OpenCV DNN模块中的安全监控:保护你的世界,10个监控策略
发布时间: 2024-08-14 20:18:39 阅读量: 27 订阅数: 29 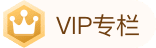
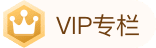
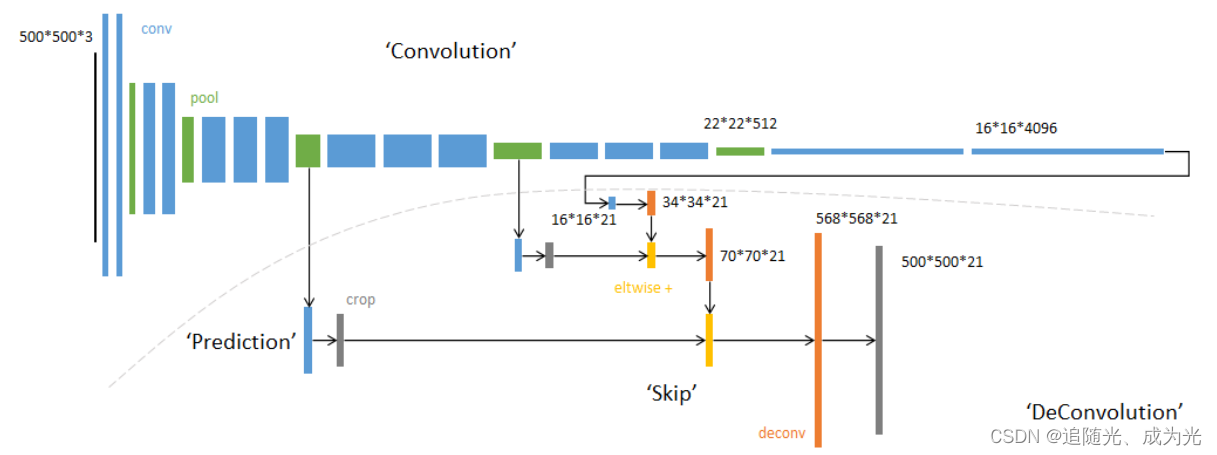
# 1. OpenCV DNN模块概述**
OpenCV DNN(深度神经网络)模块是一个用于计算机视觉和机器学习的强大库。它提供了一组丰富的函数和类,使开发人员能够轻松地构建、训练和部署DNN模型。DNN模块支持各种神经网络架构,包括卷积神经网络(CNN)、循环神经网络(RNN)和变压器网络。
DNN模块的主要优点之一是它与OpenCV库的无缝集成。这使开发人员能够利用OpenCV的图像处理和计算机视觉功能来构建强大的DNN应用程序。此外,DNN模块还支持多核并行处理,这可以显著提高推理速度。
# 2. 安全监控中的DNN模型**
DNN(深度神经网络)模型在安全监控领域发挥着至关重要的作用,为目标检测、人脸识别等任务提供强大的分析能力。本章将深入探讨用于安全监控的两种主要DNN模型类型:目标检测模型和人脸识别模型。
## 2.1 目标检测模型
目标检测模型用于识别和定位图像或视频帧中的特定对象。在安全监控中,目标检测模型可用于检测可疑人员、车辆或其他感兴趣的对象。
### 2.1.1 YOLOv3
YOLOv3(You Only Look Once version 3)是一种实时目标检测算法,因其速度快、准确性高而闻名。它使用单次卷积神经网络(CNN)来预测图像中对象的边界框和类概率。
**代码块:**
```python
import cv2
import numpy as np
# 加载 YOLOv3 模型
net = cv2.dnn.readNet("yolov3.weights", "yolov3.cfg")
# 加载图像
image = cv2.imread("image.jpg")
# 预处理图像
blob = cv2.dnn.blobFromImage(image, 1 / 255.0, (416, 416), (0, 0, 0), swapRB=True, crop=False)
# 设置输入
net.setInput(blob)
# 执行前向传播
detections = net.forward()
# 解析检测结果
for detection in detections[0, 0]:
score = float(detection[2])
if score > 0.5:
left, top, right, bottom = detection[3:7] * np.array([image.shape[1], image.shape[0], image.shape[1], image.shape[0]])
cv2.rectangle(image, (int(left), int(top)), (int(right), int(bottom)), (0, 255, 0), 2)
```
**逻辑分析:**
* `cv2.dnn.readNet()`:加载预训练的 YOLOv3 模型。
* `cv2.dnn.blobFromImage()`:将图像预处理为模型输入。
* `net.setInput()`:将预处理的图像设置为模型输入。
* `net.forward()`:执行前向传播,生成检测结果。
* 解析检测结果并绘制边界框。
### 2.1.2 Faster R-CNN
Faster R-CNN(Faster Region-based Convolutional Neural Network)是一种两阶段目标检测算法,在准确性方面比 YOLOv3 更胜一筹。它使用区域提议网络(RPN)生成候选边界框,然后使用 CNN 对这些边界框进行分类和回归。
**代码块:**
```python
import cv2
import numpy as np
# 加载 Faster R-CNN 模型
net = cv2.dnn.readNetFromTensorflow("faster_rcnn_inception_v2_coco.pb", "faster_rcnn_inception_v2_coco.pbtxt")
# 加载图像
image = cv2.imread("image.jpg")
# 预处理图像
blob = cv2.dnn.blobFromImage(image, 1 / 255.0, (600, 600), (0, 0, 0), swapRB=True, crop=False)
# 设置输入
net.setInput(blob)
# 执行前向传播
detections = net.forward()
# 解析检测结果
for detection in detections[0, 0]:
score = float(detection[2])
if score > 0.5:
left, top, right, bottom = detection[3:7] * np.array([image.shape[1], image.shape[0], image.shape[1], image.shape[0]])
cv2.rectangle(image, (int(left), int(top)), (int(right), int(bottom)), (0, 255, 0), 2)
```
**逻辑分析:**
* `cv2.dnn.readNetFromTensorflow()`:加载预训练的 Faster R-CNN 模型。
* `cv2.dnn.blobFromImage()`:将图像预处理为模型输入。
* `net.setInput()`:将预处理的图像设置为模型输入。
* `net.forward()`:执行前向传播,生成检测结果。
* 解析检测结果并绘制边界框。
## 2.2 人脸识别模型
人脸识别模型用于识别和验证图像或视频帧中的人员身份。在安全监控中,人脸识别模型可用于访问控制、人员追踪和身份验证。
### 2.2.1 FaceNet
FaceNet是一种用于
0
0
相关推荐
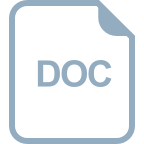
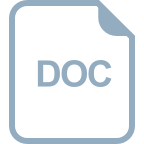
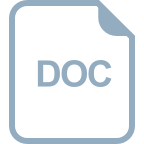
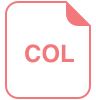
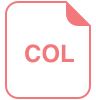
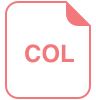
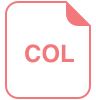
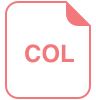
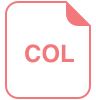