Unveiling the Significant Technical Differences in MATLAB Versions: Performance Enhancements and Functional Upgrades in New Releases
发布时间: 2024-09-14 01:26:08 阅读量: 25 订阅数: 30 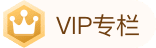
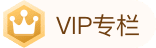
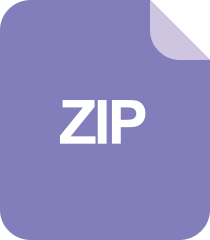
java计算器源码.zip
**MATLAB Version Evolution and Technical Overview**
MATLAB, a programming language and interactive environment extensively used in scientific computing, engineering simulation, and data analysis, has continuously evolved to deliver performance enhancements and feature improvements with each new version. From its early iterations to the latest releases, MATLAB has made significant strides in computational engines, graphic visualization, artificial intelligence, and cloud computing.
The evolution of MATLAB versions follows several technical trends:
- **Enhanced Parallel Computing Capabilities:** MATLAB has significantly improved its parallel computing performance by leveraging multicore processors and GPU acceleration, drastically reducing the processing time for large datasets and complex model solving.
- **Optimized Memory Management:** MATLAB has optimized memory allocation and management, reducing memory overhead and enhancing program execution efficiency, especially when handling large data and complex algorithms.
**2. Performance Enhancements in New Versions**
The new version of MATLAB has undergone various optimizations in performance, aiming to enhance computational efficiency and graphical processing capabilities.
### 2.1 Computational Engine Optimization
#### 2.1.1 Parallel Computing Capability Enhancement
The new version of MATLAB has notably increased the execution speed for processing large datasets and complex computational tasks through enhanced parallel computing functionalities.
- **Optimized Parallel Pool Management:** The new version has refined parallel pool management, allowing users to allocate and manage computing resources more effectively, thereby maximizing parallel computing efficiency.
- **Enhanced Multicore Support:** MATLAB now supports processors with more cores, enabling users to fully utilize the parallel processing capabilities of modern computing hardware.
- **Improved Code Parallelization Tools:** MATLAB offers new tools and syntactic features that simplify the code parallelization process, making it easier for developers to create scalable parallel applications.
#### 2.1.2 Memory Management Optimization
The new version of MATLAB has also improved its memory management mechanisms to enhance the efficiency of handling large datasets.
- **Optimized Memory Allocation:** MATLAB has refined its memory allocation algorithms, reducing memory fragmentation and improving memory utilization, thus avoiding unnecessary memory overhead.
- **Improved Garbage Collection Mechanism:** The new version has enhanced the garbage collection mechanism, more effectively releasing unused memory and reducing the likelihood of memory leaks.
- **Enhanced Large Memory Support:** MATLAB now supports processing larger datasets, allowing users to handle more significant amounts of data in memory, thereby increasing computational efficiency.
### 2.2 Enhanced Graphics and Visualization
#### 2.2.1 Interactive Visualization Tools
The new version of MATLAB introduces new interactive visualization tools, making data exploration and visualization more efficient.
- **Visualization Editor:** The visualization editor provides an interactive environment that allows users to easily create and modify charts and graphs and view changes in real-time.
- **Data Browser:** The Data Browser is an interactive tool that enables users to explore and filter large datasets and quickly generate visual representations.
- **Visualization Scripting:** MATLAB now supports visualization scripting, allowing users to create and control visualizations using scripting languages, enabling more complex interactions and automation.
#### 2.2.2 3D Drawing Performance Optimization
The new version of MATLAB has also optimized 3D drawing performance, providing a smoother and more realistic visualization experience.
- **GPU Acceleration:** MATLAB now supports the use of Graphics Processing Units (GPUs) for 3D drawing, significantly increasing the rendering speed for large and complex 3D scenes.
- **Anti-aliasing and Shadow Enhancement:** The new version has improved anti-aliasing and shadow algorithms, resulting in more realistic 3D graphics with reduced jagged edges and distortion.
- **Interactive 3D Navigation:** MATLAB offers new interactive 3D navigation tools that allow users to easily rotate, pan, and zoom 3D scenes, providing a better visualization experience.
**3. New Version Functional Enhancements**
The new version of MATLAB has also significantly improved its functionality, introducing numerous practical tools and features to meet users' application needs in areas such as artificial intelligence, machine learning, cloud computing, and distributed computing.
### 3.1 Artificial Intelligence and Machine Learning Tools
The new version of MATLAB integrates deep learning frameworks such as TensorFlow, PyTorch, and Keras, enabling MATLAB users to easily build and train deep learning models. Additionally, MATLAB has expanded its machine learning algorithm library, adding support for supervised learning, unsupervised learning, and reinforcement learning algorithms.
