报错:AttributeError: module 'tensorflow' has no attribute 'placeholder'
时间: 2024-03-30 16:32:52 浏览: 176
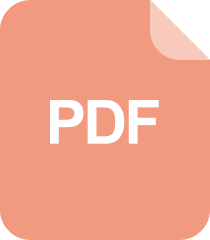
pycharm用import报错:AttributeError: module tensorflow(or other) has no attribut (import搜索路径顺序问题)
这个错误是由于在使用TensorFlow时,尝试访问`placeholder`属性时出现的。在TensorFlow 2.0版本及以上,`placeholder`已被移除,取而代之的是`tf.keras.Input`函数。`tf.keras.Input`函数用于定义模型的输入,可以指定输入的形状和数据类型。
如果你使用的是TensorFlow 1.x版本,可以通过以下方式解决该问题:
1. 确保你已正确导入TensorFlow模块:`import tensorflow as tf`
2. 检查是否正确使用了`placeholder`,例如:`x = tf.placeholder(tf.float32, shape=(None, 10))`
3. 如果以上步骤都正确无误,可能是因为TensorFlow版本不兼容导致的问题。你可以尝试升级或降级TensorFlow版本,或者查看官方文档以了解更多信息。
阅读全文
相关推荐
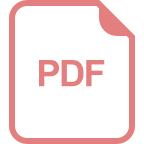
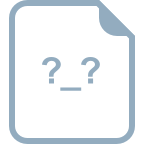











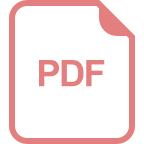
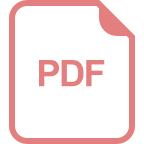
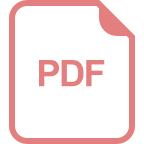
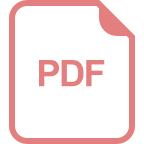
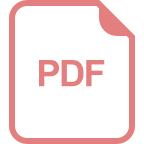