The Evolutionary History of MATLAB Versions: From the First to the Latest, Unveiling the Journey of Version Evolution
发布时间: 2024-09-14 16:01:41 阅读量: 21 订阅数: 25 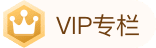
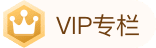
# A Brief History of MATLAB Evolution: From the First Generation to the Latest Version
MATLAB (Matrix Laboratory) is a programming language and interactive environment widely used for technical computing. Developed by MathWorks, it was initially designed for matrix operations and linear algebra computations. Over time, MATLAB has evolved into a powerful tool encompassing a wide range of scientific, engineering, and data analysis fields.
The evolution of MATLAB versions:
***First Generation MATLAB (1984):** Laid the foundation for MATLAB, focusing on matrix operations and numerical computation.
***MATLAB 5.0 (1996):** Introduced a graphical user interface (GUI), making MATLAB more user-friendly and adding new features such as symbolic math and image processing.
***MATLAB 7.0 (2004):** Introduced the concept of object-oriented programming (OOP), enabling MATLAB to create and manage complex data structures and algorithms.
***MATLAB 2008a (2008):** The release of the Parallel Computing Toolbox brought parallel computing features to MATLAB, supporting multicore and distributed computing.
# The Evolution Journey of MATLAB Versions
### 2.1 First Generation MATLAB: Laying the Foundation
#### 2.1.1 Core Functions and Features
The first generation of MATLAB was released in 1984 and is the ancestor of the MATLAB family. It laid the foundation for MATLAB as a language for technical computing, providing a range of core functions including:
- **Matrix operations:** MATLAB is renowned for its powerful matrix operations, supporting various matrix calculations such as addition, subtraction, multiplication, inverse operations, and determinant calculations.
- **Numerical analysis:** MATLAB includes a wide array of numerical analysis functions for solving equation systems, optimization problems, and performing data analysis.
- **Visualization:** The initial MATLAB version provided basic plotting functions, allowing users to create simple graphs and charts.
#### 2.1.2 Impact and Limitations
The first generation of MATLAB had a significant impact in the field of technical computing. It offered an easy-to-use interface and powerful matrix operation capabilities, enabling researchers and engineers to efficiently solve complex problems. However, it also had some limitations:
- **Limited graphical capabilities:** The graphical features of the first generation MATLAB were relatively simple and unable to create complex or interactive graphics.
- **Lack of object-oriented programming:** The initial MATLAB did not support object-oriented programming (OOP), limiting its extensibility and code reusability.
- **Memory constraints:** The limited memory capacity of the first generation MATLAB restricted its ability to handle large datasets.
### 2.2 MATLAB 5.0: The Graphical Revolution
#### 2.2.1 Introduction of Graphical User Interface (GUI)
MATLAB 5.0 was released in 1996, introducing a graphical user interface (GUI), which revolutionized the user experience of MATLAB. The GUI featured intuitive menus, toolbars, and dialog boxes, making it easy for non-programmers to use MATLAB.
#### 2.2.2 New Features and Enhancements
Besides the GUI, MATLAB 5.0 introduced many new features and enhancements, including:
- **Simulink:** Simulink is a graphical modeling and simulation environment that allows users to create and simulate dynamic systems.
- **Toolboxes:** MATLAB 5.0 introduced the concept of toolboxes, providing additional functionalities for specific domains such as signal processing, image processing, and control systems.
- **Performance optimizations:** MATLAB 5.0 saw significant performance improvements, enabling it to handle larger datasets and more complex computations.
### 2.3 MATLAB 7.0: Introduction of Object-Oriented Programming (OOP)
#### 2.3.1 Integration of OOP Concepts
MATLAB 7.0 was released in 2004, introducing the concept of object-oriented programming (OOP). OOP allows users to create and use classes and objects, enhancing code reusability, scalability, and maintainability.
#### 2.3.2 Application of Classes and Objects
In MATLAB 7.0, classes and objects can be used to represent various data structures and algorithms. For example, users can create custom classes to represent physical objects or mathematical models.
### 2.4 MATLAB 2008a: Breakthrough in Parallel Computing
#### 2.4.1 Release of Parallel Computing Toolbox
MATLAB 2008a was released in 2008, introducing the Parallel Computing Toolbox. This toolbox provides support for multicore processors and distributed computing, allowing MATLAB to handle larger datasets and more complex computations.
