cv2.findcontours和cv2.boundingRect
时间: 2023-10-27 18:55:44 浏览: 52
cv2.findContours是一个用于查找图像中的轮廓的函数,它接受一个二值图像作为输入,并返回一个轮廓的列表。每个轮廓是一个由点组成的数组,表示图像中的连续边界。
cv2.boundingRect是一个用于计算轮廓的边界框的函数。它接受一个轮廓作为输入,并返回一个矩形(x, y, w, h),其中(x, y)是矩形左上角的坐标,w是矩形的宽度,h是矩形的高度。这个边界框是能够完全包围轮廓的最小矩形。
通常,我们可以使用cv2.findContours来找到图像中的轮廓,并使用cv2.boundingRect来计算每个轮廓的边界框,以便进行进一步的处理,如目标检测或图像分割。
相关问题
使用cv2.findContours对图像进行配准和拼接
cv2.findContours函数用于查找图像中的轮廓。配准和拼接图像通常需要先找到它们的共同特征,例如边缘。以下是一个简单的例子来说明如何使用cv2.findContours进行图像配准和拼接:
```python
import cv2
import numpy as np
# 读取两张图像
img1 = cv2.imread('image1.png', 0)
img2 = cv2.imread('image2.png', 0)
# 边缘检测
edge1 = cv2.Canny(img1, 100, 200)
edge2 = cv2.Canny(img2, 100, 200)
# 查找轮廓
contours1, _ = cv2.findContours(edge1, cv2.RETR_EXTERNAL, cv2.CHAIN_APPROX_SIMPLE)
contours2, _ = cv2.findContours(edge2, cv2.RETR_EXTERNAL, cv2.CHAIN_APPROX_SIMPLE)
# 找到最大轮廓
cnt1 = max(contours1, key=cv2.contourArea)
cnt2 = max(contours2, key=cv2.contourArea)
# 计算轮廓的外接矩形
x1, y1, w1, h1 = cv2.boundingRect(cnt1)
x2, y2, w2, h2 = cv2.boundingRect(cnt2)
# 将图像1和图像2拼接在一起
result = np.zeros((max(y1+h1, y2+h2), x1+w1+x2, 3), np.uint8)
result[y1:y1+h1, 0:w1] = cv2.cvtColor(img1, cv2.COLOR_GRAY2BGR)
result[y2:y2+h2, x1:x1+w2] = cv2.cvtColor(img2, cv2.COLOR_GRAY2BGR)
# 显示结果
cv2.imshow('result', result)
cv2.waitKey(0)
cv2.destroyAllWindows()
```
在这个例子中,我们首先读取两张图像,然后对它们进行边缘检测。接下来,我们使用cv2.findContours函数查找图像中的轮廓,并找到最大轮廓。然后,我们计算轮廓的外接矩形,并将两张图像拼接在一起。最后,我们显示拼接后的图像。
请注意,这只是一个简单的例子,实际的图像配准和拼接可能需要更复杂的算法和技术。
介绍cv2.findContours
`cv2.findContours` 是 OpenCV 中用于查找图像轮廓的函数,它可以在二值化图像中查找所有的连通轮廓,返回轮廓的点集和层次结构信息。 `cv2.findContours` 的输入参数包括:
- `image`:需要查找轮廓的二值化图像,通常为灰度图像或者二值图像。
- `mode`:轮廓查找模式,可以为 `cv2.RETR_EXTERNAL`(只检测外轮廓)、`cv2.RETR_LIST`(检测所有轮廓,不建立层次关系)等。
- `method`:轮廓逼近方法,可以为 `cv2.CHAIN_APPROX_NONE`(保存所有轮廓点)、`cv2.CHAIN_APPROX_SIMPLE`(压缩水平、垂直、对角线方向的点,仅保留端点)等。
`cv2.findContours` 的输出结果是一个三元组 `(contours, hierarchy, ret)`,其中:
- `contours`:表示检测到的轮廓的点集,是一个列表,每个元素都是一个 Numpy 数组,表示一个轮廓。
- `hierarchy`:表示轮廓的层次结构,是一个 Numpy 数组,每行的四个元素表示当前轮廓的后一个轮廓、前一个轮廓、第一个子轮廓和父轮廓。
- `ret`:表示是否成功检测到轮廓的标志,如果成功则为 True,否则为 False。
可以使用 `cv2.drawContours` 函数在图像上绘制检测到的轮廓,也可以使用 `cv2.boundingRect` 函数计算轮廓的边界矩形或者最小包围矩形等形状信息。
相关推荐
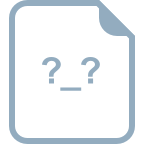
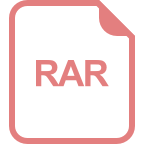
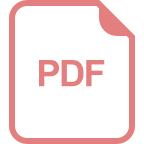












