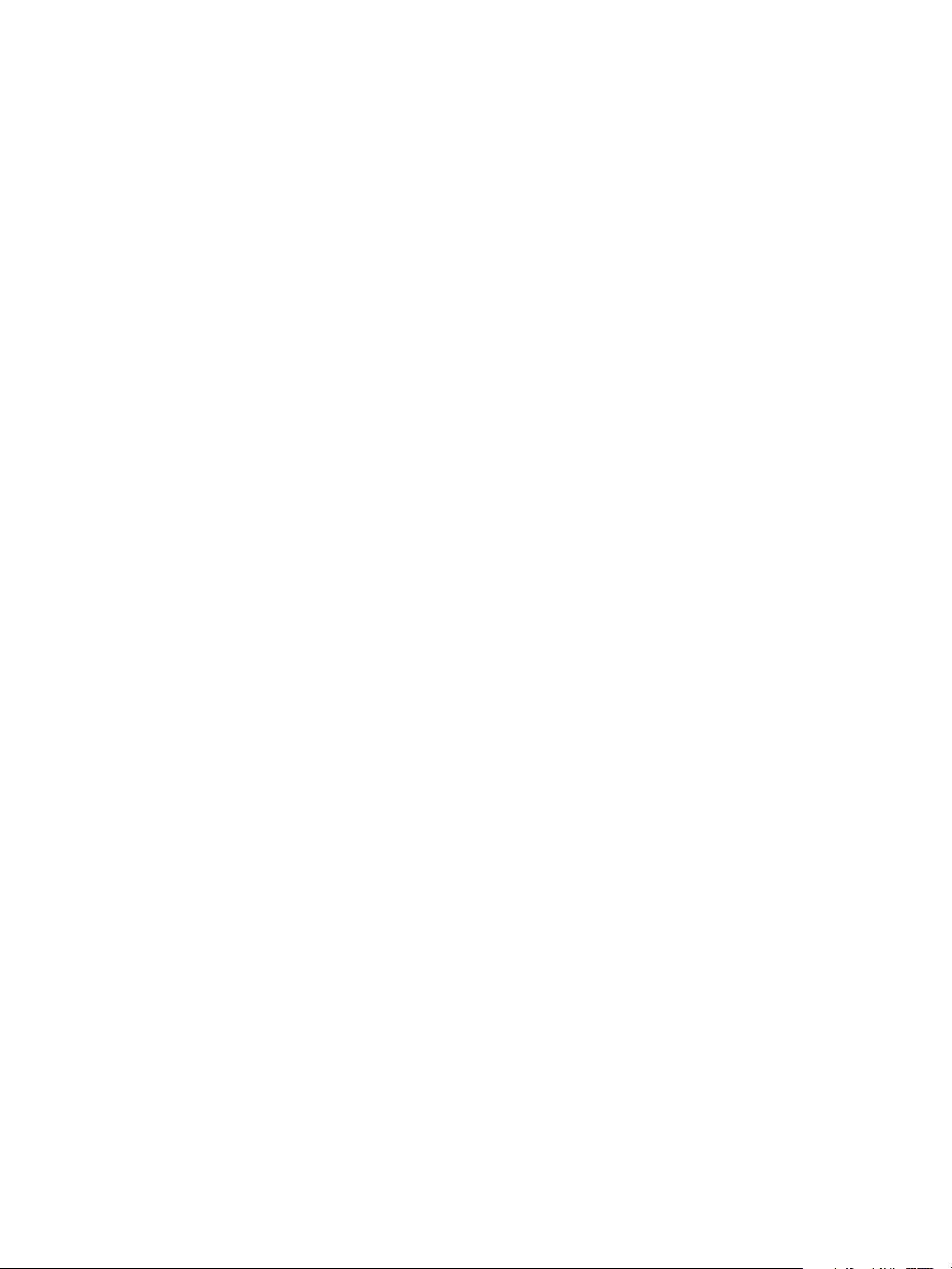
402 IEEE TRANSACTIONS ON CYBERNETICS, VOL. 43, NO. 2, APRIL 2013
Hidden Behavior Prediction of Complex Systems
Based on Hybrid Information
Zhi-Jie Zhou, Chang-Hua Hu, Bang-Cheng Zhang, Dong-Ling Xu, and Yu-Wang Chen
Abstract—It is important to predict both observable and hidden
behaviors in complex engineering systems. However, compared
with observable behavior, it is often difficult to establish a forecast-
ing model for hidden behavior. The existing methods for predicting
the hidden behavior cannot effectively and simultaneously use
the hybrid information with uncertainties that include qualitative
knowledge and quantitative data. Although belief rule base (BRB)
has been employed to predict the observable behavior using the
hybrid information with uncertainties, it is still not applicable
to predict the hidden behavior directly. As such, in this paper,
a new BRB-based model is proposed to predict the hidden be-
havior. In the proposed BRB-based model, the initial values of
parameters are usually given by experts, thus some of them may
not be accurate, which can lead to inaccurate prediction results.
