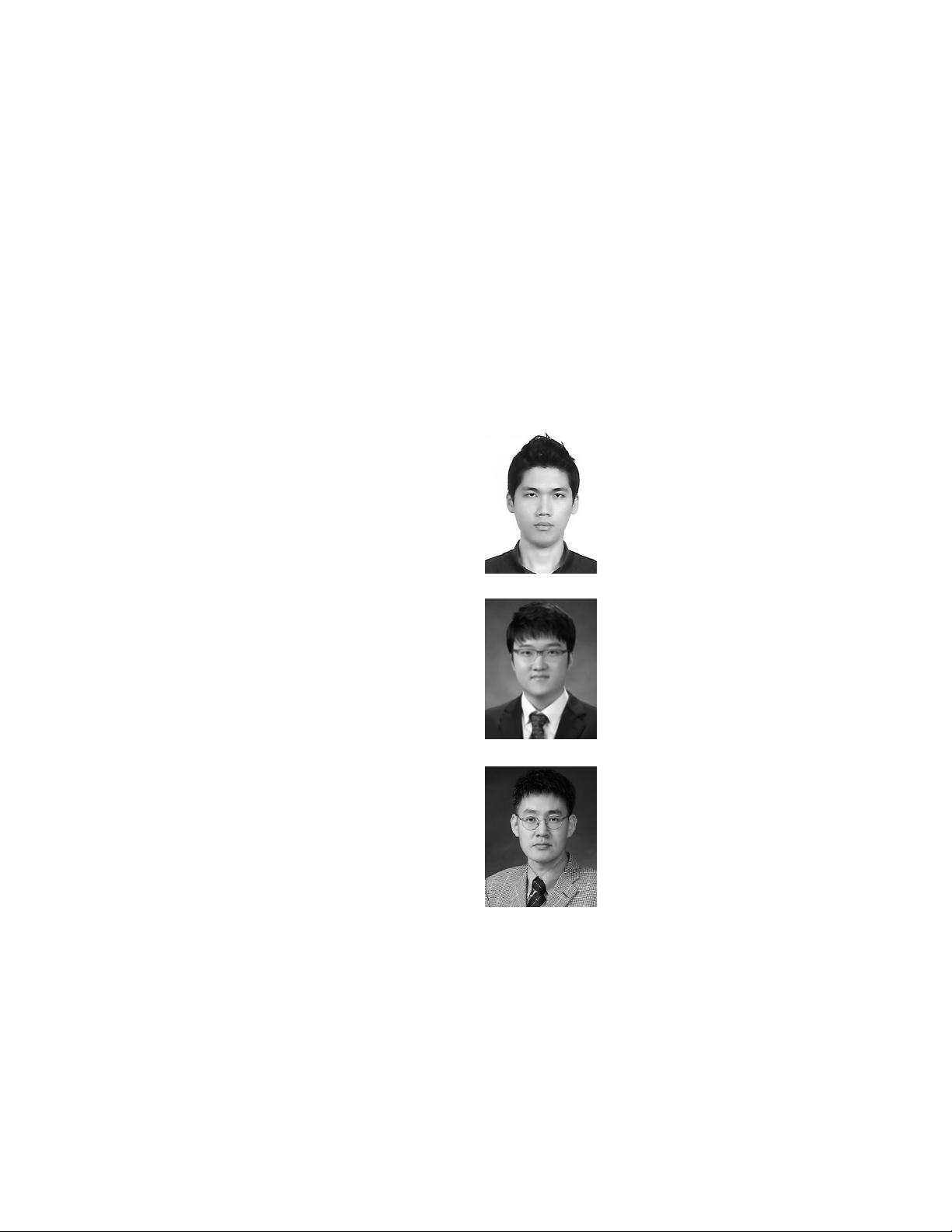
RHEE et al.: 0.3 lx–1.4 Mlx MONOLITHIC SILICON NANOWIRE LDC WITH TEMPERATURE-INDEPENDENT OFFSET CANCELLATION 391
[8] S. Sengupta, H. Ouh, and M. L. Johnston, “An all-digital CMOS ambient
light sensor using a single photon avalanche diode,” in Proc. IEEE
Sensors, Oct./Nov. 2017, pp. 1–3.
[9] H. Ouh and M. L. Johnston, “Dual-mode, in-pixel linear and single-
photon avalanche diode readout for low-light dynamic range extension
in photodetector arrays,” in Proc. IEEE Custom Integr. Circuits Conf.
(CICC), Apr. 2018, pp. 1–4.
[10] B. Shen, S. Bose, and M. L. Johnston, “A 1.2 V–20 V closed-loop charge
pump for high dynamic range photodetector array biasing,” IEEE Trans.
Circuits Syst., II, Exp. Briefs, vol. 66, no. 3, pp. 327–331, Mar. 2018.
