【Lua数据结构与算法】:10大案例带你深入理解表操作和数据处理
发布时间: 2024-12-25 03:12:53 阅读量: 4 订阅数: 5 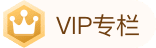
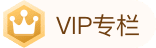
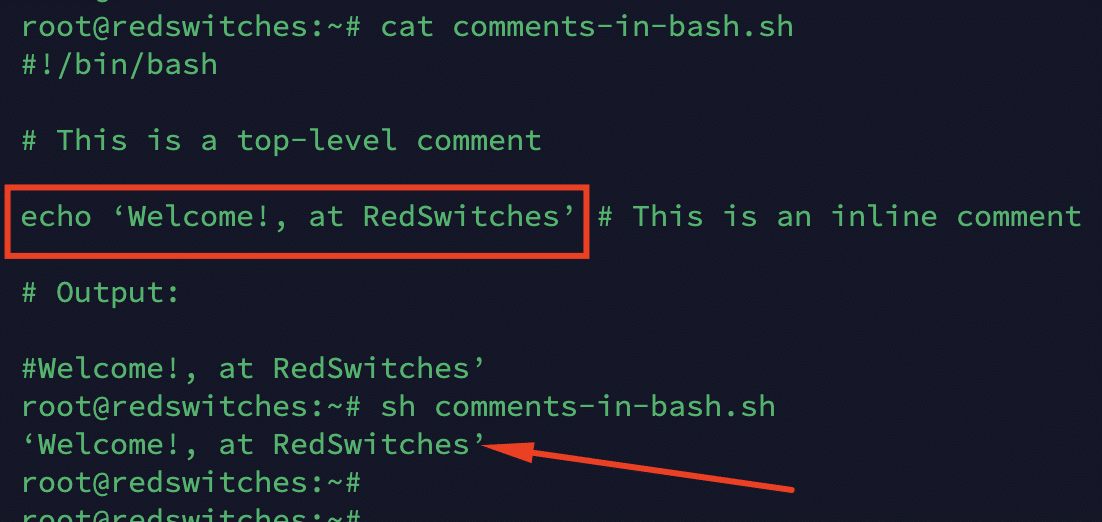
# 摘要
本文系统地探讨了Lua语言中的数据结构与算法基础。首先,深入解析了Lua中的表(table)这一核心数据结构,涵盖了其基础操作、高级特性和性能分析,强调了表在内存管理和优化方面的重要性。继而,文章着重于排序与搜索算法在Lua中的具体实现,包括常用排序算法和搜索算法的效率分析。本文还通过案例分析,详细讲解了链表、栈、队列和树结构在Lua中的应用,以及这些数据结构如何在实际开发中解决问题。最后,综合案例分析与实践部分通过哈希表和图结构的应用、遍历算法及其在算法案例实战中的应用,全面展示了Lua语言处理数据结构和算法的高效性。
# 关键字
Lua;数据结构;算法;表;排序;搜索;图结构;哈希表
参考资源链接:[Lua脚本语言:简单而强大](https://wenku.csdn.net/doc/646b4772543f844488c9e68d?spm=1055.2635.3001.10343)
# 1. Lua数据结构与算法基础
Lua语言是一种简洁、高效、灵活的脚本语言,非常适合用于嵌入应用程序中。Lua内置了丰富的数据结构和算法基础,使得开发者能够快速开发出高效的应用程序。在本章中,我们将探索Lua的核心数据结构,包括其基础的数组和哈希表特性,同时引入算法思维,为后续章节中对Lua高级数据结构的深入讨论打下坚实的基础。
Lua的数组和哈希表特性允许我们以非常高效的方式存储和操作数据。这些数据结构是所有高级数据结构与算法实现的基石。在本章中,我们将学习这些基础数据结构的操作,包括如何创建、初始化、插入和删除数据元素。同时,我们还将探讨这些操作的时间复杂度,帮助开发者更好地理解如何在Lua中进行性能优化。
在本章的后续部分,我们将介绍Lua中的常用算法,例如排序和搜索算法,并讨论这些算法在Lua中的实现。这些基础内容不仅是学习Lua数据结构与算法的起点,同时也是理解后续章节中更复杂数据结构和算法的关键。
通过本章的学习,读者将对Lua的基础数据结构和算法有一个全面的了解,并能够开始将这些知识应用于实际问题的解决中。
# 2. 深入理解Lua中的表
Lua中的表是一种非常强大的数据结构,它能够实现数组、字典、集合等多种数据类型的复杂操作。本章将深入探讨表的创建、操作和高级特性,并对表的性能进行分析。
## 2.1 表的基础操作
### 2.1.1 表的创建和初始化
Lua表的创建非常灵活,无需声明变量类型。创建表的基本语法如下:
```lua
local myTable = {}
```
可以使用多种方式初始化表:
```lua
-- 使用表构造器初始化
local t = {1, 2, 3, [5] = 5}
-- 使用预定义键值对初始化
local employee = {
["name"] = "John Doe",
["age"] = 30,
["position"] = "Developer"
}
-- 混合使用
local mixedTable = {
"one",
"two",
key3 = 3,
[4] = "four"
}
```
### 2.1.2 表的插入和删除操作
在Lua中,向表中插入元素非常简单:
```lua
local t = {1, 2, 3}
table.insert(t, 4) -- t = {1, 2, 3, 4}
```
如果要删除表中的某个元素,可以使用 `table.remove` 函数:
```lua
local t = {1, 2, 3, 4}
table.remove(t, 2) -- t = {1, 3, 4}
```
也可以通过赋值为 `nil` 来手动删除元素:
```lua
t[2] = nil -- t = {1, nil, 4}
```
## 2.2 表的高级特性
### 2.2.1 元表与元方法
元表允许我们改变表的行为,比如重载算术运算符。定义元方法的方式如下:
```lua
local a = {}
local b = {}
setmetatable(a, {__add = function(a, b) return b end}) -- a + b 将返回 b
local c = a + b -- c 现在等于 b
```
### 2.2.2 表的迭代器
迭代器允许我们以一种模式遍历表中的元素。以下是一个简单的迭代器例子:
```lua
local states = {"California", "Oregon", "Washington"}
for i,v in ipairs(states) do
print(v)
end
```
### 2.2.3 表与函数的高级结合
结合函数,表可以用来实现闭包等复杂的功能,例如:
```lua
function makeCounter()
local count = 0
return function()
count = count + 1
return count
end
end
counter = makeCounter()
print(counter()) -- 输出 1
print(counter()) -- 输出 2
```
## 2.3 表的性能分析
### 2.3.1 表操作的时间复杂度
Lua表的操作通常是 O(1) 的时间复杂度,但当涉及到遍历或者哈希冲突处理时,时间复杂度会有所不同。
