MATLAB Versions and Deep Learning: Model Development Training, Version Compatibility Guide
发布时间: 2024-09-14 01:44:59 阅读量: 13 订阅数: 18 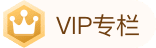
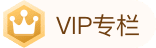
# 1. Introduction to MATLAB Deep Learning
MATLAB is a programming environment widely used for technical computation and data analysis. In recent years, MATLAB has become a popular platform for developing and training deep learning models. Its deep learning toolbox offers a wide range of functions and tools that allow developers to easily build, train, and deploy deep learning models.
This chapter will introduce the basics of deep learning in MATLAB. We will discuss the basic concepts of deep learning, an overview of the MATLAB deep learning toolbox, and the advantages of developing and training deep learning models in MATLAB.
# 2. Selecting MATLAB Versions for Deep Learning
### 2.1 The Impact of MATLAB Versions on the Deep Learning Toolbox
Different versions of MATLAB significantly affect the functionality and availability of the deep learning toolbox. Newer versions of MATLAB typically offer a broader set of features, better performance, and more stable APIs.
| MATLAB Version | Deep Learning Toolbox Version | Changes |
|---|---|---|
| R2017a | 10.0 | Initial release |
| R2018a | 11.0 | New pre-trained models and data augmentation features |
| R2018b | 11.1 | Improved training speed and model accuracy |
| R2019a | 12.0 | Introduction of TensorFlow integration and GPU acceleration |
| R2019b | 12.1 | Enhanced neural network visualization and debugging tools |
| R2020a | 13.0 | Support for PyTorch and ONNX models |
| R2020b | 13.1 | Optimized training performance and memory usage |
### 2.2 Functional Differences in Different MATLAB Versions
The main functional differences in different versions of MATLAB regarding deep learning include:
- **Pre-trained models:** Newer versions of MATLAB provide more pre-trained models, covering tasks such as image classification, object detection, and natural language processing.
- **Data augmentation:** Newer versions of MATLAB offer richer image and text data augmentation features to improve model generalization.
- **Training speed:** Newer versions of MATLAB have optimized training speed through parallel computing and GPU acceleration.
- **Model accuracy:** Newer versions of MATLAB have introduced new optimization algorithms and regularization techniques to improve model accuracy.
- **Visualization and debugging tools:** Newer versions of MATLAB offer more powerful neural network visualization and debugging tools, facilitating model development and troubleshooting.
### 2.3 Considerations for Version Selection
When selecting a MATLAB version, consider the following factors:
- **Deep learning tasks:** Different versions of MATLAB support different types of tasks. For example, R2020a and higher versions support PyTorch and ONNX models.
- **Model complexity:** More complex neural network models may require a newer version of MATLAB for better performance and stability.
- **Hardware resources:** If using GPU-accelerated training, choose a MATLAB version that supports GPUs.
- **Compatibility:** Ensure that the MATLAB version is compatible with other tools and libraries, such as TensorFlow and PyTorch.
- **Support:** Newer versions of MATLAB usually provide better technical support and documentation.
# 3. Developing Deep Learning Models in MATLAB
### 3.1 Data Preprocessing and Feature Engineering
Data preprocessing and feature engineering are crucial steps in the development of deep learning models. They help improve the model's performance and generalization ability.
**Data Preprocessing**
Data preprocessing involves the following tasks:
- **Data cleaning:** Remove missing values, outliers, and noise.
- **Data normalization:** Scale or normalize the data to a uniform range to impro
0
0
相关推荐
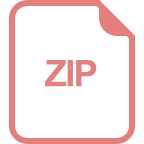
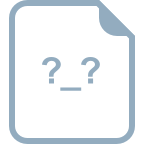