[11] C. Soci, A. Zhang, X.-Y. Bao, H. Kim, Y. Lo, and D. Wang, “Nanowire
photodetectors,” J . Nanosci. Nanotechnol., vol. 10, no. 3, pp. 1430–1449,
Mar. 2010.
[12] J.-S. Yoon, K. Kim, M. Meyyappan, and C.-K. Baek, “Optical charac-
teristics of silicon-based asymmetric vertical nanowire photodetectors,”
IEEE Trans. Electron Devices, vol. 64, no. 5, pp. 2261–2266, May 2017.
[13] K.-N. Lee et al., “Fabrication of silicon nanowire for biosensor appli-
cations,” in Proc. IEEE Sensors, Oct. 2006, pp. 1269–1271.
[14] A. Zhang, Y. Liu, and Y. H. Lo, “Characterization and physics of
top-down silicon nanowire phototransistors,” Proc. SPIE, vol. 7608,
Art. no. 76018D1, Jan. 2010.
[15] K.-N. Lee, S.-W. Jung, K.-S. Shin, W.-H. Kim, M.-H. Lee, and
W.-K. Seong, “Fabrication of suspended silicon nanowire arrays,” Small,
vol. 4, no. 5, pp. 642–648, May 2008.
[16] J. Shin, K. Pi, S. Jung, and D.-I. D. Cho, “Silicon-nanowire field-effect
transistors on a flexible substrate using top-down MEMS processes,” in
Proc. MEMS, Jan. 2016, pp. 469–472.
[17] A. Zhang, H. Kim, J. Cheng, and Y. H. Lo, “Ultrahigh responsivity
visible and infrared detection using silicon nanowire phototransistors,”
Nano Lett., vol. 10, no. 6, pp. 2117–2120, Jun. 2010.
[18] M. N. Khiarak, S. Martel, Y. D. Koninck, and B. Gosselin, “A high-
sensitivity CMOS biophotometry sensor with embedded continuous-
time modulation,” in Pr oc. IEEE Int. Symp. Cir cuits Syst. (ISCAS),
May 2017, pp. 1–4.
[19] R. Yun and V. M. Joyner, “A monolithically integrated phase-sensitive
optical sensor for frequency-domain NIR spectroscopy,” IEEE Sensors
J., vol. 10, no. 7, pp. 1234–1242, Jul. 2010.
[20] W. Ahmad, M. Abdulaziz, M. Törmänen, and H. Sjöland, “CMOS
adaptive TIA with embedded single-ended to differential conversion for
analog optical links,” in Proc. IEEE Int. Symp. Circuits Syst. (ISCAS),
May 2015, pp. 658–661.
[21] M. N. Khiarak et al., “A wireless fiber photometry system based
on a high-precision CMOS biosensor with embedded continuous-time
modulation,” IEEE Trans. Biomed. Circuits Syst., vol. 12, no. 3,
pp. 495–509, Jun. 2018.
[22] M. N. Khiarak et al., “An energy-efficient CMOS biophotometry sensor
with incremental DT- ADC conversion,” in Proc. IEEE Int. Symp.
Circuits Syst. (ISCAS), May 2018, pp. 1–4.
[23] M. Lemkin and B. Boser, “A three-axis micromachined accelerometer
with a CMOS position-sense interface and digital offset-trim electron-
ics,” IEEE J. Solid-State Circuits, vol. 34, no. 4, pp. 456–468, Apr. 1999.
[24] R. Hogervorst, J. P. Tero, R. G. H. Eschauzier, and J. H. Huijsing,
“A compact power-efficient 3 V CMOS rail-to-rail input/output opera-
tional amplifier for VLSI cell libraries,” IEEE J. Solid-State Circ uits,
vol. 29, no. 12, pp. 1505–1513, Dec. 1994.
[25] R. Wu, K. A. A. Makinwa, and J. H. Huisjing, “A chopper current-
feedback instrumentation amplifier with a 1 mHz 1/f noise corner and an
AC-coupled ripple reduction loop,” IEEE J. Solid-State Circuits, vol. 44,
no. 12, pp. 3232–3243, Dec. 2009.
[26] M. A. T. Sanduleanu, A. J. M. Van Tuijl, R. F. Wassenaar,
M. C. Lammers, and H. Wallinga, “A low noise, low residual offset,
chopped amplifier for mixed level applications,” in Proc. IEEE Int. Conf.
Electron., Circuits Syst. Surfing Waves Sci. Technol., vol. 2, Sep. 1998,
pp. 333–336.
[27] R. Wu, Y. Chae, J. H. Huijsing, and K. A. A. Makinwa, “A 20-b
± 40-mV range read-out IC with 50-nV offset and 0.04% gain error
for bridge transducers,” IEEE J. Solid-State Circuits, vol. 47, no. 9,
pp. 2152–2163, Sep. 2012.