### 2.3.2 表内存管理与优化
表的内存管理主要取决于其使用的频繁程度以及元素的类型。在处理大量数据时,需要特别注意内存占用情况。
```lua
-- 使用表的元方法控制内存使用
local t = setmetatable({}, {__mode = "k"}) -- 键为弱引用
t[1] = "one"
t[2] = "two"
-- 清除对键1和2的引用,它们将被垃圾回收
t[1] = nil
t[2] = nil
```
通过以上示例,我们可以看到Lua表的灵活性和强大的能力。在下一章,我们将深入探讨排序与搜索算法在Lua中的实现。
# 3. Lua中的排序与搜索算法
## 3.1 排序算法在Lua中的实现
### 3.1.1 冒泡排序、选择排序和插入排序
排序算法是计算机科学中的基础算法之一,用于将元素序列按照一定的顺序重新排列。在Lua中实现基本的排序算法,不仅可以帮助我们理解算法的逻辑,还能让我们熟悉Lua对数组操作的支持。
#### 冒泡排序
冒泡排序是最简单的排序算法之一,其工作原理是通过重复遍历要排序的数列,比较每对相邻元素的值,如果它们的顺序错误就把它们交换过来。遍历数列的工作是重复进行直到没有再需要交换的元素为止。
下面是冒泡排序的一个Lua实现示例:
```lua
function bubbleSort(arr)
local len = #arr
for i = 1, len do
for j = 1, len - i do
if arr[j] > arr[j + 1] then
arr[j], arr[j + 1] = arr[j + 1], arr[j]
end
end
end
end
local array = {64, 34, 25, 12, 22, 11, 90}
bubbleSort(array)
for i, v in ipairs(array) do
print(v)
end
```
在上面的示例中,我们通过双层循环实现了冒泡排序。内层循环负责比较和交换元素,外层循环负责控制排序的轮数。最终打印出排序后的数组。
#### 选择排序
选择排序算法是通过不断选择剩余元素中最小(或最大)的一个元素,然后放到未排序序列的起始位置,直到全部元素排完。
选择排序的Lua实现如下:
```lua
function selectionSort(arr)
local len = #arr
for i = 1, len do
local min_idx = i
for j = i+1, len do
if arr[j] < arr[min_idx] then
min_idx = j
end
end
arr[i], arr[min_idx] = arr[min_idx], arr[i]
end
end
local array = {64, 25, 12, 22, 11}
selectionSort(array)
for i, v in ipairs(array) do
print(v)
end
```
在选择排序中,我们通过两层循环选择最小的元素,并将其放到当前位置。通过这种方式,我们保证了每一次循环结束后,当前位置都是当前未排序部分的最小值。
#### 插入排序
插入排序的工作原理是通过构建有序序列,对于未排序数据,在已排序序列中从后向前扫描,找到相应位置并插入。
插入排序的Lua实现如下:
```lua
function insertionSort(arr)
for i = 2, #arr do
local key = arr[i]
local j = i - 1
while j >= 1 and arr[j] > key do
arr[j + 1] = arr[j]
j = j - 1
end
arr[j + 1] = key
end
end
local array = {12, 11, 13, 5, 6}
insertionSort(array)
for i, v in ipairs(array) do
print(v)
end
```
在这个例子中,我们从数组的第二个元素开始迭代,将每个元素插入到已排序的数组部分中。通过比较和移动已排序部分的元素,直到找到合适的插入位置,这样可以保证每次插入后,已排序部分仍然有序。
### 3.1.2 快速排序和归并排序
快速排序和归并排序是两种更高效的排序算法,它们利用了分治策略来提高排序的效率。
#### 快速排序
快速排序的基本思想是通过一趟排序将待排记录分隔成独立的两部分,其中一部分记录的关键字均比另一部分的关键字小,则可分别对这两部分记录继续进行排序,以达到整个序列有序。
快速排序的Lua实现如下:
```lua
function quickSort(arr)
if #arr <= 1 then
return arr
end
local pivot = table.remove(arr, #arr / 2)
local left, right = {}, {}
for _, v in ipairs(arr) do
if v < pivot then
table.insert(left, v)
else
table.insert(right, v)
end
end
return quickSort(left), {pivot}, quickSort(right)
end
local array = {10, 7, 8, 9, 1, 5}
local sortedArray = quickSort(array)
for i, v in ipairs(sortedArray) do
print(v)
end
```
