best_score = float('-inf')
时间: 2024-11-25 12:21:12 浏览: 3
在Python的GridSearchCV中,`best_score`变量默认初始化为浮点数负无穷大(`float('-inf')`),这是为了在遍历参数网格之前有一个基准来判断哪些模型的表现更好。当GridSearchCV完成模型训练并找到最佳参数组合时,`best_score`会被更新为实际的最佳交叉验证得分。
例如,在`Pipeline`和`GridSearchCV`设置中[^2]:
```python
# 初始化best_score为负无穷
best_score = float('-inf')
# 使用GridSearchCV进行模型搜索
gs = GridSearchCV(estimator=pipe_svr,
param_grid = param_grid,
scoring = 'r2', # 使用r2分数评估模型性能
cv = 10) # 10折交叉验证
# 训练模型并寻找最佳参数
gs = gs.fit(X, y)
# 最终的best_score将存储在gs对象中
print("网格搜索最优得分:", gs.best_score_)
print("网格搜索最优参数组合:\n", gs.best_params_)
# 如果gs.best_score_不再是负无穷,说明找到了一个更好的模型
if gs.best_score_ != best_score:
print("找到了一个比初始值更好的模型")
```
相关问题
import numpy as np class Node: j = None theta = None p = None left = None right = None class DecisionTreeBase: def __init__(self, max_depth, feature_sample_rate, get_score): self.max_depth = max_depth self.feature_sample_rate = feature_sample_rate self.get_score = get_score def split_data(self, j, theta, X, idx): idx1, idx2 = list(), list() for i in idx: value = X[i][j] if value <= theta: idx1.append(i) else: idx2.append(i) return idx1, idx2 def get_random_features(self, n): shuffled = np.random.permutation(n) size = int(self.feature_sample_rate * n) selected = shuffled[:size] return selected def find_best_split(self, X, y, idx): m, n = X.shape best_score = float("inf") best_j = -1 best_theta = float("inf") best_idx1, best_idx2 = list(), list() selected_j = self.get_random_features(n) for j in selected_j: thetas = set([x[j] for x in X]) for theta in thetas: idx1, idx2 = self.split_data(j, theta, X, idx) if min(len(idx1), len(idx2)) == 0 : continue score1, score2 = self.get_score(y, idx1), self.get_score(y, idx2) w = 1.0 * len(idx1) / len(idx) score = w * score1 + (1-w) * score2 if score < best_score: best_score = score best_j = j best_theta = theta best_idx1 = idx1 best_idx2 = idx2 return best_j, best_theta, best_idx1, best_idx2, best_score def generate_tree(self, X, y, idx, d): r = Node() r.p = np.average(y[idx], axis=0) if d == 0 or len(idx)<2: return r current_score = self.get_score(y, idx) j, theta, idx1, idx2, score = self.find_best_split(X, y, idx) if score >= current_score: return r r.j = j r.theta = theta r.left = self.generate_tree(X, y, idx1, d-1) r.right = self.generate_tree(X, y, idx2, d-1) return r def fit(self, X, y): self.root = self.generate_tree(X, y, range(len(X)), self.max_depth) def get_prediction(self, r, x): if r.left == None and r.right == None: return r.p value = x[r.j] if value <= r.theta: return self.get_prediction(r.left, x) else: return self.get_prediction(r.right, x) def predict(self, X): y = list() for i in range(len(X)): y.append(self.get_prediction(self.root, X[i])) return np.array(y)
这段代码实现了一个基于决策树的分类器,其中包括以下几个类和方法:
1. Node类:表示决策树节点的类,包括属性j表示节点所选择的特征,属性theta表示节点所选择的特征的阈值,属性p表示节点的预测值,属性left和right分别表示左子树和右子树。
2. DecisionTreeBase类:表示决策树分类器的基类,包括方法__init__()、split_data()、get_random_features()、find_best_split()、generate_tree()、fit()、get_prediction()和predict()。
3. __init__(self, max_depth, feature_sample_rate, get_score)方法:初始化决策树分类器的参数,包括最大深度、特征采样率和评价指标。
4. split_data(self, j, theta, X, idx)方法:根据特征j和阈值theta将数据集X中的数据划分为两部分,返回划分后的两部分数据在数据集X中的索引。
