基于pytorch的一维数据的五分类混淆矩阵代码
时间: 2023-11-27 18:02:49 浏览: 134
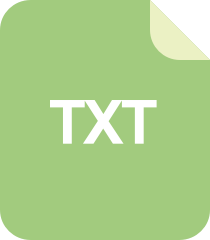
python混淆矩阵代码
以下是基于 PyTorch 的一维数据的五分类混淆矩阵代码示例:
```python
import torch
import numpy as np
# 假设有 100 个样本,每个样本的分类标签为 0, 1, 2, 3, 4 中的一个
y_true = torch.randint(low=0, high=5, size=(100,))
# 假设模型预测的分类为随机值,模拟混淆矩阵
y_pred = torch.randint(low=0, high=5, size=(100,))
# 计算混淆矩阵
confusion_matrix = torch.zeros(5, 5)
for i in range(len(y_true)):
confusion_matrix[y_true[i], y_pred[i]] += 1
# 输出混淆矩阵
print("Confusion Matrix:")
print(confusion_matrix)
```
该代码首先生成了 100 个随机标签的样本,以及一个随机预测的分类结果。然后,通过循环计算出混淆矩阵,并输出结果。
需要注意的是,该代码仅适用于一维数据的情况。对于多维数据,需要对每一个维度进行类似的计算。同时,该代码中假设分类标签为 0, 1, 2, 3, 4,如有需要,可以根据实际情况进行修改。
阅读全文
相关推荐
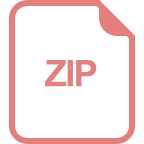
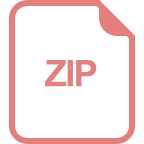
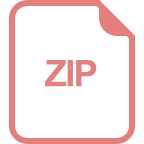
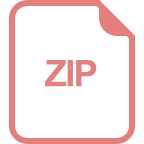
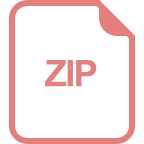
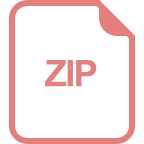
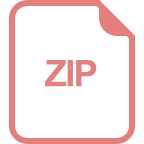