专家揭秘:Numeric库的扩展与自定义函数编写
发布时间: 2024-10-13 02:43:51 阅读量: 23 订阅数: 32 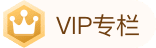
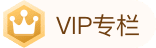
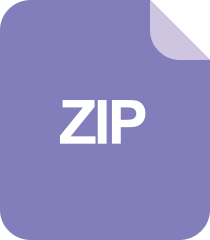
cpplib:编写一下cpp常用功能函数
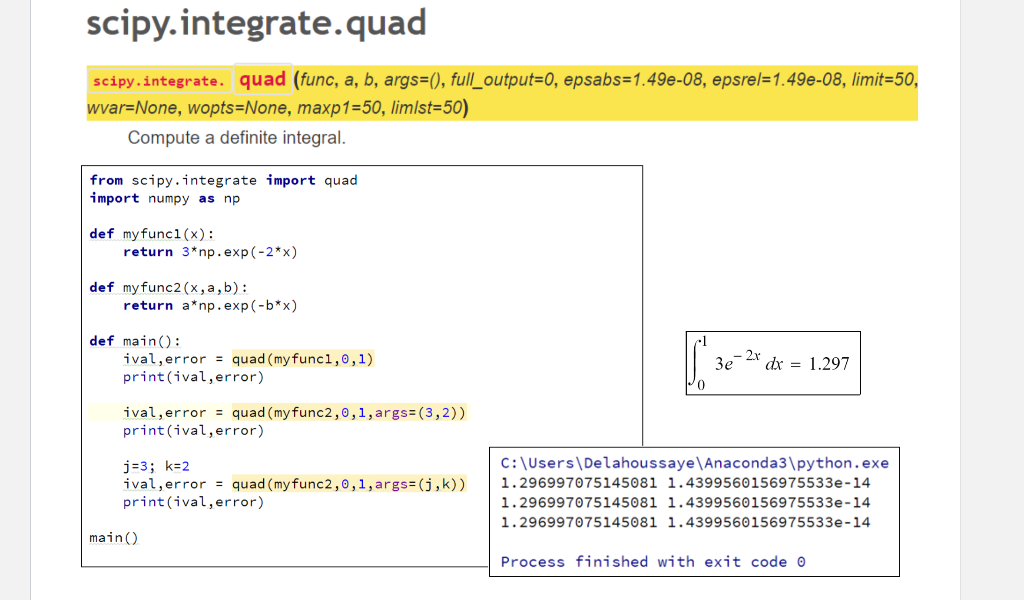
# 1. Numeric库的基础知识
## 1.1 Numeric库的介绍
Numeric库是Python中用于进行科学计算的一个基础库,它提供了强大的数值处理能力,特别是在矩阵运算和线性代数方面。该库广泛应用于数据分析、图像处理、机器学习等领域,是数据科学家和工程师不可或缺的工具之一。
## 1.2 核心功能
Numeric库的核心功能包括但不限于:
- 矩阵和向量的创建与操作
- 常用数学函数的实现
- 复数的支持
- 线性代数运算(如矩阵乘法、行列式计算等)
## 1.3 安装和导入
安装Numeric库非常简单,使用pip命令即可:
```bash
pip install numeric
```
安装完成后,就可以在Python脚本中导入使用了:
```python
import numeric
```
## 1.4 简单示例
下面是一个使用Numeric库进行矩阵创建和基本操作的简单示例:
```python
# 创建一个3x3的矩阵
matrix = numeric.array([[1, 2, 3], [4, 5, 6], [7, 8, 9]])
# 计算矩阵的转置
transpose = numeric.transpose(matrix)
# 打印结果
print("Original Matrix:\n", matrix)
print("Transpose:\n", transpose)
```
以上内容为基础章节的开头部分,简洁地介绍了Numeric库的背景、核心功能、安装方法以及一个简单的使用示例。这样的结构旨在帮助读者快速了解和掌握Numeric库的基础知识,为进一步学习和应用打下坚实的基础。
# 2. Numeric库的扩展方法
## 2.1 扩展库的创建和配置
### 2.1.1 创建自定义扩展库的步骤
创建自定义扩展库是提升Numeric库功能的一种有效方式。以下是创建自定义扩展库的基本步骤:
1. **确定需求和目标**:首先,你需要明确你的扩展库需要实现什么功能,解决什么问题。这一步是规划阶段,它将指导整个开发过程。
2. **创建项目结构**:在确定需求后,你需要创建项目的基本结构。这通常包括定义目录结构、初始化配置文件等。
3. **编写扩展代码**:根据需求编写具体的扩展代码。这部分是核心,需要你有扎实的编程基础和对Numeric库的深入理解。
4. **测试和调试**:编写单元测试来验证扩展功能的正确性,并进行调试以确保代码的健壮性。
5. **打包和发布**:将你的扩展库打包,确保它可以在其他项目中被引用和使用。你可以选择发布到公共仓库,如Python Package Index (PyPI)。
### 2.1.2 配置环境以支持扩展库
配置环境是确保扩展库能够被正确加载和使用的前提。以下是配置环境的基本步骤:
1. **安装Python和pip**:确保你的系统中安装了Python和pip工具,这是使用Python扩展库的基础。
2. **创建虚拟环境**:使用`virtualenv`或`conda`创建一个虚拟环境,以隔离项目依赖,避免版本冲突。
3. **安装依赖**:在你的项目中创建一个`requirements.txt`文件,列出所有需要的依赖,并通过`pip install -r requirements.txt`安装它们。
4. **安装扩展库**:如果扩展库已经被打包发布,你可以通过`pip install <package_name>`安装它。如果是本地开发版本,可以使用`pip install -e .`命令进行本地安装。
### 2.1.3 示例代码和解释
以下是一个简单的示例,展示了如何创建一个简单的自定义扩展库,并在环境中进行配置。
```python
# my_numeric_extension/__init__.py
def my_custom_function(value):
"""
A custom function that extends the Numeric library.