#### 归并排序
归并排序是建立在归并操作上的一种有效的排序算法。该算法是采用分治法的一个非常典型的应用。将已有序的子序列合并,得到完全有序的序列;即先使每个子序列有序,再使子序列段间有序。
归并排序的Lua实现如下:
```lua
function mergeSort(arr)
if #arr <= 1 then
return arr
end
local mid = #arr / 2
local left, right = {}, {}
for i = 1, mid do
table.insert(left, arr[i])
end
for i = mid + 1, #arr do
table.insert(right, arr[i])
end
return merge(mergeSort(left), mergeSort(right))
end
function merge(left, right)
local result = {}
while #left > 0 and #right > 0 do
if left[1] < right[1] then
table.insert(result, table.remove(left, 1))
else
table.insert(result, table.remove(right, 1))
end
end
for i = 1, #left do
table.insert(result, table.remove(left, 1))
end
for i = 1, #right do
table.insert(result, table.remove(right, 1))
end
return result
end
local array = {38, 27, 43, 3, 9, 82, 10}
local sortedArray = mergeSort(array)
for i, v in ipairs(sortedArray) do
print(v)
end
```
在归并排序中,我们首先将数组分成两半,然后递归地对这两半进行归并排序。一旦两个子序列被排序,我们使用一个辅助函数来合并这两个子序列,并保持合并后的序列是有序的。
## 3.2 搜索算法在Lua中的实现
### 3.2.1 线性搜索和二分搜索
搜索算法用于在数据集合中查找特定的元素。线性搜索是最简单的搜索方法,而二分搜索则是一种更高效的搜索算法,适用于已排序的数据集。
#### 线性搜索
线性搜索是最基本的搜索算法,它通过逐一检查每个元素直到找到所需的特定项或者搜索完所有的元素。
线性搜索的Lua实现如下:
```lua
function linearSearch(arr, x)
for i, v in ipairs(arr) do
if v == x then
return i
end
end
return nil
end
local array = {2, 4, 6, 8, 10, 12}
local search_value = 10
local index = linearSearch(array, search_value)
if index then
print("Element found at index " .. index)
else
print("Element not found")
end
```
#### 二分搜索
二分搜索算法适用于有序数组,它通过不断将搜索区间缩小一半来加快搜索速度。每次将搜索区间的中间元素与目标值进行比较,从而确定搜索的上界或下界。
二分搜索的Lua实现如下:
```lua
function binarySearch(arr, x)
local left, right = 1, #arr
while left <= right do
local mid = math.floor((left + right) / 2)
if arr[mid] == x then
return mid
elseif arr[mid] < x then
left = mid + 1
else
right = mid - 1
end
end
return nil
end
local array = {1, 3, 5, 7, 9, 11}
local search_value = 5
local index = binarySearch(array, search_value)
if index then
print("Element found at index " .. index)
else
print("Element not found")
end
```
在二分搜索算法中,我们首先初始化搜索范围为整个数组。然后在循环中,我们不断计算中间索引,并根据中间元素与目标值的比较结果调整搜索范围的上下界。一旦找到目标值,算法返回其在数组中的位置;如果搜索范围被缩小到没有元素,说明目标值不存在于数组中。
### 3.2.2 搜索算法的效率分析
搜索算法的效率分析涉及时间复杂度的计算,主要根据算法的执行步骤数目与数据规模的关系来进行。
线性搜索的时间复杂度为O(n),因为它需要检查数组中的每一个元素。当数组很大时,其性能较差。
二分搜索的时间复杂度为O(log n),算法的执行速度随着数组大小的增长呈对数增长。这意味着即使在大型数组中,二分搜索也可以快速找到目标值。
通过本章节的介绍,读者应当能清晰地了解到排序与搜索算法在Lua中的实现方式,以及它们的时间复杂度,为使用Lua进行数据处理打下坚实的理论基础。在下一章节,我们将进一步深入讨论数据结构案例详解,其中包括链表、栈、队列以及树结构,并在Lua中探讨它们的实现和应用。