#### 2.4.2 Support for Multicore and Distributed Computing
The Parallel Computing Toolbox allows users to execute code in parallel on multicore processors and employ distributed computing techniques to distribute tasks across multiple computers. This greatly enhances the computational performance of MATLAB.
# 3. Trends in MATLAB Version Evolution
The evolution of MATLAB versions reflects the evolving needs of computational technology and scientific computing fields. With the rise of cloud computing, artificial intelligence (AI), machine learning (ML), big data analysis, and visualization, MATLAB continuously adapts to these trends, providing tools and functionalities to meet the changing demands of its users.
### 3.1 Cloud Computing and Distributed Computing
**Integration with Cloud Platforms**
MATLAB integrates with cloud platforms such as Amazon Web Services (AWS), Microsoft Azure, and Google Cloud Platform, allowing users to access and use MATLAB on the cloud. This offers several advantages:
- **Scalability:** Cloud platforms provide on-demand scalable computing resources, enabling users to expand or reduce their computing capabilities as needed.
- **Cost-effectiveness:** Cloud platforms charge based on usage, so users only pay for the resources they consume, thereby reducing costs.
- **Collaboration:** Cloud platforms enable multiple users to collaborate on projects, easily sharing data and results.
**Expansion of Distributed Computing**
The MATLAB Parallel Computing Toolbox has been expanded to support computations on distributed systems. This allows users to leverage multiple computing nodes to solve complex problems, significantly reducing computation time.
**Code Block:**
```matlab
% Create a distributed computing job
job = createParallelJob();
% Add tasks to the job
addTask(job, @myFunction, 100);
% Submit the job
submit(job);
% Wait for the job to complete
waitFor(job);
% Retrieve results
results = getAllOutputArguments(job);
```
**Logical Analysis:**
This code block demonstrates how to use the Parallel Computing Toolbox to create and submit jobs on a distributed system. The `createParallelJob` function creates a job object, `addTask` function adds tasks to the job, `submit` function submits the job, `waitFor` function waits for the job to complete, and `getAllOutputArguments` function retrieves the results of the job.
### 3.2 Artificial Intelligence (AI) and Machine Learning (ML)
**Introduction of AI and ML Algorithms**
MATLAB has introduced a wide range of AI and ML algorithms, including:
- **Supervised learning:** Linear regression, logistic regression, support vector machines.
- **Unsupervised learning:** Clustering, principal component analysis, singular value decomposition.
- **Deep learning:** Convolutional neural networks, recurrent neural networks, generative adversarial networks.
**Support for Deep Learning and Neural Networks**
MATLAB provides specialized toolboxes for deep learning and neural networks, including:
- **Deep Learning Toolbox:** Offers functions and tools for creating and training deep learning models.
- **Neural Network Toolbox:** Provides functions and tools for creating and training neural networks.
**Code Block:**
```matlab
% Create a neural network
net = feedforwardnet([10 10 1]);
% Train the neural network
net = train(net, inputs, targets);
% Use the neural network for prediction
outputs = net(inputs);
```
**Logical Analysis:**
This code block demonstrates how to use the Neural Network Toolbox to create, train, and use a neural network. The `feedforwardnet` function creates a feedforward neural network, the `train` function trains the neural network, and the `net` function uses the neural network for prediction.
### 3.3 Big Data Analysis and Visualization
**Enhancements to Big Data Processing Tools**
MATLAB has enhanced its big data processing tools, including:
- **Parallel Computing Toolbox:** Supports distributed big data processing.
- **Big Data Toolbox:** Provides functions for processing and analyzing big datasets.
**Optimization of Interactive Data Visualization**
MATLAB has improved its data visualization capabilities, including:
- **Interactive charts:** Allow users to zoom, pan, and rotate charts.
- **Data Explorer:** Offers interactive tools for exploring and visualizing data.
**Code Block:**
```matlab
% Create an interactive chart
figure;
plot(data);
xlabel('X');
ylabel('Y');
title('Data Plot');
% Make the chart interactive
set(gca, 'Interactive', 'on');
```
**Logical Analysis:**
This code block demonstrates how to create an interactive chart. The `figure` function creates a graphical window, the `plot` function plots data, the `xlabel`, `ylabel`, and `title` functions set axis labels and titles, and the `set` function makes the chart interactive.