5. get_random_features(self, n)方法:从数据集X中随机选择一定比例的特征,返回特征的索引。
6. find_best_split(self, X, y, idx)方法:在数据集X和标签y中,根据评价指标找到最优的特征和阈值,返回最优特征的索引、最优阈值、划分后的两部分数据在数据集X中的索引以及最优评价指标的值。
7. generate_tree(self, X, y, idx, d)方法:根据数据集X、标签y和索引idx生成一棵决策树,返回根节点。
8. fit(self, X, y)方法:训练决策树分类器,生成决策树。
9. get_prediction(self, r, x)方法:对于输入的数据x,根据决策树节点r的特征和阈值进行判断,选择左子树或右子树,并递归调用get_prediction()方法,直到到达叶子节点返回预测值。
10. predict(self, X)方法:对于输入的数据集X,返回预测值。
import numpy as np from scipy.stats import f 构造数据集 X = np.array([[1, 7, 26, 6, 60], [1, 1, 29, 15, 52], [1, 11, 56, 8, 20], [1, 11, 31, 8, 47], [1, 7, 52, 6, 33], [1, 11, 55, 9, 22], [1, 3, 71, 17, 6], [1, 1, 31, 22, 44], [1, 2, 54, 18, 22], [1, 21, 47, 4, 26], [1, 1, 40, 23, 34], [1, 11, 66, 9, 12], [1, 10, 68, 8, 12]]) Y = np.array([78.5, 74.3, 104.3, 87.6, 95.9, 109.2, 102.7, 72.5, 93.1, 115.9, 83.8, 113.3, 109.4]) 求解回归系数 beta = np.linalg.inv(X.T @ X) @ X.T @ Y 输出回归结果 print('回归系数:', beta) 求解残差平方和和总平方和 Y_pred = X @ beta SSE = np.sum((Y - Y_pred) ** 2) SST = np.sum((Y - np.mean(Y)) ** 2) 计算R平方和调整R平方 R2 = 1 - SSE / SST adj_R2 = 1 - SSE / (len(Y) - len(beta) - 1) / SST print('R平方:', R2) print('调整R平方:', adj_R2) 进行方差分析 MSR = np.sum((Y_pred - np.mean(Y)) ** 2) / (len(beta) - 1) MSE = SSE / (len(Y) - len(beta)) F = MSR / MSE p = 1 - f.cdf(F, len(beta) - 1, len(Y) - len(beta)) print('F值:', F) print('p值:', p) 你能接着上面的代码用全子集法求最优回归方程,请写出完整的py程序
import numpy as np
from itertools import combinations
from scipy.stats import f
# 构造数据集
X = np.array([[1, 7, 26, 6, 60],
[1, 1, 29, 15, 52],
[1, 11, 56, 8, 20],
[1, 11, 31, 8, 47],
[1, 7, 52, 6, 33],
[1, 11, 55, 9, 22],
[1, 3, 71, 17, 6],
[1, 1, 31, 22, 44],
[1, 2, 54, 18, 22],
[1, 21, 47, 4, 26],
[1, 1, 40, 23, 34],
[1, 11, 66, 9, 12],
[1, 10, 68, 8, 12]])
Y = np.array([78.5, 74.3, 104.3, 87.6, 95.9, 109.2, 102.7, 72.5, 93.1, 115.9, 83.8, 113.3, 109.4])
# 全子集法求最优回归方程
n_features = X.shape[1]
best_score = float('-inf')
best_feature_idx = None
for k in range(1, n_features+1):
for subset in combinations(range(n_features), k):
X_subset = X[:, subset]
beta_subset = np.linalg.inv(X_subset.T @ X_subset) @ X_subset.T @ Y
Y_pred_subset = X_subset @ beta_subset
SSE_subset = np.sum((Y - Y_pred_subset) ** 2)
SST_subset = np.sum((Y - np.mean(Y)) ** 2)
R2_subset = 1 - SSE_subset / SST_subset
if R2_subset > best_score:
best_score = R2_subset
best_feature_idx = subset
# 输出最优回归方程
print('最优回归方程的特征索引:', best_feature_idx)
X_best = X[:, best_feature_idx]
beta_best = np.linalg.inv(X_best.T @ X_best) @ X_best.T @ Y
print('最优回归方程的系数:', beta_best)
# 求解残差平方和和总平方和
Y_pred = X_best @ beta_best
SSE = np.sum((Y - Y_pred) ** 2)
SST = np.sum((Y - np.mean(Y)) ** 2)
# 计算R平方和调整R平方
R2 = 1 - SSE / SST
adj_R2 = 1 - SSE / (len(Y) - len(beta_best) - 1) / SST
print('R平方:', R2)
print('调整R平方:', adj_R2)
# 进行方差分析
MSR = np.sum((Y_pred - np.mean(Y)) ** 2) / (len(beta_best) - 1)
MSE = SSE / (len(Y) - len(beta_best))
F = MSR / MSE
p = 1 - f.cdf(F, len(beta_best) - 1, len(Y) - len(beta_best))
print('F值:', F)
print('p值:', p)
阅读全文
相关推荐
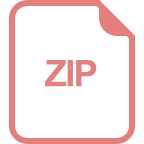
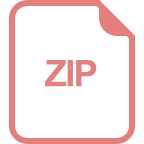
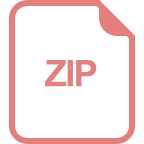