In order to solve the problem, a parameter estimation algorithm
for training the parameters of the forecasting model is further
proposed on the basis of maximum likelihood algorithm. Using
the hybrid information with uncertainties, the proposed model can
combine together with the parameter estimation algorithm and
improve the forecasting precision in an integrated and effective
manner. A case study is conducted to demonstrate the capability
and potential applications of the proposed forecasting model with
the parameter estimation algorithm.
Index Terms—Belief rule base (BRB), hidden behavior, hybrid
information, Maximum likelihood (ML), parameter estimation
algorithm, prediction, uncertainty.
I. INTRODUCTION
I
T IS very important to predict system behaviors. For exam-
ple, in some systems such as nuclear power stations and
rocket control loops that have high requirements for safety,
it is necessary to predict the system behaviors that may lead
to major faults [38]. In engineering practice, the system be-
haviors can be divided into two types: the first type is the
Manuscript received October 20, 2011; revised March 2, 2012 and
June 17, 2012; accepted June 29, 2012. Date of publication August 14, 2012;
date of current version April 16, 2013. This work was supported by the National
Science Foundation of China under Grant 61004069, the National Natural
Science Funds for Distinguished Young Scholar under grant 61025014, the
Natural Science Foundation of Shaanxi Province under Grant 2011JQ8007, the
Foundation of Department of Education of JiLin Province of China under Grant
2009109, and the U.K. Engineering and Physical Science Research Council
under Grant No.: EP/F024606/1. This paper was recommended by Associate
Editor Y. Xiang.
Z.-J. Zhou and C.-H. Hu are with High-Tech Institute of Xi’an, Xi’an
710025, China (e-mail: zhouzj04@mails.tsinghua.edu.cn; hch6603@263.net).
B.-C. Zhang is with the School of Mechatronic Engineering Changchun
University of Technology, Changchun 130012, China (e-mail: zhang-
bangcheng@mail.ccut.edu.cn).
D.-L. Xu and Y.-W. Chen are with the Manchester Business School, Univer-
sity of Manchester, M15 6PB Manchester, U.K. (e-mail: Ling. Xu@mbs. ac.uk;
Chen@mbs.ac.uk).
Color versions of one or more of the figures in this paper are available online
at http://ieeexplore.ieee.org.
Digital Object Identifier 10.1109/TSMCB.2012.2208266
behavior that can be observed directly, and the second type
is that is hidden and can be observed indirectly. For example,
gyro is an important device in the inertial platform system,
and drift is the main factor of affecting the performance of
gyro. However, when the inertial platform works, the gyro
drift is not directly observable and can only be assessed via
other measured condition data such as voltage or electricity.
Compared with observable behavior, hidden behavior is often
difficult to identify, and its forecasting model is also difficult to
establish [20]. This paper focuses on addressing the latter type.
In order to predict the hidden behavior, two types of meth-
ods that include analytical model-based methods and data-
driven-based methods have been proposed. The analytical
model-based methods usually involve two steps: first, a state
or parameter is chosen to reflect the hidden behavior if the
mathematical model of a system is known. Then, according to
the characteristics of the system and noises, the corresponding
filter-based predictors including Kalman predictor [31], strong
tracking predictor [3], fuzzy Kalman predictor [35], and particle
predictor [4] are chosen to predict the state or parameter.
The data-driven-based methods include qualitative
knowledge-based method and hybrid information-based
method (i.e., method based on qualitative knowledge and
quantitative data). If some qualitative knowledge about a
system is known, the qualitative knowledge-based method,
such as expert system-based method [1] and Petri net-based
method [30], can be used to infer the hidden behavior of the
system. If the hybrid information i s available, a number of
theoretical methods have been employed to use the hybrid
information for predicting the hidden behavior, such as hidden
Markov model (HMM) [2], [8], [12], [15], [ 16], [32]–[34],
[37], dynamic Bayesian network (DBN) [9], [13], [17],
[19], [22], domain knowledge and statistical model-based
method [10], qualitative knowledge and linear partial least
square-based method [11], and fuzzy logic and artificial neural
network (FL-ANN)-based method [18], [25]. The main steps
of the hybrid information-based method are: the qualitative
knowledge is first used to establish the initial forecasting
model, and then the quantitative data are employed to train the
forecasting model so that the future behaviors can be predicted
accurately.
Although the above forecasting methods have been applied in
some engineering problems, there are some shortcomings [14],
[38]. Model-based methods mainly use quantitative data and
are not applicable in the cases where the accurate mathematical
or statistical model of a complex system is difficult to ob-
tain. Data-driven methods can use either qualitative knowledge
or quantitative data or both to predict the hidden behavior.
2168-2267/$31.00 © 2012 IEEE