# 4. 数据结构案例详解
本章节将深入探讨几种常见的数据结构,并分析它们在Lua语言中的实现和应用。我们将从链表结构开始,讨论其单向与双向链表的特点和应用,然后转向栈和队列的基本实现及其应用领域。最后,我们将探讨树结构在Lua中的实现,包括二叉树及其变种以及树结构的遍历算法。
## 4.1 链表结构及其应用
链表是一种常见的线性数据结构,由一系列节点组成,每个节点包含数据部分和指向下一个节点的指针。在本节中,我们将介绍单向链表和双向链表的概念、实现和应用。
### 4.1.1 单向链表与双向链表
单向链表中的每个节点仅包含一个指向下一个节点的指针,而双向链表的每个节点还包含一个指向前一个节点的指针。这种结构使得双向链表在某些操作上(如反向遍历)更加高效。
#### 单向链表的实现
在Lua中,我们可以使用表来模拟节点,并用一个全局变量来追踪链表的头部。以下是一个简单的单向链表实现:
```lua
-- 定义节点结构
function create_node(data)
return {data = data, next = nil}
end
-- 向链表尾部添加节点
function append_node(head, data)
local new_node = create_node(data)
if head == nil then
return new_node
end
local current = head
while current.next do
current = current.next
end
current.next = new_node
return head
end
-- 打印链表
function print_list(head)
local current = head
while current do
io.write(current.data .. " -> ")
current = current.next
end
print("nil")
end
-- 创建链表和添加节点
local list = append_node(append_node(nil, 10), 20)
print_list(list) -- 输出: 10 -> 20 -> nil
```
#### 双向链表的实现
双向链表需要额外的指针来追踪前一个节点,实现上会稍微复杂一些:
```lua
-- 定义双向链表节点结构
function create_dnode(data)
return {data = data, prev = nil, next = nil}
end
-- 向双向链表尾部添加节点
function append_dnode(head, data)
local new_node = create_dnode(data)
if head == nil then
return new_node
end
local current = head
while current.next do
current = current.next
end
current.next = new_node
new_node.prev = current
return head
end
-- 打印双向链表
function print_dlist(head)
local current = head
while current do
io.write(current.data .. " <-> ")
current = current.next
end
print("nil")
end
-- 创建双向链表和添加节点
local dlist = append_dnode(append_dnode(nil, 10), 20)
print_dlist(dlist) -- 输出: 10 <-> 20 <-> nil
```
#### 单向链表与双向链表的比较
| 特性 | 单向链表 | 双向链表 |
|----------------|---------------------------------|---------------------------------|
| 内存占用 | 较少,每个节点只需要存储数据和一个指针 | 较多,每个节点需要额外存储一个指向前一个节点的指针 |
| 插入/删除操作 | 可以快速完成,只要改变相邻节点的指针 | 在链表中间插入或删除节点时效率更高,因为不需要改变后继节点的指针 |
| 访问元素 | 只能从前向后访问,访问效率低 | 可以前后访问,访问效率较高 |
### 4.1.2 链表在Lua中的实现和应用
链表在Lua中的应用广泛,尤其是在需要动态数据结构的场合。以下是一些链表的应用场景:
- **内存管理**:链表可用于实现内存池,通过链表来管理空闲内存块。
- **缓存机制**:实现LRU(最近最少使用)缓存时,链表能有效地维护数据的使用顺序。
- **事件驱动系统**:链表可以用于事件队列管理,按照事件发生的时间顺序进行处理。
## 4.2 栈和队列的应用
栈和队列是两种具有特殊访问规则的线性结构。栈遵循后进先出(LIFO)原则,而队列则遵循先进先出(FIFO)原则。
### 4.2.1 栈的实现与应用场景
栈是一种具有限制的线性表,仅允许在表的一端进行插入和删除操作。栈的这种后进先出特性使得它在许多场合非常有用。
#### 栈的实现
在Lua中实现栈相对简单:
```lua
function create_stack()
return {top = 0}
end
function push(stack, item)
stack.top = stack.top + 1
stack[stack.top] = item
end
function pop(stack)
local item = stack[stack.top]
