nesterov accelerated gradient 好处
时间: 2023-11-16 17:02:33 浏览: 100
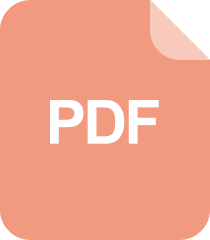
Nesterov Accelerated Gradient Descent-Based Convolution Neural Network with Dropout for Facial Expression Recognition
Nesterov加速梯度(NAG)是一种优化算法,与传统的梯度下降算法相比有许多优点。首先,NAG可以更快地收敛到最优解,这是因为它可以在更新参数时考虑梯度的方向,而不是只考虑当前位置的梯度。这样可以减少震荡,并且能够更快地收敛到最优解附近,从而加速了模型的训练速度。
其次,NAG可以更好地处理高曲率的情况,这是因为它可以更准确地预测参数的下一个位置,并能够更快地调整步长。这使得NAG在处理复杂的优化问题时能够更加稳定和可靠。
此外,NAG还可以避免梯度下降算法中的震荡现象,因为它可以根据之前的梯度方向来调整参数的更新方向,从而减少了参数更新的不稳定性。这使得NAG在实际应用中更加容易调节和优化。
总的来说,Nesterov加速梯度算法相比传统的梯度下降算法有着更快的收敛速度、更好的稳定性和更好的处理高曲率情况的能力,因此在实际应用中具有更好的效果和性能。
阅读全文
相关推荐
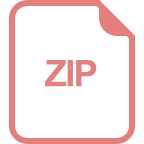
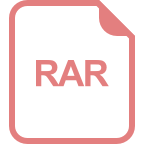

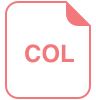

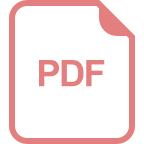
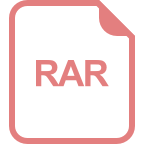
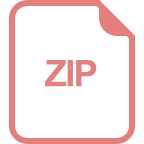
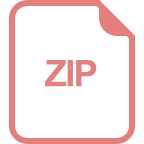
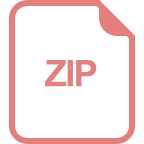
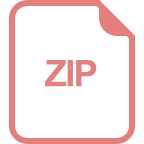
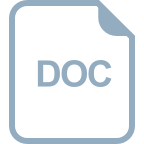
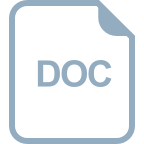
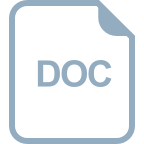



