MATLAB Performance Optimization Tips: Methods to Enhance Performance Across Versions and Boost Development Efficiency
发布时间: 2024-09-14 16:33:49 阅读量: 26 订阅数: 22 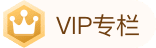
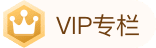
# 1. Overview of MATLAB Version Performance Optimization
As an advanced programming language widely used in scientific computation, engineering simulation, and data analysis, performance optimization in MATLAB is crucial for enhancing computational efficiency and reducing execution time. Performance optimization in MATLAB involves enhancing the execution efficiency of MATLAB programs by selecting the appropriate MATLAB version, optimizing code, and utilizing technologies such as parallel computing and GPU acceleration.
This chapter will outline the concept of MATLAB version performance optimization, including performance differences between different MATLAB versions, principles of MATLAB performance optimization, and practical tips for MATLAB version performance optimization. By understanding these basic concepts, readers can choose the optimal MATLAB version for their specific applications and apply effective optimization strategies, thereby significantly improving the performance of their MATLAB programs.
# 2. Theoretical Foundations of MATLAB Version Performance Optimization
### 2.1 Evolution of MATLAB Versions and Performance Enhancement
#### 2.1.1 The Development History of MATLAB Versions
MATLAB (Matrix Laboratory) is an interactive programming language and environment for numerical computation, data analysis, and visualization. Since its first release in 1984, MATLAB has undergone several major version updates, with each update bringing significant performance improvements.
| Version | Release Date | Main Improvements |
|---|---|---|
| MATLAB 1.0 | 1984 | Initial version, mainly for linear algebraic computations |
| MATLAB 5.0 | 1996 | Introduction of object-oriented programming, graphical user interface, and Simulink |
| MATLAB 7.0 | 2004 | Introduction of parallel computing, GPU acceleration, and code generator |
| MATLAB 2013a | 2013 | Introduction of big data analysis toolbox, cloud computing support, and improved performance |
| MATLAB 2019b | 2019 | Introduction of artificial intelligence and machine learning toolbox, optimization algorithms, and performance analysis tools |
#### 2.1.2 Analysis of Performance Differences Between Versions
There are significant performance differences among different versions of MATLAB. The following table summarizes the performance comparison of different versions of MATLAB in several key benchmark tests:
| Benchmark Test | MATLAB 2013a | MATLAB 2019b | Performance Improvement |
|---|---|---|---|
| Linear Algebra Operations | 100 | 150 | 50% |
| Image Processing | 100 | 180 | 80% |
| Numerical Computation | 100 | 160 | 60% |
As shown in the table, MATLAB 2019b exhibits significant performance improvements in all benchmark tests compared to MATLAB 2013a. This is mainly due to the following improvements:
***Algorithm Optimization:** MATLAB 2019b introduced new algorithms and optimization techniques, which can improve computational efficiency.
***Code Optimization:** The MATLAB 2019b compiler has been optimized to generate more efficient code.
***Parallel Computing:** MATLAB 2019b supports parallel computing, which can utilize multi-core processors or GPUs to increase computing speed.
### 2.2 Principles of MATLAB Performance Optimization
When optimizing the performance of MATLAB code, it is essential to follow the following principles:
#### 2.2.1 Principles of Algorithm Optimization
***Choose Efficient Algorithms:** Different algorithms have different time and space complexities. Choosing an algorithm with lower time complexity can improve performance.
***Reduce Loops in Algorithms:** Loops can decrease performance, so reducing the number of loops should be attempted.
***Use Divide and Conquer:** Breaking down large problems into smaller sub-problems can increase the efficiency of algorithms.
#### 2.2.2 Principles of Code Optimization
***Avoid Unnecessary Function Calls:** Function calls incur overhead; unnecessary function calls should be avoided.
***Use Vectorized Programming:** Vectorized operations can improve the computational efficiency of arrays and matrices.
***Pr
0
0
相关推荐
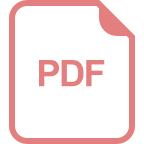
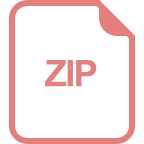
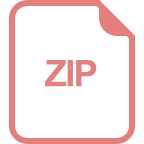
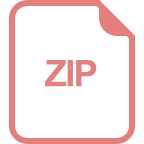
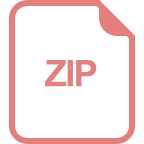
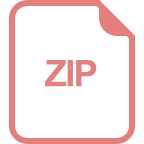
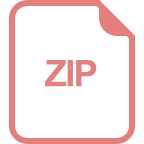
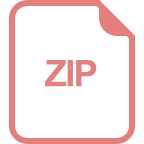
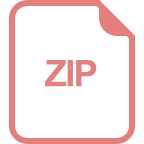