stack[stack.top] = nil -- 清除元素
stack.top = stack.top - 1
return item
end
function peek(stack)
return stack[stack.top]
end
function is_empty(stack)
return stack.top == 0
end
-- 使用栈
local stack = create_stack()
push(stack, 1)
push(stack, 2)
print(pop(stack)) -- 输出: 2
```
#### 栈的应用场景
- **表达式求值**:在计算数学表达式时,栈可以用来处理运算符的优先级和括号。
- **浏览器历史记录**:后退按钮的功能可以用栈来实现,后访问的页面放在栈顶,点击后退则弹出栈顶页面。
- **递归算法**:在递归调用过程中,局部变量和返回地址都是通过栈来管理的。
### 4.2.2 队列的实现与应用场景
队列是一种先进先出(FIFO)的数据结构,它允许在一端进行插入操作,在另一端进行删除操作。
#### 队列的实现
在Lua中实现队列,我们可以使用表来模拟,同样使用一个变量来追踪队列的头部和尾部:
```lua
function create_queue()
return {front = 1, rear = 0}
end
function enqueue(queue, item)
queue.rear = queue.rear + 1
queue[queue.rear] = item
end
function dequeue(queue)
if is_empty(queue) then
return nil
end
local item = queue[queue.front]
queue[queue.front] = nil -- 移除元素
queue.front = queue.front + 1
return item
end
function is_empty(queue)
return queue.front > queue.rear
end
-- 使用队列
local queue = create_queue()
enqueue(queue, 1)
enqueue(queue, 2)
print(dequeue(queue)) -- 输出: 1
```
#### 队列的应用场景
- **任务调度**:操作系统中的进程调度可以使用队列来管理运行进程的顺序。
- **缓冲处理**:在I/O操作中,数据的接收和发送经常用队列进行缓存。
- **打印队列**:文档打印时,打印任务按提交顺序排入打印队列,依次打印。
## 4.3 树结构在Lua中的实现
树是一种分层的数据结构,具有根节点,每个节点可能有多个子节点,但只有一个父节点(除了根节点)。树结构常用于表示具有层次关系的数据,如组织架构、文件系统等。
### 4.3.1 二叉树及其变种
二叉树是每个节点最多有两个子节点的树,是树结构中应用最广泛的一种。在Lua中实现二叉树需要注意节点类的定义和树的插入、删除、遍历操作。
```lua
-- 定义二叉树节点结构
function create_btree_node(data)
return {data = data, left = nil, right = nil}
end
-- 向二叉树中插入节点
function insert_btree_node(root, data)
if root == nil then
return create_btree_node(data)
end
if data < root.data then
root.left = insert_btree_node(root.left, data)
else
root.right = insert_btree_node(root.right, data)
end
return root
end
```
二叉树有许多变种,包括但不限于:
- **二叉搜索树**(BST):对于树中的任意节点,其左子树中的所有元素都小于该节点,其右子树中的所有元素都大于该节点。
- **平衡二叉树**(AVL):任何节点的两个子树的高度最大差别为1,这使得AVL树在动态插入和删除操作时保持较好的平衡性。
- **红黑树**:一种自平衡的二叉搜索树,通过在节点中引入颜色信息和对树旋转来保持树的平衡。
### 4.3.2 树结构的遍历算法
树结构的遍历主要有三种方式:前序遍历、中序遍历和后序遍历。
#### 前序遍历
在前序遍历中,节点的处理发生在其子节点处理之前。遍历从根节点开始,递归遍历左子树,然后递归遍历右子树。
```lua
-- 前序遍历二叉树
function preorder_traversal(root)
if root ~= nil then
print(root.data) -- 访问节点
preorder_traversal(root.left) -- 遍历左子树
preorder_traversal(root.right) -- 遍历右子树
end
end
```
#### 中序遍历
在中序遍历中,节点的处理发生在其左子树处理之后,右子树处理之前。对于二叉搜索树,中序遍历可以得到一个有序的节点值序列。
```lua
-- 中序遍历二叉树
function inorder_traversal(root)
if root ~= nil then
inorder_traversal(root.left) -- 遍历左子树
print(root.data) -- 访问节点
inorder_traversal(root.right) -- 遍历右子树
