The task of monitoring malicious activities in large-scale networks has become increasingly challenging. The sheer volume and heterogeneity of network traffic hinder the manual definition of IDS signatures and deep packet inspection. In this thesis, the author presents MINOS, a novel, completely unsupervised method for generating anomaly scores for each host, enabling high-precision classification of infected (host generating malicious activity), attacked (host under attack), or clean (no infection) hosts. The hourly scores generated can detect the time range of host being infected or attacked without any prior knowledge. MINOS automatically creates personalized traffic behavior models for each host, without the need for any prior knowledge of existing or unknown attacks. Experimental evaluations on real large-scale academic networks data spanning over a year show that MINOS achieves very high accuracy even with just two weeks of data analysis. The author demonstrates that MINOS is also more effective and faster than state-of-the-art unsupervised anomaly detection methods for traffic data. The thesis "MINOS: Unsupervised Netflow-Based Detection of Infected and Attacked Hosts, and Attack Time in Large Networks" by Mousume Bhowmick, submitted for the degree of Master of Science in Computer Science at Boise State University in August 2019, presents a significant contribution to the field of network security, offering a promising approach to addressing the challenges of monitoring and detecting malicious activities in large-scale networks.
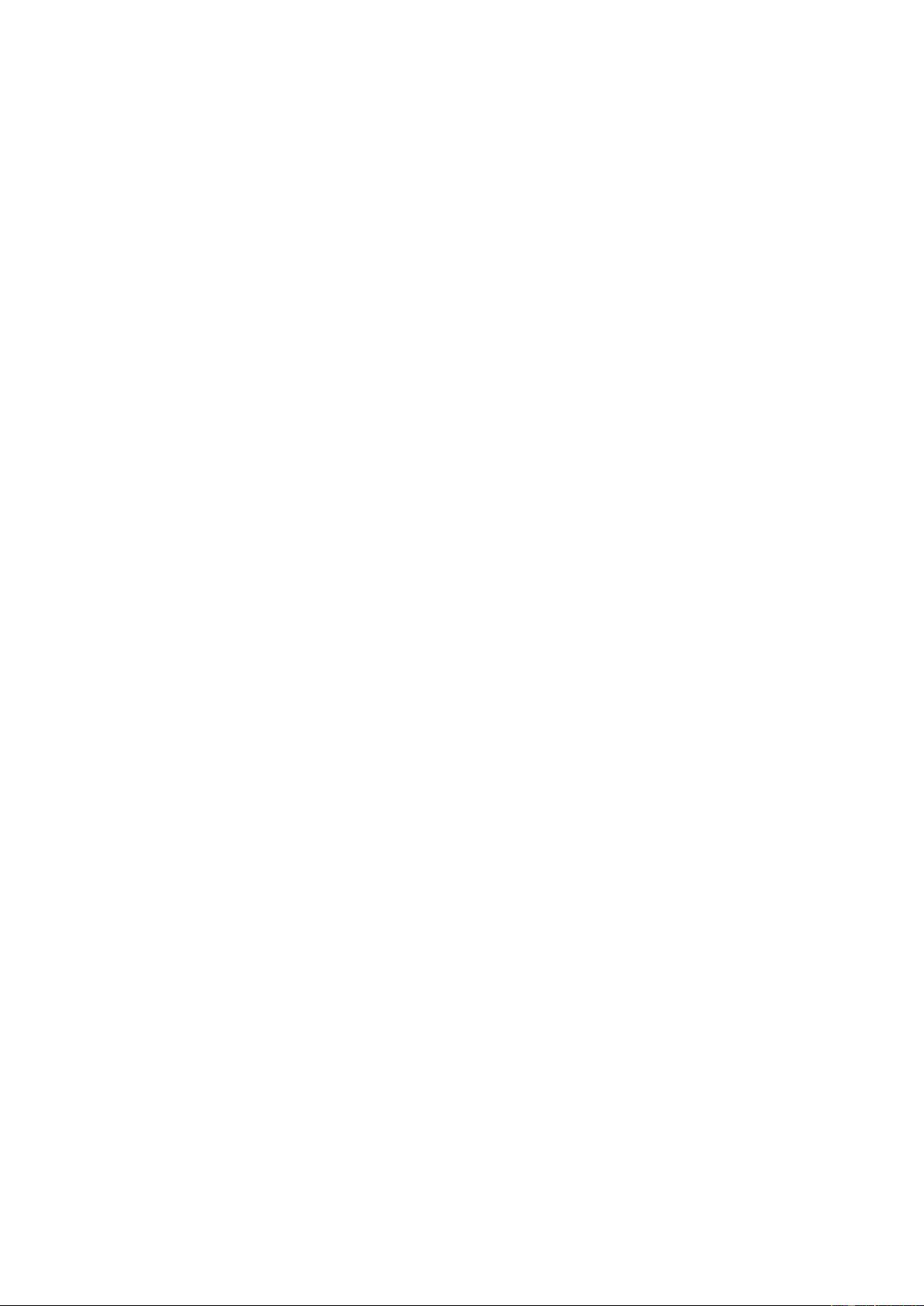
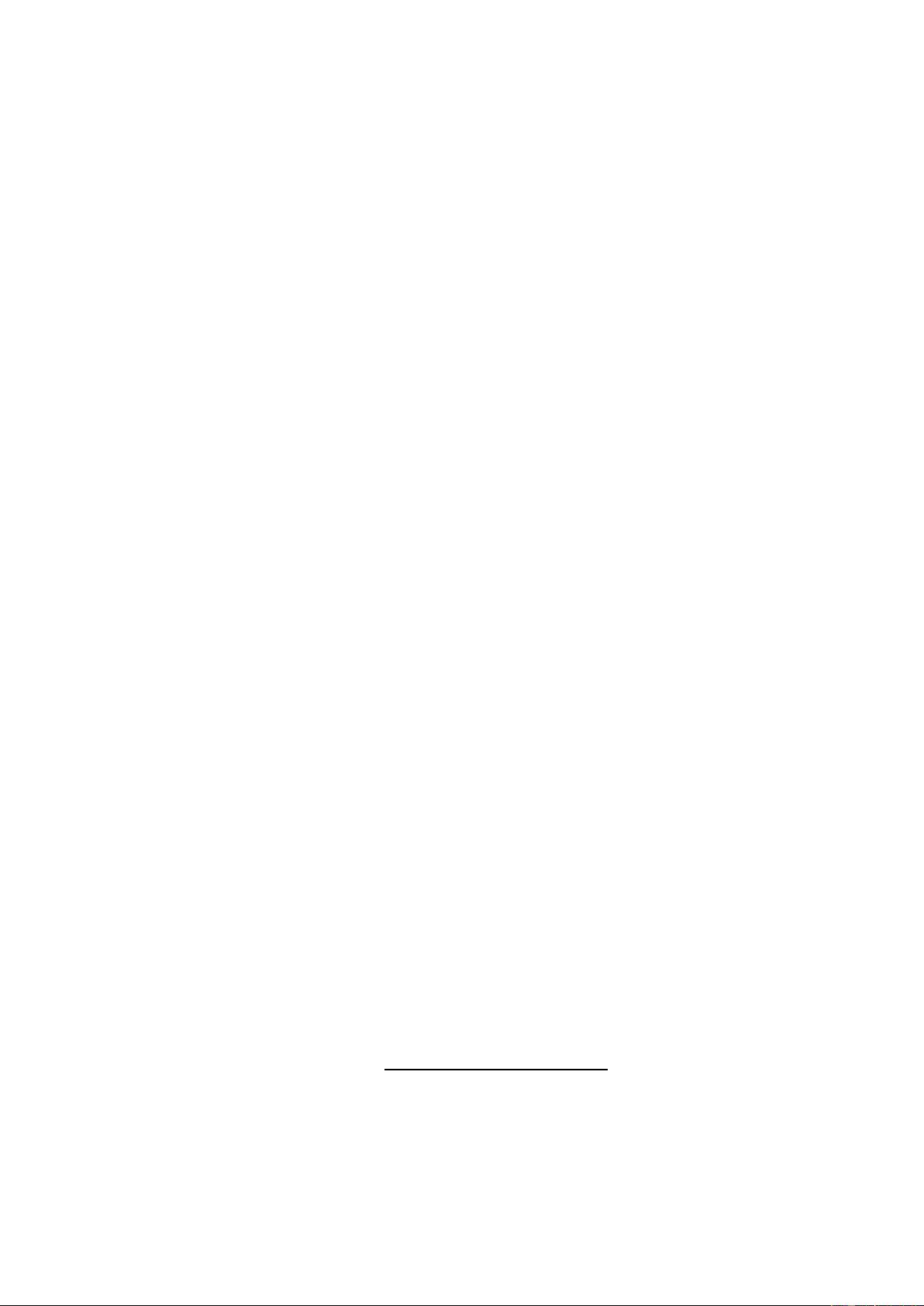
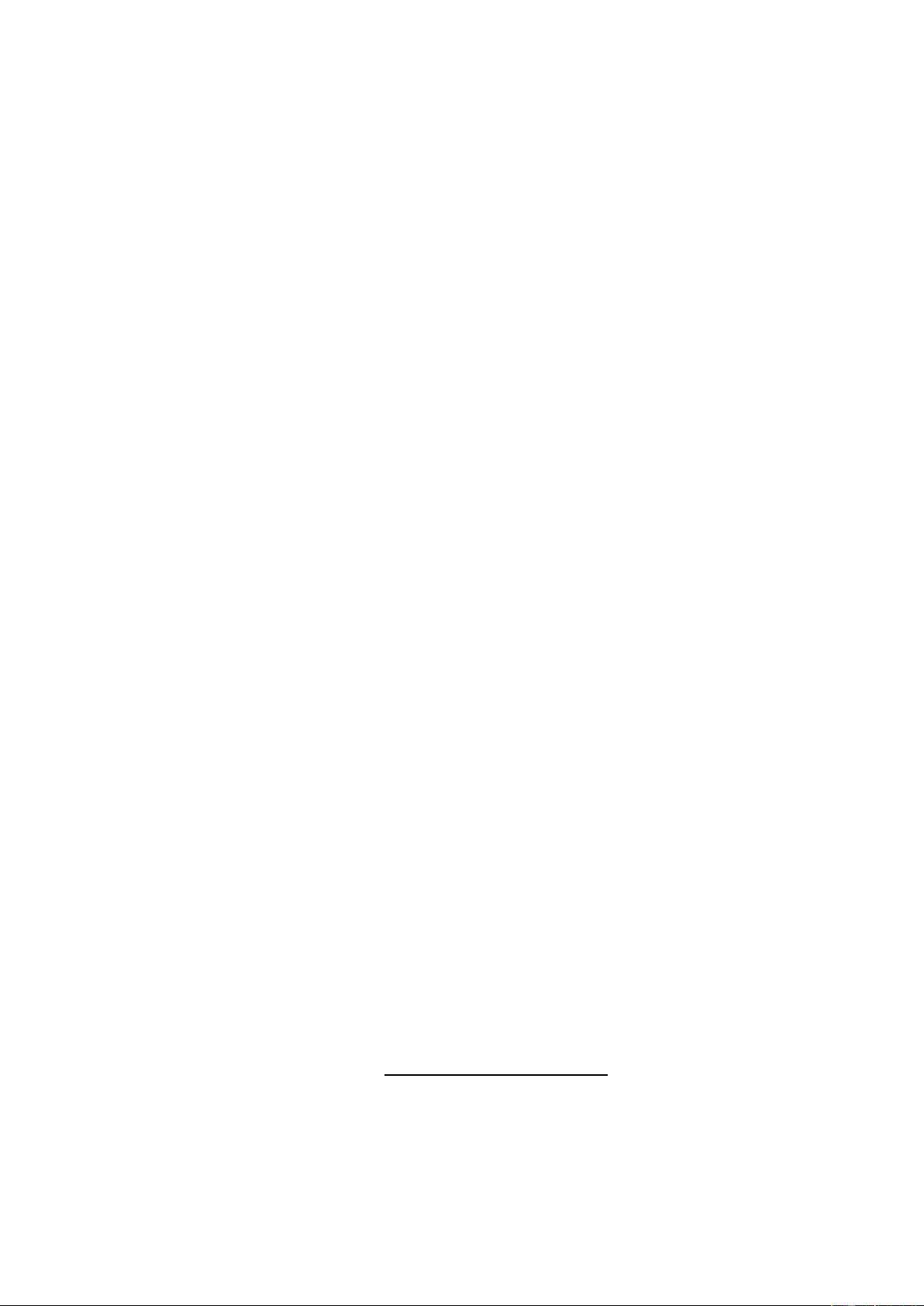
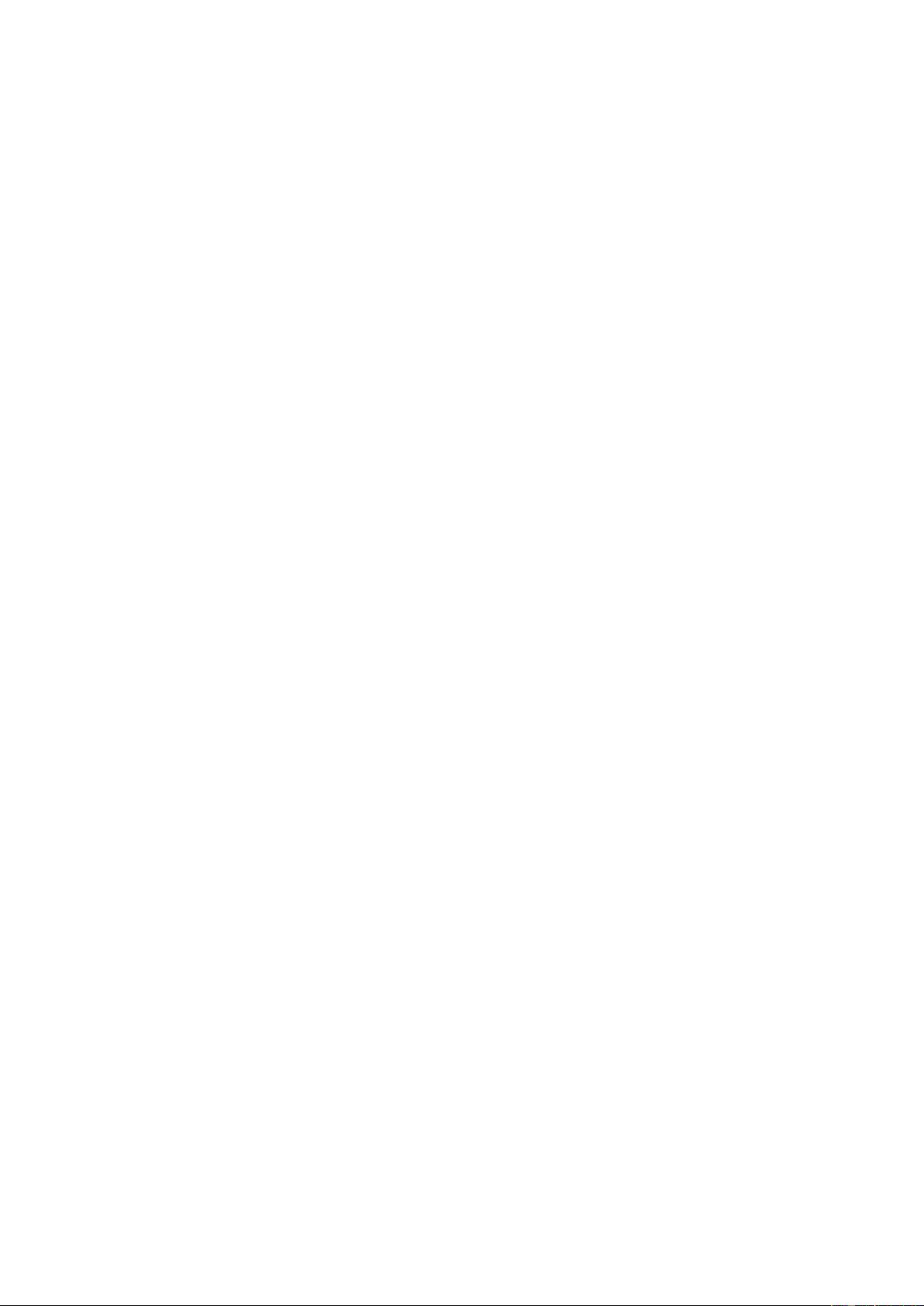
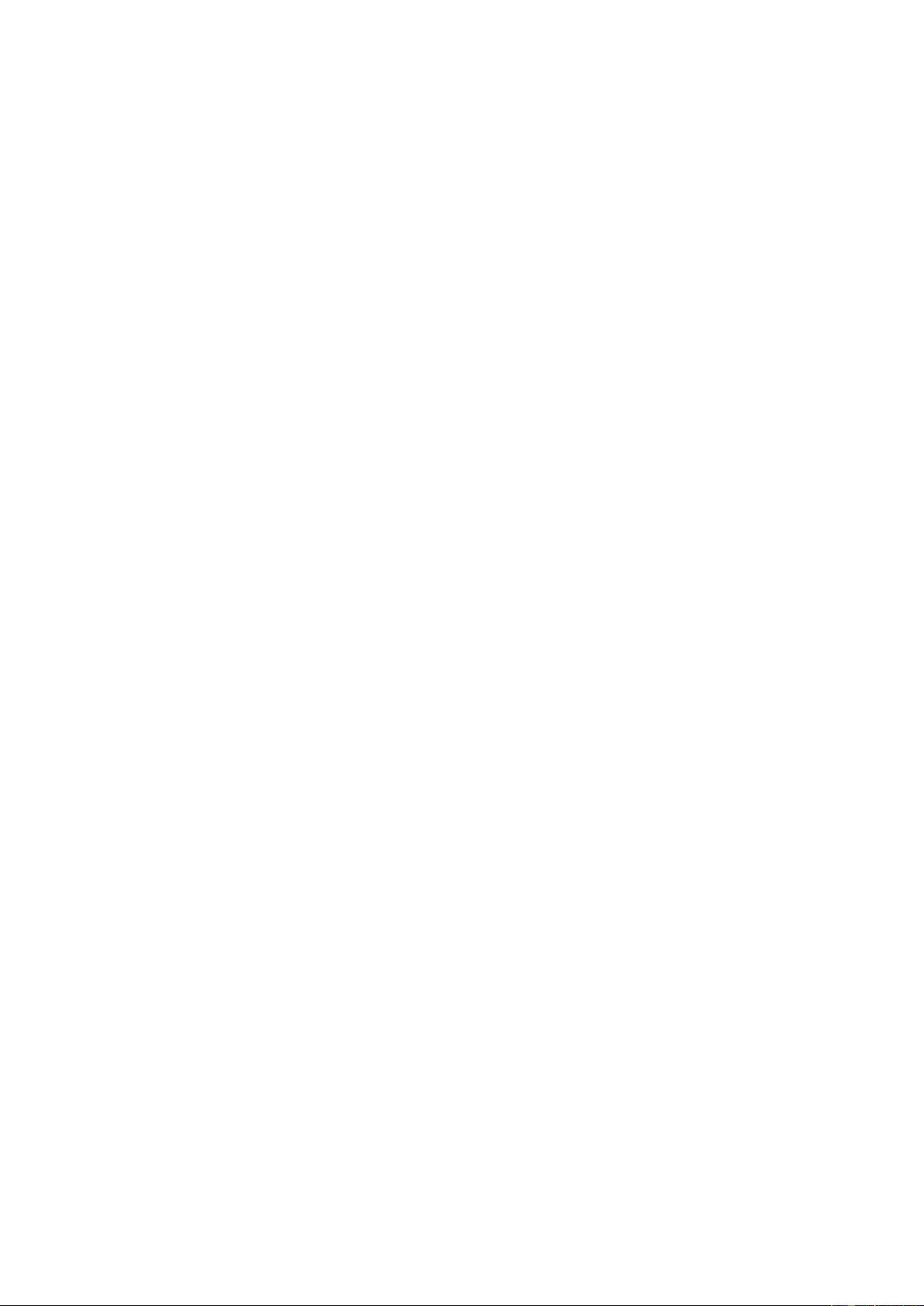
剩余99页未读,继续阅读
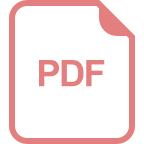
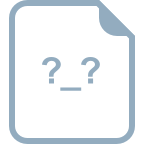







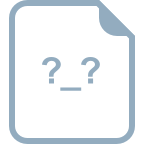
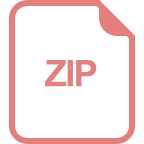
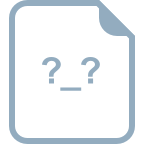

- 粉丝: 243
- 资源: 1940
我的内容管理 展开
