ctc-attention
时间: 2023-10-29 07:08:11 浏览: 147
CTC-Attention是一种结合了CTC和Attention机制的语音识别模型。CTC用于解决序列标注问题中输入标签与输出标签的对齐问题,而Attention机制则用于从众多信息中选出对当前任务目标来说重要的信息,忽略其他不重要的信息。CTC-Attention模型的优点在于可以同时利用CTC和Attention的优势,提高语音识别的准确率。同时,CTC-Attention模型也可以应用于在线识别任务中,通过单调逐块注意力和单调截断注意力等方法来解决在线识别中的问题。
相关问题
ctc和attention代码
CTC(Connectionist Temporal Classification)和Attention是两种不同的序列建模方法,分别用于语音识别和自然语言处理等任务。下面是一个简单的实现示例:
1. CTC
CTC是一种无需对齐标签的序列建模方法,常用于语音识别和手写字符识别等任务。以下是一个使用TensorFlow实现CTC的示例:
```python
import tensorflow as tf
from tensorflow.keras import layers
# 定义模型
def ctc_model(input_dim, output_dim, units=128):
input = layers.Input(shape=(None, input_dim))
lstm = layers.LSTM(units, return_sequences=True)(input)
lstm = layers.LSTM(units, return_sequences=True)(lstm)
output = layers.Dense(output_dim, activation='softmax')(lstm)
model = tf.keras.Model(inputs=input, outputs=output)
return model
# 编译模型
model = ctc_model(input_dim=20, output_dim=10)
model.compile(loss=tf.keras.backend.ctc_batch_cost, optimizer='adam')
# 训练模型
model.fit(x_train, y_train, validation_data=(x_val, y_val), epochs=10)
```
其中,`ctc_batch_cost`是TensorFlow中的CTC损失函数。
2. Attention
Attention是一种机制,用于增强序列模型的表现力。以下是一个使用PyTorch实现Attention的示例:
```python
import torch
import torch.nn as nn
# 定义模型
class Attention(nn.Module):
def __init__(self, input_dim, hidden_dim):
super(Attention, self).__init__()
self.input_dim = input_dim
self.hidden_dim = hidden_dim
self.W = nn.Linear(input_dim, hidden_dim, bias=False)
self.U = nn.Linear(hidden_dim, hidden_dim, bias=False)
self.v = nn.Linear(hidden_dim, 1, bias=False)
def forward(self, inputs):
# inputs shape: (batch_size, seq_len, input_dim)
e = torch.tanh(self.W(inputs)) # e shape: (batch_size, seq_len, hidden_dim)
a = torch.softmax(self.v(e).transpose(1, 2), dim=2) # a shape: (batch_size, 1, seq_len)
v = torch.bmm(a, inputs).squeeze(1) # v shape: (batch_size, input_dim)
return v
class Seq2Seq(nn.Module):
def __init__(self, input_dim, output_dim, hidden_dim):
super(Seq2Seq, self).__init__()
self.encoder = nn.LSTM(input_dim, hidden_dim, batch_first=True)
self.decoder = nn.LSTM(output_dim, hidden_dim, batch_first=True)
self.attention = Attention(hidden_dim, hidden_dim)
self.fc = nn.Linear(hidden_dim, output_dim)
def forward(self, inputs, targets):
# inputs shape: (batch_size, seq_len, input_dim)
# targets shape: (batch_size, seq_len, output_dim)
encoder_outputs, _ = self.encoder(inputs)
decoder_outputs, _ = self.decoder(targets)
seq_len = decoder_outputs.size(1)
outputs = []
for t in range(seq_len):
context = self.attention(encoder_outputs)
decoder_input = decoder_outputs[:, t, :]
decoder_input = torch.cat((decoder_input, context), dim=1)
decoder_output, _ = self.decoder(decoder_input.unsqueeze(1))
output = self.fc(decoder_output.squeeze(1))
outputs.append(output)
return torch.stack(outputs, dim=1)
# 实例化模型
model = Seq2Seq(input_dim=20, output_dim=10, hidden_dim=128)
criterion = nn.CrossEntropyLoss()
optimizer = torch.optim.Adam(model.parameters())
# 训练模型
for epoch in range(10):
for inputs, targets in train_loader:
optimizer.zero_grad()
outputs = model(inputs, targets[:, :-1, :])
loss = criterion(outputs.reshape(-1, 10), targets[:, 1:, :].argmax(dim=2).reshape(-1))
loss.backward()
optimizer.step()
```
其中,`Attention`是一个自定义的Attention模块,`Seq2Seq`是一个基于LSTM和Attention的序列模型。在训练过程中,我们使用交叉熵损失函数计算模型的损失。
在语音信号处理中,Transformer模型如何通过Self-Attention机制提取特征,并在语音识别中发挥怎样的作用?