end
end
```
#### 后序遍历
在后序遍历中,节点的处理发生在其子节点处理之后。这种遍历方式特别适合于删除树结构,因为可以确保在删除节点之前先删除其所有子节点。
```lua
-- 后序遍历二叉树
function postorder_traversal(root)
if root ~= nil then
postorder_traversal(root.left) -- 遍历左子树
postorder_traversal(root.right) -- 遍历右子树
print(root.data) -- 访问节点
end
end
```
树结构的遍历算法是各种树操作(如查找、插入、删除等)的基础。通过这些基本操作,树数据结构可以有效地支持复杂的数据管理和检索功能。
# 5. 综合案例分析与实践
## 5.1 哈希表的应用与实现
### 5.1.1 哈希表的基本原理
哈希表是一种使用哈希函数组织数据,以便于快速插入和查找数据的结构。它通过一个哈希函数将关键字映射到表中的一个位置,以加快搜索速度。哈希表的关键在于哈希函数的设计,它应该尽可能地减少哈希冲突,即不同的关键字映射到同一个表的位置。
```lua
--Lua中实现哈希表的一个简单示例
function hash(key, size)
return key % size
end
local hashTable = {}
local function put(key, value)
local index = hash(key, 10) -- 假设表的大小为10
hashTable[index] = value
end
local function get(key)
local index = hash(key, 10)
return hashTable[index]
end
```
### 5.1.2 哈希冲突的解决方法
当两个不同的关键字通过哈希函数映射到相同的表位置时,哈希冲突就发生了。解决哈希冲突的方法有很多,包括开放寻址法和链表法。链表法是最常用的解决方法,它将哈希到同一个位置的所有元素存储在链表中。
```lua
--链表法处理哈希冲突
local hashTable = {}
function put(key, value)
local index = hash(key, 10)
local bucket = hashTable[index]
if not bucket then
bucket = {}
hashTable[index] = bucket
end
-- 插入链表头部
table.insert(bucket, {key, value})
end
function get(key)
local index = hash(key, 10)
local bucket = hashTable[index]
if bucket then
for i, pair in ipairs(bucket) do
if pair[1] == key then
return pair[2]
end
end
end
return nil
end
```
## 5.2 图结构的遍历与应用
### 5.2.1 图的表示方法
图是一种复杂的数据结构,用于表示多对多的关系。图可以用邻接矩阵或邻接表表示。邻接矩阵适合表示稠密图,而邻接表适合表示稀疏图。
```lua
--使用邻接表表示图的Lua实现
local graph = {
['A'] = {'B', 'C'},
['B'] = {'A', 'D', 'E'},
['C'] = {'A', 'F'},
['D'] = {'B'},
['E'] = {'B', 'F'},
['F'] = {'C', 'E'}
}
```
### 5.2.2 图的深度优先搜索与广度优先搜索
深度优先搜索(DFS)和广度优先搜索(BFS)是两种图遍历的基本算法。DFS使用递归的方式,尽可能深地搜索图的分支;BFS则逐层进行遍历。
```lua
--深度优先搜索(DFS)
local visited = {}
function DFS(graph, node)
if not visited[node] then
visited[node] = true
print(node)
for _, neighbor in ipairs(graph[node]) do
DFS(graph, neighbor)
end
end
end
--广度优先搜索(BFS)
function BFS(graph, start)
local queue = {start}
visited[start] = true
while #queue > 0 do
local node = table.remove(queue, 1)
print(node)
for _, neighbor in ipairs(graph[node]) do
if not visited[neighbor] then
visited[neighbor] = true
table.insert(queue, neighbor)
end
end
end
end
DFS(graph, 'A')
visited = {} -- 重置访问状态
BFS(graph, 'A')
```
## 5.3 算法案例实战演练
### 5.3.1 最短路径问题求解
最短路径问题是指在一个图中找到两个顶点之间的最短路径。Dijkstra算法是最常用的算法之一,适用于带权重的图,其中权重非负。
```lua
--Dijkstra算法求最短路径
function dijkstra(graph, start)
local distances = {}
