神经网络 bias variance
时间: 2023-09-24 20:07:00 浏览: 143
神经网络的偏差(bias)和方差(variance)是衡量模型的两个重要指标。
偏差是指模型对于训练数据的拟合能力,即模型是否能够准确地捕捉到数据中的真实模式。如果模型具有较高的偏差,意味着模型无法很好地拟合训练数据,可能存在欠拟合的问题。换句话说,模型的偏差较大时,其预测结果与真实值之间存在较大的误差。
方差是指模型对于不同训练集的稳定性,即当给定不同的训练集时,模型是否能够产生一致的预测结果。如果模型具有较高的方差,意味着模型对于训练数据过于敏感,可能存在过拟合的问题。换句话说,模型的方差较大时,其预测结果在不同样本上会有较大的变化。
偏差和方差通常是一个折衷问题。高偏差的模型可能会忽略数据中的重要特征,导致欠拟合;而高方差的模型可能过于复杂,过度拟合了训练数据,导致在新数据上表现不佳。
为了找到一个合适的模型,我们需要在偏差和方差之间进行权衡。通过调整模型的复杂度、增加训练数据量、使用正则化方法等方式,可以尝试降低偏差或方差,从而提高模型的整体性能。
相关问题
基于卷积神经网络的图像识别外文翻译
Title: Image Recognition Based on Convolutional Neural Networks
Abstract: Image recognition has been a popular research topic in the field of computer vision. With the development of deep learning, convolutional neural networks (CNNs) have shown excellent performance in this area. In this paper, we introduce the basic structure and principles of CNNs, and then discuss the application of CNNs in image recognition. Specifically, we focus on the training process of CNNs, including data preprocessing, network initialization, and optimization algorithms. We also compare different CNN architectures and evaluate their performance on benchmark datasets. Finally, we summarize the advantages and limitations of CNNs in image recognition, and suggest some potential directions for future research.
Keywords: Convolutional neural networks, image recognition, deep learning, data preprocessing, network initialization, optimization algorithms
1. Introduction
Image recognition, also known as image classification, is a fundamental task in computer vision. The goal is to assign a label to an input image from a predefined set of categories. Image recognition has a wide range of applications, such as object detection, face recognition, and scene understanding. Traditional image recognition methods usually rely on handcrafted features and machine learning algorithms, which require domain expertise and extensive manual effort. In recent years, deep learning has emerged as a powerful tool for image recognition, and convolutional neural networks (CNNs) have become the state-of-the-art approach in this area.
CNNs are a class of neural networks that are specifically designed for image analysis. They employ convolutional layers to extract local features from the input image, and use pooling layers to reduce the spatial dimensionality. The output of the convolutional layers is then fed into fully connected layers, which perform high-level reasoning and produce the final classification result. CNNs have several advantages over traditional methods. First, they can automatically learn hierarchical representations of the input data, without the need for manual feature engineering. Second, they are able to capture spatial correlations and translation invariance, which are important characteristics of natural images. Third, they can handle large-scale datasets and are computationally efficient.
In this paper, we provide a comprehensive overview of CNNs for image recognition. We begin by introducing the basic structure and principles of CNNs, including convolutional layers, pooling layers, and fully connected layers. We then discuss the training process of CNNs, which includes data preprocessing, network initialization, and optimization algorithms. We also compare different CNN architectures, such as LeNet, AlexNet, VGG, GoogLeNet, and ResNet, and evaluate their performance on benchmark datasets, such as MNIST, CIFAR-10, and ImageNet. Finally, we summarize the advantages and limitations of CNNs in image recognition, and suggest some potential directions for future research.
2. Convolutional Neural Networks
2.1 Basic Structure and Principles
CNNs are composed of multiple layers, including convolutional layers, pooling layers, and fully connected layers. The input to a CNN is an image, represented as a matrix of pixel values. The output is a predicted label, which is one of the predefined categories.