# 4. Practical Applications of MATLAB Version Evolution
The evolution of MATLAB has not only brought about technological advancements but has also significantly expanded its application scope across various fields. Here are a few key practical applications of MATLAB in different industries:
### 4.1 Scientific Computing and Engineering Modeling
MATLAB has extensive applications in scientific computing and engineering modeling. Its powerful numerical analysis and solver capabilities make it an ideal tool for solving complex mathematical problems.
#### Numerical Analysis and Solvers
MATLAB provides a range of numerical analysis tools, including linear algebra, calculus, optimization, and statistics. These tools can be used to solve various problems, such as solving equation systems, fitting curves, and optimizing functions.
```
% Solve a system of linear equations
A = [2 1; 3 4];
b = [5; 7];
x = A\b;
% Fit a curve
xdata = [0:0.1:10];
ydata = sin(xdata);
fitresult = fit(xdata', ydata', 'poly3');
```
#### Physical Modeling and Simulation
MATLAB is also widely used for physical modeling and simulation. Its Simulink module offers a graphical environment for creating and simulating dynamic systems. Simulink can be used to simulate mechanical, electrical, thermodynamic, and control systems.
```
% Create a simple mechanical system model
m = 1; % Mass (kilograms)
k = 10; % Spring constant (Newtons/meter)
b = 1; % Damping coefficient (Newtons·seconds/meter)
% Simulate the system
sim('mass_spring_damper.slx');
```
### 4.2 Data Analysis and Machine Learning
MATLAB also plays a crucial role in data analysis and machine learning. Its powerful data processing tools and machine learning algorithm library make it an ideal platform for processing and analyzing large datasets.
#### Statistical Analysis and Data Mining
MATLAB offers a range of statistical analysis and data mining tools, including descriptive statistics, hypothesis testing, clustering, and classification. These tools can be used for data exploration, pattern recognition, and trend prediction.
```
% Import data
data = importdata('data.csv');
% Compute descriptive statistics
mean_value = mean(data);
median_value = median(data);
std_dev = std(data);
% Perform clustering analysis
[idx, C] = kmeans(data, 3);
```
#### Implementation of Machine Learning Algorithms
MATLAB also provides a comprehensive library of machine learning algorithms, including supervised learning (such as regression, classification, and support vector machines) and unsupervised learning (such as clustering and dimensionality reduction). These algorithms can be used to build predictive models, recognize patterns, and perform data exploration.
```
% Train a linear regression model
X = [ones(size(data, 1), 1), data(:, 1)];
y = data(:, 2);
model = fitlm(X, y);
% Predict new data
new_data = [1, 10];
predicted_value = predict(model, new_data);
```
### 4.3 Image Processing and Computer Vision
MATLAB is also powerful in the fields of image processing and computer vision. Its image processing toolbox provides various image processing and analysis algorithms, while the computer vision toolbox provides algorithms for image recognition, object detection, and image segmentation.
#### Image Processing Toolbox
MATLAB's image processing toolbox offers a wide range of image processing capabilities, including image enhancement, filtering, transformation, and segmentation. These capabilities can be used to improve image quality, extract features, and perform image analysis.
```
% Read an image
image = imread('image.jpg');
% Convert the image to grayscale
gray_image = rgb2gray(image);
% Apply Gaussian filtering
filtered_image = imgaussfilt(gray_image, 2);
```
#### Application of Computer Vision Algorithms
MATLAB's computer vision toolbox provides algorithms for image recognition, object detection, and image segmentation. These algorithms can be used to automate visual tasks such as face recognition, object detection, and scene understanding.
```
% Use deep learning for image classification
net = alexnet;
image_size = net.Layers(1).InputSize;
image_data = imresize(image, image_size);
[label, scores] = classify(net, image_data);
```
# 5. Future Outlook for MATLAB Version Evolution
### 5.1 Continuous Innovation and Optimization
As a leading technical computing platform, MATLAB is committed to continuous innovation and optimization to meet the changing needs of users. In the future, MATLAB version evolution will focus on the following aspects:
- **Addition of new features and algorithms:** MATLAB will continuously expand its function library by introducing new algorithms and tools to meet the latest demands in scientific computing, data analysis, and machine learning.
