def init_weights(self, num_layers, pretrained=True): if pretrained: # print('=> init resnet deconv weights from normal distribution') for _, m in self.deconv_layers.named_modules(): if isinstance(m, nn.ConvTranspose2d): # print('=> init {}.weight as normal(0, 0.001)'.format(name)) # print('=> init {}.bias as 0'.format(name)) nn.init.normal_(m.weight, std=0.001) if self.deconv_with_bias: nn.init.constant_(m.bias, 0) elif isinstance(m, nn.BatchNorm2d): # print('=> init {}.weight as 1'.format(name)) # print('=> init {}.bias as 0'.format(name)) nn.init.constant_(m.weight, 1) nn.init.constant_(m.bias, 0) # print('=> init final conv weights from normal distribution') for head in self.heads: final_layer = self.__getattr__(head) for i, m in enumerate(final_layer.modules()): if isinstance(m, nn.Conv2d): # nn.init.kaiming_normal_(m.weight, mode='fan_out', nonlinearity='relu') # print('=> init {}.weight as normal(0, 0.001)'.format(name)) # print('=> init {}.bias as 0'.format(name)) if m.weight.shape[0] == self.heads[head]: if 'hm' in head: nn.init.constant_(m.bias, -2.19) else: nn.init.normal_(m.weight, std=0.001) nn.init.constant_(m.bias, 0) #pretrained_state_dict = torch.load(pretrained) url = model_urls['resnet{}'.format(num_layers)] pretrained_state_dict = model_zoo.load_url(url) print('=> loading pretrained model {}'.format(url)) self.load_state_dict(pretrained_state_dict, strict=False) else: print('=> imagenet pretrained model dose not exist') print('=> please download it first') raise ValueError('imagenet pretrained model does not exist')
时间: 2024-02-14 14:32:11 浏览: 171
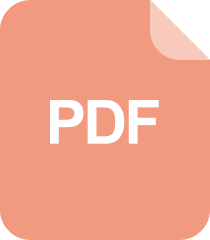
keras读取h5文件load_weights、load代码操作
这段代码是一个神经网络模型初始化权重的函数。根据输入的参数,函数会根据预训练的权重初始化模型的卷积层和批归一化层的权重。如果pretrained参数为True,函数还会加载预训练模型的权重。
具体地,函数会遍历模型的deconv_layers,并对其中的卷积层和批归一化层进行初始化。对于卷积层,权重会从均值为0、标准差为0.001的正态分布中采样得到,偏置会被初始化为0。对于批归一化层,权重会被初始化为1,偏置会被初始化为0。
接着,函数会遍历模型的heads,并对每个head中的最后一层卷积层进行初始化。初始化方式与上述相同,不过对于特定的head(如包含'hm'的head),偏置会被初始化为-2.19。
最后,如果pretrained为True,函数会加载预训练模型的权重。预训练模型的URL由参数num_layers指定。
如果pretrained为False,则会打印错误信息并抛出ValueError异常。
阅读全文
相关推荐
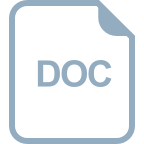
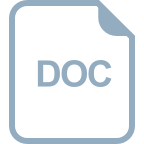















