python 实现xgb回归预测
时间: 2023-09-22 20:02:37 浏览: 45
Python实现XGBoost回归预测可以通过以下步骤完成:
1. 导入必要的库:
```
import xgboost as xgb
from sklearn.metrics import mean_squared_error
from sklearn.model_selection import train_test_split
```
2. 准备数据:
```
# 假设我们已经有了一个包含特征和目标变量的数据集
X, y = load_data()
```
3. 划分训练集和测试集:
```
X_train, X_test, y_train, y_test = train_test_split(X, y, test_size=0.2, random_state=42)
```
4. 定义和训练模型:
```
# 定义XGBoost回归模型
model = xgb.XGBRegressor()
# 训练模型
model.fit(X_train, y_train)
```
5. 预测和评估:
```
# 预测测试集
y_pred = model.predict(X_test)
# 计算均方根误差(RMSE)
rmse = np.sqrt(mean_squared_error(y_test, y_pred))
```
6. 调参优化:
```
# 可以通过调整模型参数来进一步优化模型性能
model = xgb.XGBRegressor(n_estimators=100, max_depth=3)
# 使用交叉验证选择最佳参数
params = {'max_depth': [3, 4, 5], 'learning_rate': [0.1, 0.01, 0.001]}
grid_search = GridSearchCV(model, params, scoring='neg_mean_squared_error', cv=5)
grid_search.fit(X, y)
best_params = grid_search.best_params_
```
通过以上步骤,我们可以实现使用Python和XGBoost库进行回归预测,并根据需要进行调参优化。希望以上信息对你有所帮助。
相关推荐
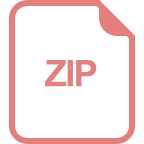
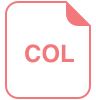