#### 3.1.1 Deep Learning Framework Integration
The integration of MATLAB with deep learning frameworks like TensorFlow, PyTorch, and Keras provides users with powerful tools to construct and train deep learning models. These frameworks offer pre-trained models, optimization algorithms, and visualization tools, enabling MATLAB users to rapidly develop and deploy deep learning applications.
```
% Using TensorFlow to build a simple convolutional neural network
layers = [
imageInputLayer([28, 28, 1])
convolution2dLayer(3, 32, 'Padding', 'same')
reluLayer
maxPooling2dLayer(2, 'Stride', 2)
convolution2dLayer(3, 64, 'Padding', 'same')
reluLayer
maxPooling2dLayer(2, 'Stride', 2)
fullyConnectedLayer(10)
softmaxLayer
classificationLayer
];
options = trainingOptions('sgdm', ...
'InitialLearnRate', 0.01, ...
'MaxEpochs', 10, ...
'MiniBatchSize', 128);
net = trainNetwork(data, labels, layers, options);
```
**Code Logic Analysis:**
* This code utilizes TensorFlow to build a convolutional neural network for classification tasks.
* `imageInputLayer` defines the size and number of channels of the input images.
* `convolution2dLayer` and `reluLayer` are used for feature extraction and nonlinear activation.
* `maxPooling2dLayer` reduces the size of the feature maps.
* `fullyConnectedLayer` and `softmaxLayer` are used for classification.
* `classificationLayer` specifies the loss function and evaluation metrics.
* `trainingOptions` defines training parameters such as learning rate, maximum iterations, and batch size.
#### 3.1.2 Machine Learning Algorithm Library Expansion
MATLAB has expanded its machine learning algorithm library, adding support for supervised learning, unsupervised learning, and reinforcement learning algorithms. These algorithms include Support Vector Machines, Decision Trees, Clustering, and Reinforcement Learning algorithms.
```
% Using MATLAB's Decision Tree algorithm to classify data
data = load('data.mat');
features = data.features;
labels = data.labels;
tree = fitctree(features, labels);
predictedLabels = predict(tree, features);
accuracy = sum(predictedLabels == labels) / length(labels);
```
**Code Logic Analysis:**
* This code uses MATLAB's Decision Tree algorithm to classify data.
* The `fitctree` function is used to train the Decision Tree model.
* The `predict` function is used to predict new data.
* The `accuracy` variable stores the classification accuracy.
### 3.2 Cloud Computing and Distributed Computing Support
The new version of MATLAB provides cloud platform connectors, making it easy for MATLAB users to deploy MATLAB applications to cloud platforms such as AWS, Azure, and Google Cloud. Additionally, MATLAB supports distributed computing frameworks such as MPI and Spark, enabling MATLAB users to execute MATLAB code in parallel on cluster environments.
#### 3.2.1 Cloud Platform Connectors
Connectors for cloud platforms such as AWS, Azure, and Google Cloud allow MATLAB users to easily deploy MATLAB applications to cloud platforms. These connectors provide integration with cloud platform services, such as storage, computing, and machine learning services.
```
% Using MATLAB's AWS connector to upload data to S3
import aws
s3 = aws.S3();
bucketName = 'my-bucket';
fileName = 'data.mat';
s3.putObject(bucketName, fileName, 'data.mat');
```
**Code Logic Analysis:**
* This code uses MATLAB's AWS connector to upload data to an S3 bucket.
* `import aws` imports the AWS connector.
* `s3 = aws.S3()` creates an S3 object.
* The `s3.putObject` function uploads the data to the specified bucket and file name.
#### 3.2.2 Distributed Computing Frameworks
MATLAB supports distributed computing frameworks such as MPI and Spark, allowing MATLAB users to execute MATLAB code in parallel on cluster environments. This greatly enhances the performance of processing large datasets and complex computational tasks.
```
% Using MATLAB's MPI parallel computing framework for matrix multiplication
import matlab.mpi
mpi = matlab.mpi.MPI();
A = randn(1000, 1000);
B = randn(1000, 1000);
% Distribute matrices to different processes
localA = mpi.scatter(A);
localB = mpi.scatter(B);
% Compute local matrix multiplication in each process
localC = localA * localB;
% Gather local results and aggregate
C = mpi.gather(localC);
```
**Code Logic Analysis:**
* This code uses MATLAB's MPI parallel computing framework for matrix multiplication.
* `import matlab.mpi` imports the MPI framework.