我的资源 快来上传第一个资源
我的收益
登录查看自己的收益我的积分 登录查看自己的积分
我的C币 登录后查看C币余额
我的收藏
我的下载
下载帮助

最新资源
- 计算机人脸表情动画技术发展综述
- 关系数据库的关键字搜索技术综述:模型、架构与未来趋势
- 迭代自适应逆滤波在语音情感识别中的应用
- 概念知识树在旅游领域智能分析中的应用
- 构建is-a层次与OWL本体集成:理论与算法
- 基于语义元的相似度计算方法研究:改进与有效性验证
- 网格梯度多密度聚类算法:去噪与高效聚类
- 网格服务工作流动态调度算法PGSWA研究
- 突发事件连锁反应网络模型与应急预警分析
- BA网络上的病毒营销与网站推广仿真研究
- 离散HSMM故障预测模型:有效提升系统状态预测
- 煤矿安全评价:信息融合与可拓理论的应用
- 多维度Petri网工作流模型MD_WFN:统一建模与应用研究
- 面向过程追踪的知识安全描述方法
- 基于收益的软件过程资源调度优化策略
- 多核环境下基于数据流Java的Web服务器优化实现提升性能

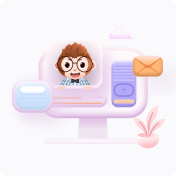