for node in pairs(graph) do
distances[node] = math.huge
end
distances[start] = 0
local visited = {}
while true do
-- 选择最小距离未访问的节点
local minDistance, currentNode = math.huge, nil
for node, distance in pairs(distances) do
if not visited[node] and distance < minDistance then
minDistance, currentNode = distance, node
end
end
if not currentNode then break end
visited[currentNode] = true
for _, neighbor in ipairs(graph[currentNode]) do
local distance = distances[currentNode] + 1 -- 假设权重为1
if distance < distances[neighbor] then
distances[neighbor] = distance
end
end
end
return distances
end
local shortestPaths = dijkstra(graph, 'A')
for node, distance in pairs(shortestPaths) do
print("The shortest path from A to " .. node .. " is " .. distance)
end
```
### 5.3.2 最小生成树问题求解
最小生成树问题是指在一个加权连通图中找到一个边的子集,这些边构成了一棵树,覆盖了图中的所有顶点,并且边的权重和最小。
```lua
--Kruskal算法求最小生成树
function find(parent, i)
if parent[i] == nil then
return i
end
return find(parent, parent[i])
end
function union(parent, rank, x, y)
local xset = find(parent, x)
local yset = find(parent, y)
if rank[xset] < rank[yset] then
parent[xset] = yset
else
parent[yset] = xset
if rank[xset] == rank[yset] then
rank[xset] = rank[xset] + 1
end
end
end
function kruskal(graph, nodes)
local mst = {}
local cost = 0
local i = 0
local e = 0
table.sort(graph, function(a, b) return a.weight < b.weight end)
while e < #nodes - 1 do
local edge = table.remove(graph, 1)
local x = find(parent, edge.node1)
local y = find(parent, edge.node2)
if x ~= y then
e = e + 1
union(parent, rank, x, y)
cost = cost + edge.weight
table.insert(mst, edge)
end
end
return mst, cost
end
-- 假设的边集合
local edges = {
{node1 = 'A', node2 = 'B', weight = 10},
{node1 = 'B', node2 = 'C', weight = 2},
{node1 = 'A', node2 = 'C', weight = 5},
-- ... 其他边
}
local parent = {}
local rank = {}
for _, node in ipairs(nodes) do
parent[node] = node
end
local mst, cost = kruskal(edges, nodes)
print("Minimum Spanning Tree: ", mst)
print("Total cost: ", cost)
```
以上章节涉及了数据结构综合案例分析与实践,从哈希表的基本原理和冲突解决,到图结构的遍历算法,再到算法案例实战演练中最小生成树和最短路径问题的解决方案,为读者提供了从理论到实践的深入理解和应用。
0
0
相关推荐
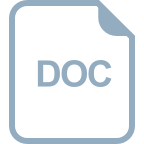
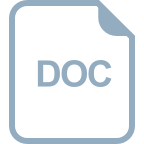
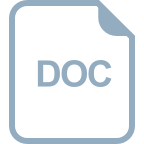
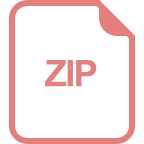
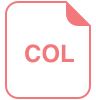
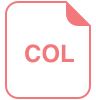
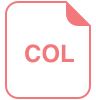
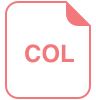
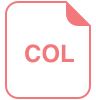