在语音信号处理领域,Transformer模型通过其核心组件Self-Attention机制,能够在处理语音信号时捕捉序列内各个时间步之间的依赖关系,这对于提取有效特征并进行准确的语音识别至关重要。Self-Attention机制允许模型在计算每个时间步的输出时,直接考虑整个序列的上下文信息,这通过计算Query(Q)、Key(K)和Value(V)三个向量来实现。每个Query向量都会与所有的Key向量计算相似度得分,然后这些得分会被用于加权Value向量,最终得到当前时间步的上下文表示。
参考资源链接:[李宏毅语音信号处理课程笔记:从Transformer到SpeechRecognition](https://wenku.csdn.net/doc/4quesaqmha?spm=1055.2569.3001.10343)
在《李宏毅语音信号处理课程笔记:从Transformer到SpeechRecognition》中,详尽地介绍了Transformer模型的结构和Self-Attention的工作原理。课程笔记中指出,Transformer模型由多个Encoder和Decoder层构成,每个层都包含Self-Attention机制。此外,引入Multi-Head Attention允许模型同时在不同的表示子空间并行计算Self-Attention,从而获得更丰富的特征表示。
对于语音识别任务,Transformer模型不仅可以用于特征提取,还能够集成到序列到序列的模型中,例如使用CTC、RNN-T或NeuralTransducer等架构。在这些模型中,Self-Attention机制有助于提高对声音数据的建模能力,特别是在复杂的语言环境下,例如多语种识别和噪声背景下的语音识别。
总体而言,Self-Attention机制使得Transformer模型在处理序列数据时具有极高的灵活性和效率,对于提取声音特征和实现高效准确的语音识别起着关键作用。学习者可以通过《李宏毅语音信号处理课程笔记:从Transformer到SpeechRecognition》更深入地了解Transformer模型在语音信号处理中的应用,进而掌握语音识别的核心技术和实践技巧。
参考资源链接:[李宏毅语音信号处理课程笔记:从Transformer到SpeechRecognition](https://wenku.csdn.net/doc/4quesaqmha?spm=1055.2569.3001.10343)
阅读全文
相关推荐
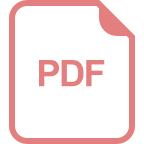
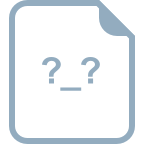
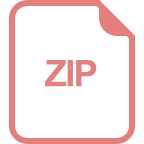


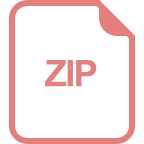
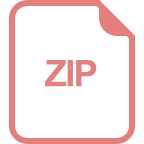
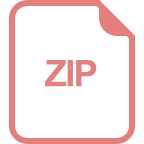
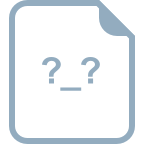
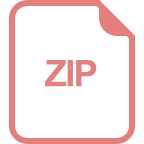
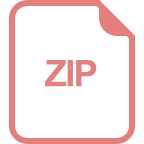
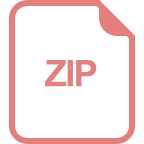




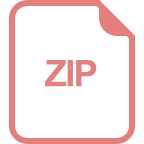