TensorFlow安装性能优化秘诀:让你的安装飞起来
发布时间: 2024-06-22 12:49:31 阅读量: 90 订阅数: 36 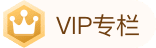
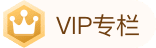
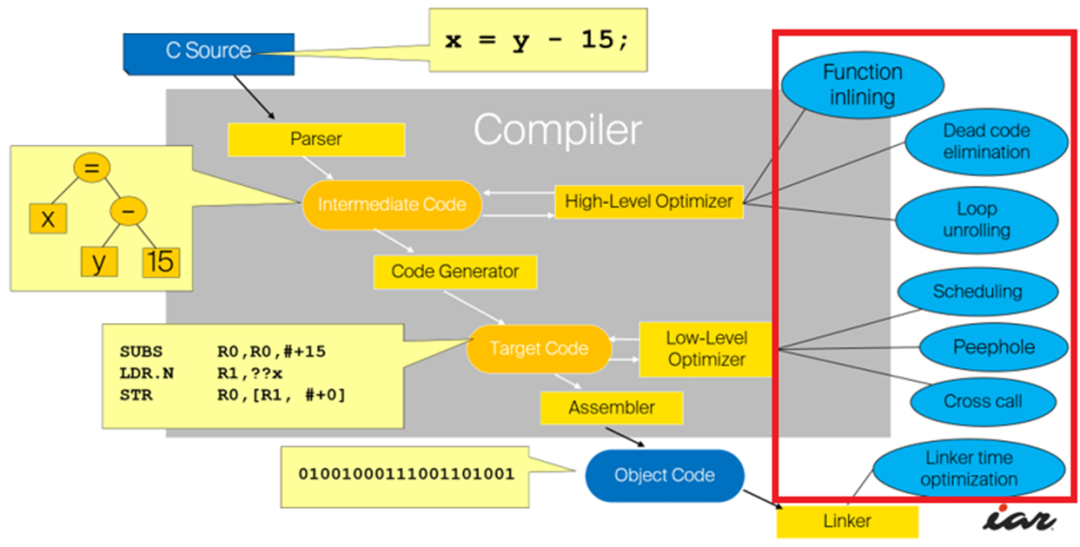
# 1. TensorFlow简介**
TensorFlow是一个开源机器学习库,用于构建和训练神经网络模型。它由Google开发,是目前最流行的机器学习库之一。TensorFlow以其灵活性、可扩展性和高性能而闻名。
TensorFlow使用数据流图模型来表示计算。数据流图由节点和边组成,其中节点表示操作,边表示数据流。这种模型使TensorFlow能够轻松地并行化和分布式计算,从而提高性能。
TensorFlow广泛应用于各种机器学习任务,包括图像识别、自然语言处理和语音识别。它还被用于强化学习和生成式对抗网络等高级机器学习技术。
# 2. TensorFlow安装优化
### 2.1 TensorFlow安装环境要求
**2.1.1 系统要求**
| 操作系统 | 最低版本 | 推荐版本 |
|---|---|---|
| Windows | Windows 7 64位 | Windows 10 64位 |
| macOS | macOS 10.12 | macOS 12 |
| Linux | Ubuntu 16.04 LTS | Ubuntu 20.04 LTS |
**2.1.2 硬件要求**
| 组件 | 最低要求 | 推荐要求 |
|---|---|---|
| CPU | 2核 | 4核或以上 |
| 内存 | 8GB | 16GB或以上 |
| 硬盘空间 | 50GB | 100GB或以上 |
| 显卡 | 无 | NVIDIA GeForce GTX 1080 Ti或以上 |
### 2.2 TensorFlow安装方式
TensorFlow提供多种安装方式,包括:
**2.2.1 Pip安装**
```
pip install tensorflow
```
**2.2.2 Docker安装**
```
docker pull tensorflow/tensorflow
docker run -it tensorflow/tensorflow
```
**2.2.3 源码编译安装**
```
git clone https://github.com/tensorflow/tensorflow.git
cd tensorflow
./configure
make
make install
```
### 2.3 TensorFlow安装优化技巧
**2.3.1 选择合适的安装方式**
根据具体需求和环境选择合适的安装方式。对于快速安装和易于管理,推荐使用Pip安装。对于隔离性和可移植性,推荐使用Docker安装。对于定制化和性能优化,推荐使用源码编译安装。
**2.3.2 优化安装环境**
* 确保系统满足最低要求,并根据推荐要求进行优化。
* 安装必要的依赖库,如CUDA和cuDNN。
* 配置环境变量,如`PATH`和`LD_LIBRARY_PATH`。
**2.3.3 启用GPU加速**
如果使用NVIDIA显卡,可以启用GPU加速以提升训练和推理性能。
```
import tensorflow as tf
# 创建GPU设备
gpu_device = tf.config.list_physical_devices('GPU')[0]
# 限制GPU内存使用
tf.config.experimental.set_memory_growth(gpu_device, True)
```
# 3. TensorFlow性能优化
### 3.1 TensorFlow模型优化
TensorFlow模型优化旨在减小模型的大小和计算复杂度,同时保持或提高其准确性。常用的模型优化技术包括:
**3.1.1 模型压缩**
模型压缩通过移除冗余参数和结构来减小模型的大小。常用的模型压缩技术包括:
- **剪枝:**移除对模型性能影响较小的权重和神经元。
- **量化:**将浮点权重和激活转换为低精度整数,从而减少存储和计算成本。
- **蒸馏:**使用较小的学生模型从较大的教师模型中学习知识,从而获得更紧凑的模型。
**3.1.2 模型量化**
模型量化将浮点权重和激活转换为低精度整数,例如 int8 或 int16。这可以显著减少模型的大小和计算成本,而对准确性影响较小。
```python
import tensorflow as tf
# 创建一个浮点模型
model = tf.keras.models.Sequential([
tf.keras.layers.Dense(10, activation='relu'),
tf.keras.layers.Dense(10, activation='softmax')
])
# 量化模型
quantized_model = tf.keras.models.quantization.quantize_model(model)
# 评估量化模型的准确性
loss, accuracy = quantized_model.evaluate(x_test, y_test)
print(f'Loss: {loss}, Accuracy: {accuracy}')
```
**3.1.3 模型蒸馏**
模型蒸馏使用较小的学生模型从较大的教师模型中学习知识。通过最小化学生模型的输出与教师模型输出之间的差异,学生模型可以获得与教师模型相似的性能,同时具有更小的尺寸。
```python
import tensorflow as tf
# 创建教师模型
teacher_model = tf.keras.models.Sequential([
tf.keras.layers.Dense(10, activation
```
0
0
相关推荐
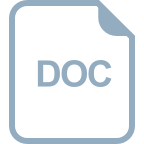
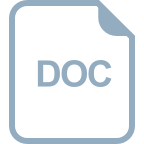
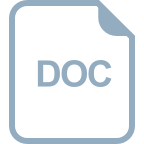
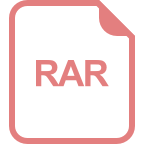
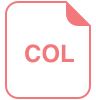
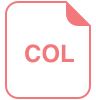
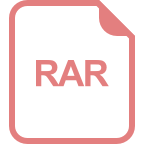
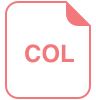
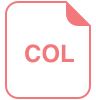