python代码怎么写多分类的化auc曲线
时间: 2023-09-12 08:08:46 浏览: 83
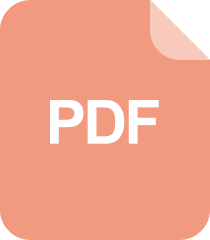
AUC计算方法与Python实现代码
要绘制多分类任务的AUC曲线,您可以使用`sklearn.metrics.roc_curve`函数来计算每个类别的假正率(False Positive Rate)和真正率(True Positive Rate),然后使用Matplotlib库来绘制曲线。
以下是一个示例代码,演示如何计算并绘制多分类任务的AUC曲线:
```python
from sklearn.metrics import roc_curve, auc
import matplotlib.pyplot as plt
import numpy as np
# 假设有3个类别,每个类别有10个样本
# 假设真实标签为labels,预测概率为probs
# labels是每个样本的真实标签,以0、1、2表示三个类别
labels = np.array([0, 1, 2, 0, 1, 2, 0, 1, 2, 0, 1, 2, 0, 1, 2, 0, 1, 2, 0, 1])
# probs是每个样本属于各个类别的预测概率,以二维数组表示
probs = np.array([[0.9, 0.05, 0.05], [0.2, 0.7, 0.1], [0.1, 0.4, 0.5],
[0.8, 0.15, 0.05], [0.3, 0.5, 0.2], [0.1, 0.3, 0.6],
[0.7, 0.2, 0.1], [0.3, 0.4, 0.3], [0.05, 0.2, 0.75],
[0.6, 0.25, 0.15], [0.3, 0.4, 0.3], [0.2, 0.6, 0.2],
[0.7, 0.15, 0.15], [0.2, 0.3, 0.5], [0.1, 0.4, 0.5],
[0.8, 0.1, 0.1], [0.3, 0.55, 0.15], [0.05, 0.25, 0.7],
[0.9, 0.05, 0.05], [0.2, 0.6, 0.2]])
# 计算多分类AUC曲线
fpr = dict()
tpr = dict()
roc_auc = dict()
n_classes = len(np.unique(labels))
for i in range(n_classes):
fpr[i], tpr[i], _ = roc_curve(labels == i, probs[:, i])
roc_auc[i] = auc(fpr[i], tpr[i])
# 绘制AUC曲线
plt.figure()
colors = ['blue', 'red', 'green']
for i in range(n_classes):
plt.plot(fpr[i], tpr[i], color=colors[i], label='Class %d (AUC = %0.2f)' % (i, roc_auc[i]))
plt.plot([0, 1], [0, 1], color='black', linestyle='--')
plt.xlim([0.0, 1.0])
plt.ylim([0.0, 1.05])
plt.xlabel('False Positive Rate')
plt.ylabel('True Positive Rate')
plt.title('Multi-class ROC Curve')
plt.legend(loc="lower right")
plt.show()
```
在上面的示例中,我们首先使用`roc_curve`函数计算每个类别的假正率(FPR)和真正率(TPR)。然后,使用`auc`函数计算每个类别的AUC。最后,使用Matplotlib库绘制AUC曲线。每个类别的AUC值以标签形式显示在曲线旁边。
请注意,上述示例假设了三个类别,您可以根据您的实际情况进行调整。此外,如果您的模型输出的是类别标签而不是概率,您需要将其转换为概率形式,例如使用softmax函数。
阅读全文
相关推荐











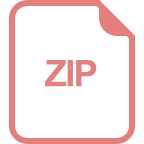
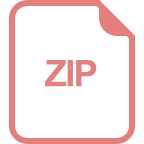