Convolutional layers are the core components of a CNN. They consist of a set of learnable filters, each of which is a small matrix of weights. The filters are convolved with the input image, producing a feature map that highlights the presence of certain patterns or structures. The convolution operation is defined as follows:
\begin{equation}
y_{i,j}=\sum_{m=1}^{M}\sum_{n=1}^{N}w_{m,n}x_{i+m-1,j+n-1}+b
\end{equation}
where y_{i,j} is the output at position (i,j) of the feature map, x_{i+m-1,j+n-1} is the input at position (i+m-1,j+n-1), w_{m,n} is the weight at position (m,n) of the filter, b is a bias term, and M and N are the dimensions of the filter.
Pooling layers are used to reduce the spatial dimensionality of the feature map. They operate on small regions of the map, such as 2x2 or 3x3 patches, and perform a simple operation, such as taking the maximum or average value. Pooling helps to improve the robustness of the network to small translations and distortions in the input image.
Fully connected layers are used to perform high-level reasoning and produce the final classification result. They take the output of the convolutional and pooling layers, flatten it into a vector, and pass it through a set of nonlinear activation functions. The output of the last fully connected layer is a probability distribution over the predefined categories, which is obtained by applying the softmax function:
\begin{equation}
p_{i}=\frac{e^{z_{i}}}{\sum_{j=1}^{K}e^{z_{j}}}
\end{equation}
where p_{i} is the predicted probability of category i, z_{i} is the unnormalized score of category i, and K is the total number of categories.
2.2 Training Process
The training process of a CNN involves several steps, including data preprocessing, network initialization, and optimization algorithms.
Data preprocessing is a crucial step in CNN training, as it can significantly affect the performance of the network. Common preprocessing techniques include normalization, data augmentation, and whitening. Normalization scales the pixel values to have zero mean and unit variance, which helps to stabilize the training process and improve convergence. Data augmentation generates new training examples by applying random transformations to the original images, such as rotations, translations, and flips. This helps to increase the size and diversity of the training set, and reduces overfitting. Whitening removes the linear dependencies between the pixel values, which decorrelates the input features and improves the discriminative power of the network.
Network initialization is another important aspect of CNN training, as it can affect the convergence and generalization of the network. There are several methods for initializing the weights, such as random initialization, Gaussian initialization, and Xavier initialization. Random initialization initializes the weights with small random values, which can lead to slow convergence and poor performance. Gaussian initialization initializes the weights with random values drawn from a Gaussian distribution, which can improve convergence and performance. Xavier initialization initializes the weights with values that are scaled according to the number of input and output neurons, which helps to balance the variance of the activations and gradients.
Optimization algorithms are used to update the weights of the network during training, in order to minimize the objective function. Common optimization algorithms include stochastic gradient descent (SGD), Adam, and Adagrad. SGD updates the weights using the gradient of the objective function with respect to the weights, multiplied by a learning rate. Adam adapts the learning rate dynamically based on the first and second moments of the gradient. Adagrad adapts the learning rate for each weight based on its past gradients, which helps to converge faster for sparse data.
3. CNN Architectures
There have been many CNN architectures proposed in the literature, each with its own strengths and weaknesses. In this section, we briefly introduce some of the most popular architectures, and evaluate their performance on benchmark datasets.
LeNet is one of the earliest CNN architectures, proposed by Yann LeCun in 1998 for handwritten digit recognition. It consists of two convolutional layers, followed by two fully connected layers, and uses the sigmoid activation function. LeNet achieved state-of-the-art performance on the MNIST dataset, with an error rate of 0.8%.
AlexNet is a landmark CNN architecture, proposed by Alex Krizhevsky et al. in 2012 for the ImageNet challenge. It consists of five convolutional layers, followed by three fully connected layers, and uses the rectified linear unit (ReLU) activation function. AlexNet achieved a top-5 error rate of 15.3% on the ImageNet dataset, which was a significant improvement over the previous state-of-the-art method.
VGG is another CNN architecture, proposed by Karen Simonyan and Andrew Zisserman in 2014. It consists of up to 19 convolutional layers, followed by two fully connected layers, and uses the ReLU activation function. VGG achieved a top-5 error rate of 7.3% on the ImageNet dataset, which was the best performance at the time.