- **Performance and efficiency improvements:** MATLAB will continue to optimize its code and algorithms to increase execution speed and memory efficiency, providing users with a smoother and faster computing experience.
### 5.2 Integration with Other Technologies and Platforms
MATLAB is actively integrating with other technologies and platforms to enhance its functionality and expand its application range. In the future, MATLAB version evolution will focus on the following aspects:
- **Interoperability with Python and R:** MATLAB will enhance interoperability with other popular programming languages such as Python and R, allowing users to seamlessly integrate MATLAB code with other languages, achieving more robust data analysis and modeling capabilities.
- **Application in cloud platforms and the Internet of Things (IoT):** MATLAB will further extend its application in cloud platforms and IoT, enabling users to perform large-scale computing and data analysis in distributed environments.
### 5.3 Improvement of User Experience and Education
MATLAB is dedicated to providing users with an excellent user experience and abundant educational resources to lower the barriers to learning and using the platform. In the future, MATLAB version evolution will focus on the following aspects:
- **Simplified user interface:** MATLAB will continuously simplify its user interface to make it easier for new users to get started and increase the efficiency of experienced users.
- **Rich educational resources:** MATLAB will continue to provide abundant educational resources, including tutorials, documentation, and online courses, to help users quickly master the powerful features of MATLAB.
# 6. The Impact of MATLAB Version Evolution on Industries
The evolution of MATLAB versions has had a profound impact on various industries, especially in scientific computing, engineering, data analysis, and machine learning.
**Scientific Computing and Engineering**
MATLAB plays a crucial role in scientific computing and engineering. Its powerful numerical analysis and solver capabilities enable researchers and engineers to solve complex problems, such as finite element analysis, fluid dynamics modeling, and optimization. With the evolution of MATLAB versions, these functions have been continuously enhanced, improving computational accuracy and efficiency.
**Data Analysis and Machine Learning**
MATLAB has also made significant progress in data analysis and machine learning. It offers a wide range of statistical analysis and data mining tools, allowing data scientists to extract valuable insights from large datasets. Additionally, MATLAB supports various machine learning algorithms, including supervised learning, unsupervised learning, and deep learning.
**Image Processing and Computer Vision**
MATLAB is widely used in image processing and computer vision. Its image processing toolbox provides rich features for image enhancement, segmentation, and feature extraction. The introduction of computer vision algorithms, such as object detection and image classification, has made MATLAB a powerful tool in the field of computer vision.
**Industry Trends**
The trends in MATLAB version evolution have impacted industries in the following ways:
***Cloud Computing and Distributed Computing:** The integration of MATLAB with cloud platforms like AWS and Azure enables researchers and engineers to access powerful computing resources to process large datasets and perform complex computations.
***Artificial Intelligence (AI) and Machine Learning (ML):** MATLAB's support for AI and ML algorithms enables researchers and engineers to develop and deploy intelligent systems, automate tasks, and improve decision-making.
***Big Data Analysis and Visualization:** The enhancements to MATLAB's big data processing tools allow researchers and engineers to process and analyze vast amounts of data and gain valuable insights through interactive data visualization features.
**Conclusion**
The evolution of MATLAB versions has had a transformative impact on industries, providing researchers, engineers, and data scientists with powerful tools to solve complex problems, analyze data, and develop intelligent systems. As MATLAB continues to innovate and optimize, its influence in the industry is expected to grow even further.
0
0
相关推荐
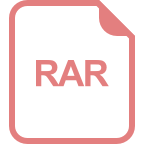
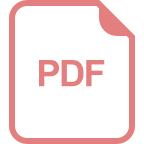
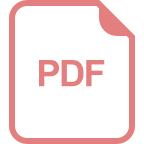
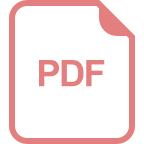
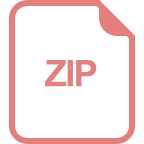
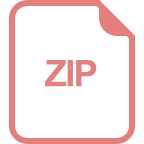
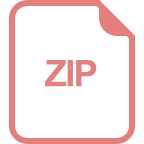
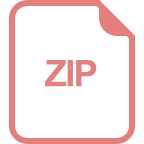
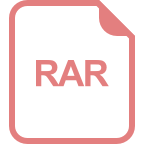