* `mpi = matlab.mpi.MPI()` creates an MPI object.
* The `mpi.scatter` function distributes matrices A and B across different processes.
* Each process computes local matrix multiplication.
* The `mpi.gather` function collects local results and aggregates them.
**4. Impact of Version Differences on Real-World Applications**
### 4.1 Scientific Computing and Engineering Simulation
MATLAB is widely used in scientific computing and engineering simulation. The performance improvements and functional enhancements brought by new versions have had a significant impact on these applications.
**4.1.1 Performance Improvement in Large Dataset Processing**
The new version of MATLAB has significantly improved large dataset processing performance through enhanced parallel computing capabilities and optimized memory management. Parallel computing leverages multicore processors to execute computational tasks simultaneously, drastically reducing the execution time for compute-intensive tasks such as large matrix solutions, image processing, and signal processing. Optimized memory management reduces memory consumption through more efficient memory allocation and release mechanisms, allowing MATLAB to handle larger datasets.
**4.1.2 Optimization of Complex Model Solving Efficiency**
MATLAB offers a rich set of solvers and toolboxes for solving complex models and equations. In the new version, solver algorithms have been optimized, improving solution efficiency and accuracy. For example, the fsolve solver for solving nonlinear equation systems has adopted a new hybrid algorithm, which is several times faster than previous versions when solving complex systems.
### 4.2 Data Analysis and Visualization
MATLAB has also seen significant enhancements in data analysis and visualization.
**4.2.1 Interactive Data Exploration Tools**
The new version of MATLAB has introduced interactive data exploration tools such as the Interactive Data Inspector and Data Apps, making data analysis more intuitive and efficient. These tools allow users to quickly browse and filter data, perform statistical analysis, and create interactive visualizations.
**4.2.2 Enhanced Visualization Effects**
The graphical and visualization capabilities of MATLAB have also been enhanced. The new version provides new types of plots, such as heat maps and scatter plot matrices, along with more powerful data visualization options. Additionally, 3D drawing performance has been optimized, enabling MATLAB to create more realistic 3D models and animations.
### 4.2.3 Real-World Application Examples
The following are real-world application examples that demonstrate the advantages of the new version of MATLAB in scientific computing and engineering simulation:
- **Large Dataset Processing:** An automotive manufacturer uses MATLAB to process vast amounts of sensor data to optimize vehicle performance. The enhanced parallel computing capabilities of the new version of MATLAB have reduced data processing time from hours to minutes, significantly improving efficiency.
- **Complex Model Solving:** An aerospace company uses MATLAB to solve complex fluid dynamics models to design more energy-efficient aircraft. The solver algorithm optimization in the new version of MATLAB has reduced model solving time by 50%, accelerating the design iteration process.
- **Interactive Data Exploration:** A biopharmaceutical company uses MATLAB to analyze clinical trial data. The interactive data exploration tools in the new version of MATLAB enable researchers to quickly identify patterns and anomalies in the data, thereby accelerating the drug development process.
**5. Version Selection and Upgrade Guide**
### 5.1 Criteria for Version Selection
When selecting a MATLAB version, consider the following factors:
- **Application Scenarios and Performance Requirements:** Different versions of MATLAB have performance and functional differences. For high-performance computing tasks such as large dataset processing and complex model solving, newer versions should be selected. For lighter tasks or scenarios with higher code compatibility requirements, lower versions may be chosen.
- **Compatibility of Existing Code:** If there is a substantial amount of MATLAB code, compatibility with the new version must be considered. Newer versions of MATLAB may include syntax or functional updates that could cause errors in existing code. Therefore, compatibility issues should be carefully evaluated before upgrading.
### 5.2 Precautions for Upgrading
When upgrading MATLAB versions, the following points should be noted:
- **License Update:** MATLAB is commercial software that requires a purchased license to use. When upgrading versions, the license may need to be updated.
- **Code Migration and Debugging:** Newer versions of MATLAB may include syntax or functional updates that could cause errors in existing code. Before upgrading, code should be migrated and debugged to ensure it operates correctly in the new version.
**Code Block:**
```
% Check MATLAB version
ver = ver('MATLAB');
% Select an appropriate upgrade strategy based on the version number
if strcmp(ver.Version, 'R2022b')
% Upgrade to R2023a
disp('Upgrade to R2023a to enjoy new features and performance improvements.');
elseif strcmp(ver.Version, 'R2023a')
% Already the latest version
disp('You are already using the latest version of MATLAB.');
else
% Upgrade to R2023a
disp('It is recommended to upgrade to R2023a for better performance and features.');
end
```
0
0