GoogLeNet is a CNN architecture, proposed by Christian Szegedy et al. in 2014. It consists of 22 layers, including multiple inception modules, which are composed of parallel convolutional and pooling layers at different scales. GoogLeNet achieved a top-5 error rate of 6.7% on the ImageNet dataset, with much fewer parameters than VGG.
ResNet is a CNN architecture, proposed by Kaiming He et al. in 2015. It consists of residual blocks, which allow the network to learn residual connections between layers, and avoid the vanishing gradient problem. ResNet achieved a top-5 error rate of 3.57% on the ImageNet dataset, which was the best performance at the time.
4. Conclusion and Future Work
In this paper, we provided a comprehensive overview of CNNs for image recognition, including the basic structure and principles, the training process, and the comparison of different architectures on benchmark datasets. CNNs have shown remarkable performance in image recognition, and have become the state-of-the-art approach in this area. However, there are still some challenges that need to be addressed, such as improving the robustness and interpretability of the network, handling noisy and incomplete data, and scaling up the training process to larger datasets and more complex tasks. In the future, we expect to see more research on these topics, and more applications of CNNs in various domains.
基于tensorflow的卷积神经网络空间注意力机制代码
以下是基于TensorFlow的卷积神经网络空间注意力机制的代码示例:
```python
import tensorflow as tf
def spatial_attention(input_feature, name='spatial_attention'):
with tf.variable_scope(name):
kernel_size = 7
kernel_initializer = tf.contrib.layers.variance_scaling_initializer()
bias_initializer = tf.constant_initializer(value=0.0)
filters = input_feature.get_shape()[-1]
# Compute attention map
attention = tf.layers.conv2d(inputs=input_feature,
filters=filters,
kernel_size=kernel_size,
padding='same',
activation=tf.nn.sigmoid,
kernel_initializer=kernel_initializer,
bias_initializer=bias_initializer,
name='attention')
# Apply attention map
attention_feature = tf.multiply(input_feature, attention, name='attention_feature')
return attention_feature
```
在这个函数中,我们首先定义了一些超参数,如卷积核大小、初始化方式等。然后,我们使用一个卷积层来计算输入特征的注意力图。注意力图是一个与输入特征相同大小的张量,其中每个元素都是一个0到1之间的数,用于控制输入特征的重要程度。最后,我们将输入特征与注意力图相乘,得到加权后的特征图作为输出。
在使用这个函数时,我们只需要将待处理的特征图作为输入传入即可:
```python
input_feature = tf.placeholder(tf.float32, shape=[None, 64, 64, 32])
attention_feature = spatial_attention(input_feature)
```
这里我们使用了一个占位符来表示输入特征,然后使用`spatial_attention`函数对其进行处理,得到加权后的特征图。
阅读全文
相关推荐
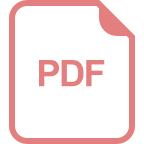
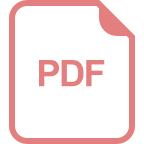
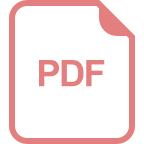
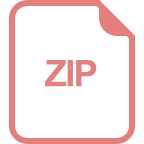
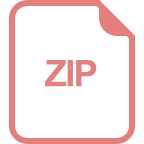
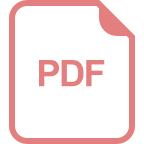
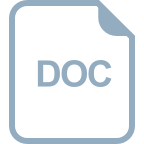
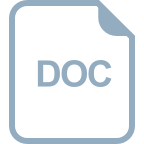
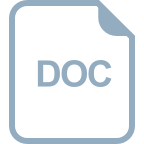
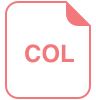
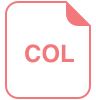
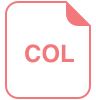
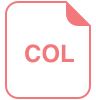
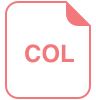
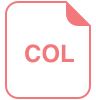
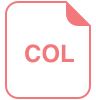
