer.Adam( learning_rate=fluid.layers.piecewise_decay( boundaries=[ 100 * step_per_epoch, 120 * step_per_epoch, 140 * step_per_epoch, 160 * step_per_epoch, 180 * step_per_epoch ], values=[ lr, lr * 0.8, lr * 0.6, lr * 0.4, lr * 0.2, lr * 0.1 ]), beta1=0.5, name="g_A") optimizer.minimize(self.g_loss_A, parameter_list=vars)
这段代码定义了一个 GATrainer 类,用于训练图像转换模型 CycleGAN 中的生成器模型 g_A。在初始化函数中,它使用 PaddlePaddle 框架的 fluid.default_main_program() 函数克隆默认的主程序,并使用 with fluid.program_guard() 语句将克隆的程序设置为默认程序。接下来,它定义了四个生成器模型:self.fake_B、self.fake_A、self.cyc_A 和 self.cyc_B。其中,self.fake_B 和 self.fake_A 分别将输入的图像 input_A 和 input_B 转换为假的图像。self.cyc_A 和 self.cyc_B 则将生成的假图像 self.fake_B 和 self.fake_A 分别还原回原始图像 input_A 和 input_B。然后,它定义了一个 inference 程序,用于测试模型。接着,它计算了循环一致性损失和生成器损失。循环一致性损失是指将生成的假图像还原为原始图像的损失。生成器损失是指通过判别器模型将生成的假图像区分为真实图像或假图像的损失。最后,它定义了一个优化器,使用 Adam 优化算法对生成器模型进行优化,以最小化生成器损失。
class PPO(object): def __init__(self): self.sess = tf.Session() self.tfs = tf.placeholder(tf.float32, [None, S_DIM], 'state') # critic with tf.variable_scope('critic'): l1 = tf.layers.dense(self.tfs, 100, tf.nn.relu) self.v = tf.layers.dense(l1, 1) self.tfdc_r = tf.placeholder(tf.float32, [None, 1], 'discounted_r') self.advantage = self.tfdc_r - self.v self.closs = tf.reduce_mean(tf.square(self.advantage)) self.ctrain_op = tf.train.AdamOptimizer(C_LR).minimize(self.closs) # actor pi, pi_params = self._build_anet('pi', trainable=True) oldpi, oldpi_params = self._build_anet('oldpi', trainable=False) with tf.variable_scope('sample_action'): self.sample_op = tf.squeeze(pi.sample(1), axis=0) # choosing action with tf.variable_scope('update_oldpi'): self.update_oldpi_op = [oldp.assign(p) for p, oldp in zip(pi_params, oldpi_params)] self.tfa = tf.placeholder(tf.float32, [None, A_DIM], 'action') self.tfadv = tf.placeholder(tf.float32, [None, 1], 'advantage') with tf.variable_scope('loss'): with tf.variable_scope('surrogate'): # ratio = tf.exp(pi.log_prob(self.tfa) - oldpi.log_prob(self.tfa)) ratio = pi.prob(self.tfa) / (oldpi.prob(self.tfa) + 1e-5) surr = ratio * self.tfadv if METHOD['name'] == 'kl_pen': self.tflam = tf.placeholder(tf.float32, None, 'lambda') kl = tf.distributions.kl_divergence(oldpi, pi) self.kl_mean = tf.reduce_mean(kl) self.aloss = -(tf.reduce_mean(surr - self.tflam * kl)) else: # clipping method, find this is better self.aloss = -tf.reduce_mean(tf.minimum( surr, tf.clip_by_value(ratio, 1.-METHOD['epsilon'], 1.+METHOD['epsilon'])*self.tfadv))
这段代码是使用 PPO(Proximal Policy Optimization)算法实现的一个 actor-critic 模型。其中,critic 用来评价当前状态的价值,actor 用来生成在当前状态下采取的动作。在训练过程中,会使用 advantage(优势值)来评价采取某个动作的好坏,并且使用 surrogate loss(代理损失)来训练 actor,同时使用 clipping 或 kl penalty 的方式来限制优势值的大小,以保证训练的稳定性。
阅读全文
相关推荐
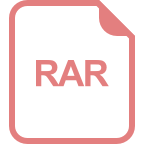
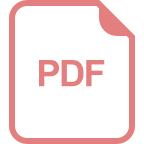
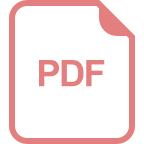