[28] J. Markus, J. Silva, and G. C. Temes, “Theory and applications of
incremental converters,” IEEE Trans. Circuits Syst. I, Reg. Papers,
vol. 51, no. 4, pp. 678–690, Apr. 2004.
[29] J. Jun, C. Rhee, S. Kim, and S. Kim, “An SC interface with
programmable-gain embedded ADC for monolithic three-axis 3-D
stacked capacitive MEMS accelerometer,” IEEE Sensors J., vol. 17,
no. 17, pp. 5558–5568, Sep. 2017.
[30] J. Silva, U. Moon, J. Steensgaard, and G. C. Temes, “Wideband low-
distortion delta-sigma ADC topology,” Electron. Lett., vol. 37, no. 12,
pp. 737–738, Jun. 2001.
[31] C. C. Enz and G. C. Temes, “Circuit techniques for reducing the effects
of op-amp imperfections: Autozeroing, correlated double sampling, and
chopper stabilization,” Proc. IEEE, vol. 84, no. 11, pp. 1584–1614,
Nov. 1996.
[32] B. E. A. Saleh and M. C. Teich, Fundamentals of Photonics, 2nd ed.
Hoboken, NJ, USA: Wiley, 2007.
[33] C.-T. Chiang, “Design of CMOS monolithic digitized light transducer
with calibration technique for ambient light sensor applications,” IEEE
Sensors J., vol. 13, no. 5, pp. 1931–1940, May 2013.
[34] F. Tang et al., “A linear 126-dB dynamic range light-to-frequency
converter with dark current suppression upto 125
◦
C for blood oxygen
concentration detection,” IEEE Trans. Electron Devices, vol. 63, no. 10,
pp. 3983–3988, Oct. 2016.
[35] C.-T. Chiang and J.-Y. Liou, “Design of a CMOS intelligent light sensing
chip for automatic brightness tuning applications,” IEEE Sensors J.,
vol. 13, no. 12, pp. 4955–4961, Dec. 2013.
[36] C.-T. Chiang, “Design of a CMOS monolithic digitized light detector
with noise insensitivity for light monitoring applications,” IEEE Sensors
J., vol. 14, no. 8, pp. 2537–2545, Aug. 2014.
Cyuyeol Rhee received the B.S. degree in electrical
engineering from the University of Toronto, Toronto,
ON, Canada, in 2010, and the Ph.D. degree in elec-
trical engineering from Seoul National University,
Seoul, South Korea, in 2019.
He is currently a Post-Doctoral Researcher with
Seoul National University. His research interests
include sensor readout circuits for various sensors
and data converters.
Junyoung Park (S’18) received the B.S. degree in
electrical engineering and computer science from
Seoul National University, Seoul, South Korea,
in 2015, where he is currently pursuing the Ph.D.
degree.
His research interests include data convert-
ers and sensor interfaces, including low-power,
high-resolution systems.
Suhwan Kim (S’97–M’01–SM’07) received the
B.S. and M.S. degrees in electrical engineering
and computer science from Korea University, Seoul,
South Korea, in 1990 and 1992, respectively, and the
Ph.D. degree in electrical engineering and computer
science from the University of Michigan, Ann Arbor,
MI, USA, in 2001.
From 1993 to 1999, he was with LG Elec-
tronics, Seoul, South Korea. From 2001 to 2004,
he was a Research Staff Member with IBM Thomas
J. Watson Research Center, Yorktown Heights, NY,
USA. In 2004, he joined Seoul National University, Seoul, where he is
currently a Professor of electrical and computer engineering. His research
interests include analog and mixed-signal integrated circuits, high-speed I/O
circuits, and silicon-photonic integrated circuits.
Dr. Kim was a recipient of the 1991 Best Student Paper Award of the IEEE
Korea Section, the First Prize (Operational Category) in the VLSI Design Con-
test of the 2001 ACM/IEEE Design Automation Conference, the Best Paper
Award of the 2009 Korean conference on semiconductors, and the 2011 Best
Paper Award of the International Symposium on Low-Power Electronics and
Design. He has served as the Organizing Committee Chair of the IEEE Asian
Solid-State Circuits Conference and the General Co-Chair and the Technical
Program Chair of the IEEE International System-on-Chip (SoC) Conference.
He has participated multiple times on the Technical Program Committee
of the IEEE International SOC Conference, the International Symposium
on Low-Power Electronics and Design, the IEEE Asian Solid-State Circuits
Conference, and the IEEE International Solid-State Circuits Conference.
He has served as a Guest Editor for the IEEE J
OURNAL OF SOLID-STATE
CIRCUITS special issue on the IEEE Asian Solid-State Circuits Conference.