:param value: The input value to be processed.
:return: The processed value.
"""
# Perform some operations and return the result
return value * 2
```
在上述代码中,我们创建了一个名为`my_numeric_extension`的目录,该目录包含一个`__init__.py`文件,定义了一个简单的自定义函数`my_custom_function`。
接下来,我们需要在`requirements.txt`中添加以下内容:
```
# requirements.txt
my-numeric-extension==0.1
```
然后,你可以通过以下命令安装依赖并测试你的扩展库:
```bash
# Create a virtual environment (assuming virtualenv is installed)
virtualenv venv
source venv/bin/activate # On Windows use `venv\Scripts\activate`
# Install dependencies
pip install -r requirements.txt
# Test the extension
python -c "from my_numeric_extension import my_custom_function; print(my_custom_function(10))"
```
在上述步骤中,我们创建了一个虚拟环境,安装了依赖,并测试了我们的扩展函数。
## 2.2 扩展Numeric库的核心函数
### 2.2.1 重载和自定义核心函数
通过重载和自定义核心函数,我们可以扩展Numeric库的功能,使其适应特定的计算需求。以下是如何重载和自定义核心函数的步骤:
1. **确定要重载的函数**:首先,你需要确定要重载的核心函数。这通常基于你的具体需求。
2. **编写重载函数**:编写一个新的函数,该函数具有与原函数相同的名称和参数列表。在函数体内,你可以添加额外的逻辑来改变原有的行为。
3. **注册重载函数**:使用Numeric库提供的机制将你的重载函数注册到核心函数中。
### 2.2.2 实现特定功能的自定义函数
为了实现特定功能,我们通常需要编写自定义函数,并将其集成到Numeric库中。以下是实现步骤:
1. **定义函数功能**:明确你想要实现的功能,这将指导你编写函数的逻辑。
2. **编写函数代码**:根据功能需求编写函数的代码。
3. **集成到Numeric库**:将你的自定义函数集成到Numeric库中,使其可以被调用和使用。
### 2.2.3 示例代码和解释
以下是一个示例,展示了如何重载`sum`函数,并添加一个自定义函数。
```python
import numpy
# Overload the built-in sum function
def overloaded_sum(array):
"""
Overloaded sum function that performs additional checks.
:param array: The array to sum.
:return: The sum of the array elements.
"""
if not isinstance(array, numpy.ndarray):
raise TypeError("Input must be a numpy.ndarray")
# Perform additional checks or preprocessing
return numpy.sum(array)
# Register the overloaded function
numpy.lib.mixins._wrapit(numpy.sum, overloaded_sum)
# Custom function to calculate the sum of squares
def sum_of_squares(array):
"""
Custom function to calculate the sum of squares of array elements.
:param array: The array to calculate the sum of squares.
:return: The sum of squares.
"""
return numpy.sum(numpy.square(array))
# Test the overloaded and custom functions
test_array = numpy.array([1, 2, 3, 4])
print("Overloaded sum:", overloaded_sum(test_array))
print("Sum of squares:", sum_of_squares(test_array))
```
在上述代码中,我们首先重载了`numpy.sum`函数,添加了类型检查。然后,我们定义了一个计算平方和的自定义函数`sum_of_squares`。最后,我们测试了这两个函数。
## 2.3 扩展库的性能优化
### 2.3.1 性能监控和分析
性能监控和分析是优化扩展库的关键步骤。以下是进行性能监控和分析的基本步骤:
1. **监控性能指标**:监控扩展库的关键性能指标,如执行时间、内存使用等。
2. **使用分析工具**:使用性能分析工具,如`cProfile`、`line_profiler`等,来识别瓶颈。
3. **记录和比较**:记录性能数据,并与未优化的版本进行比较。
#
0
0
相关推荐







