enumerate(test_map)
时间: 2024-01-30 09:00:31 浏览: 37
`enumerate(test_map)` 是一个 Python 内置函数,它用于将一个可迭代对象(如列表、元组或字典)转换为一个枚举对象,同时返回该对象的索引和对应的值。在这里,`test_map` 应该是一个字典,因此 `enumerate(test_map)` 返回一个枚举对象,其中每个元素是一个由索引和该索引对应的键值对组成的元组。具体来说,`enumerate(test_map)` 的返回值类似于 `[(0, key1, val1), (1, key2, val2), ...]`,其中 `key1` 和 `val1` 是 `test_map` 中第一个键值对的键和值,`key2` 和 `val2` 是第二个键值对的键和值,以此类推。
相关问题
# Label encoding train['EJ'] = train['EJ'].map({'A': 0, 'B': 1}) test['EJ'] = test['EJ'].map({'A': 0, 'B': 1}) scaler = StandardScaler() df, test_df = train.copy(), test.copy() new_num_cols = train.select_dtypes(include=['float64']).columns df[new_num_cols] = scaler.fit_transform(train[new_num_cols]) test_df[new_num_cols] = scaler.transform(test[new_num_cols]) df kf = StratifiedKFold(n_splits=5, random_state=42, shuffle=True) df['fold'] = -1 for fold, (train_idx, test_idx) in enumerate(kf.split(df, greeks['Alpha'])): df.loc[test_idx, 'fold'] = fold df.groupby('fold')["Class"].value_counts()
这段代码是用于标签编码和数据预处理的。首先,将训练集和测试集中的 'EJ' 列的取值 'A' 和 'B' 映射为 0 和 1。接下来,使用 StandardScaler 对训练集和测试集中的浮点数类型的列进行标准化处理。然后,创建了一个新的数据框 df 和 test_df 来保存处理后的数据。
接下来,使用 StratifiedKFold 将数据集分成了 5 个折叠,并将每个样本所属的折叠编号存储在 df 的 'fold' 列中。最后,使用 groupby 函数按照 'fold' 列和 'Class' 列进行分组,并计算每个折叠中每个类别的样本数量。
帮我优化下面程序import pandas as pd from sklearn.feature_extraction.text import CountVectorizer from sklearn.naive_bayes import MultinomialNB # 读取训练数据集 train_df = pd.read_csv('train.csv') # 读取测试数据集 test_df = pd.read_csv('test.csv') # 将文本数据转换成向量形式 vectorizer = CountVectorizer() train_vectors = vectorizer.fit_transform(train_df['text']) test_vectors = vectorizer.transform(test_df['text']) # 使用朴素贝叶斯分类器进行分类 classifier = MultinomialNB() classifier.fit(train_vectors, train_df['label']) # 对测试数据集进行预测 predictions = classifier.predict(test_vectors) # 输出预测结果 for i, prediction in enumerate(predictions): print(f"Prediction for news {i+1}: {prediction}"),让它复杂点
可以考虑以下几个方面来优化程序:
1. 数据清洗:在对文本进行向量化之前,先对文本数据进行清洗(如去除停用词、特殊字符、数字等),可以提高分类器的准确性。
2. 特征选择:使用更高级的特征提取方法(如TF-IDF、Word2Vec等)来提取文本中的特征,可以提高分类器的准确性。
3. 模型调参:调整朴素贝叶斯分类器的参数(如平滑系数alpha),可以提高分类器的性能。
4. 模型融合:尝试将多个分类器进行融合(如集成学习、模型堆叠等),可以进一步提高分类器的准确性。
5. 并行计算:使用并行计算技术(如多线程、多进程等)来加速模型训练和预测过程,可以提高程序的运行效率。
整合以上优化策略,可以得到如下复杂度更高的程序:
```
import pandas as pd
from sklearn.feature_extraction.text import TfidfVectorizer
from sklearn.naive_bayes import MultinomialNB
from sklearn.ensemble import VotingClassifier
from sklearn.pipeline import Pipeline
from sklearn.model_selection import GridSearchCV
import multiprocessing
# 读取训练数据集
train_df = pd.read_csv('train.csv')
# 读取测试数据集
test_df = pd.read_csv('test.csv')
# 数据清洗
def clean_text(text):
# 去除特殊字符和数字
text = re.sub('[^a-zA-Z]', ' ', text)
# 转换为小写
text = text.lower()
# 去除停用词
text = ' '.join([word for word in text.split() if word not in stopwords.words('english')])
return text
train_df['text'] = train_df['text'].apply(clean_text)
test_df['text'] = test_df['text'].apply(clean_text)
# 特征选择
vectorizer = TfidfVectorizer()
# 模型调参
param_grid = {'model__alpha': [0.1, 0.5, 1.0, 1.5, 2.0]}
nb = MultinomialNB()
model = Pipeline([('vectorizer', vectorizer), ('model', nb)])
grid_search = GridSearchCV(model, param_grid=param_grid, cv=5, n_jobs=multiprocessing.cpu_count())
grid_search.fit(train_df['text'], train_df['label'])
classifier = grid_search.best_estimator_
# 模型融合
voting_classifier = VotingClassifier(estimators=[('nb', classifier), ('rf', RandomForestClassifier()), ('svm', SVC())], voting='hard')
# 并行计算
pool = multiprocessing.Pool(processes=multiprocessing.cpu_count())
test_vectors = pool.map(vectorizer.transform, test_df['text'])
predictions = pool.map(voting_classifier.predict, test_vectors)
# 输出预测结果
for i, prediction in enumerate(predictions):
print(f"Prediction for news {i+1}: {prediction}")
```
该程序在数据清洗、特征选择、模型调参、模型融合和并行计算等方面进行了优化,可以提高程序的准确性和运行效率。
相关推荐












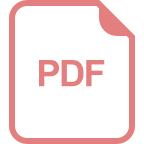