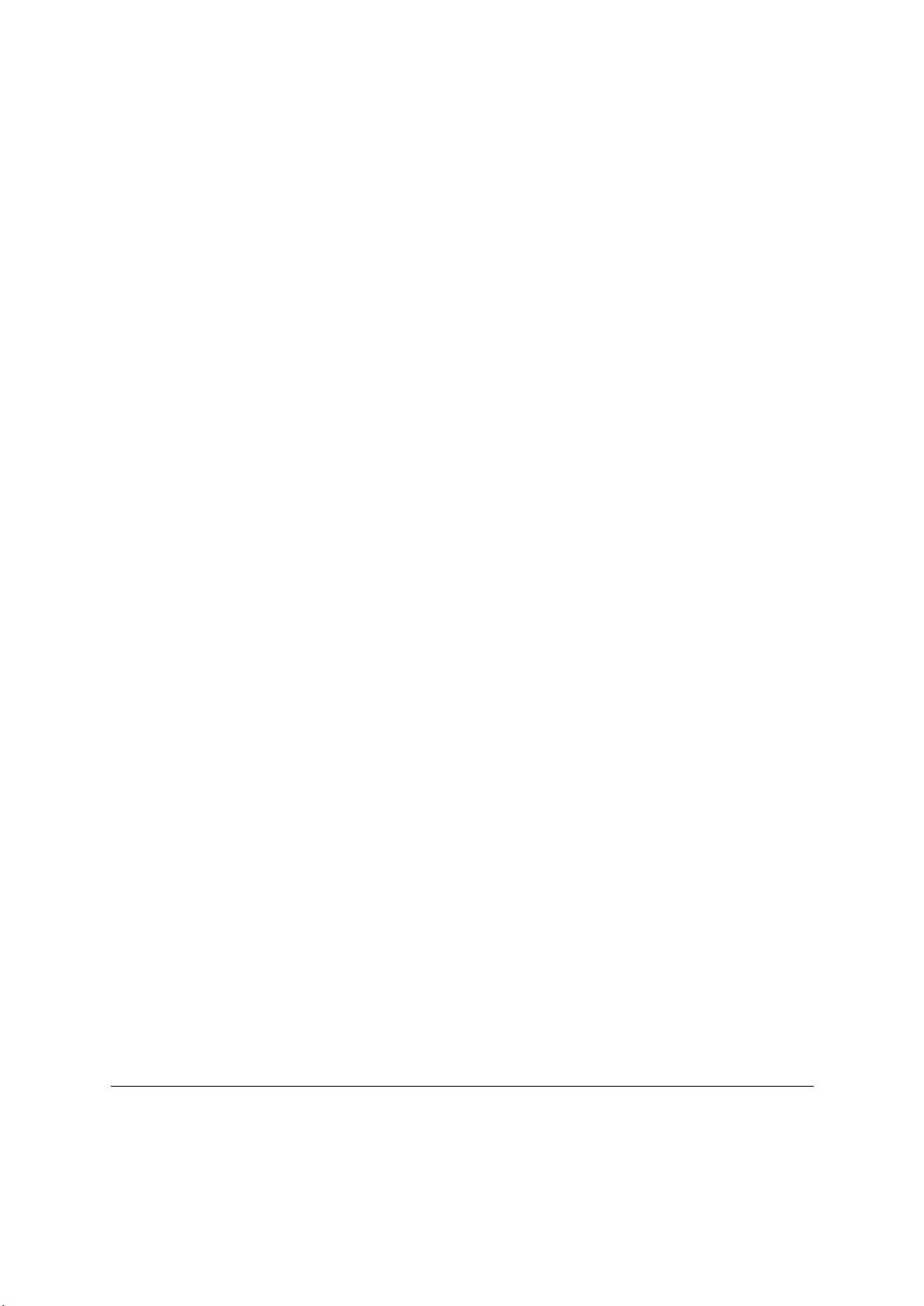
An ensemble feature selection technique for
cancer recognition
Jiucheng Xu
∗
, Lin Sun, Yunpeng Gao and Tianhe Xu
College of Computer and Information Engineering, Henan Normal University, Xinxiang, China
Engineering Technology Research Center for Computing Intelligence and Data Mining, Henan
Province, China
Abstract. Correlation-based feature selection (CFS) using neighborhood mutual information (NMI) and particle swarm opti-
mization (PSO) are combined into an ensemble technique in this paper. Based on this observation, an efficient gene selection
algorithm, denoted by NMICFS-PSO, is proposed. Several cancer recognition tasks are gathered for testing the proposed tech-
nique. Moreover, support vector machine (SVM), integrated with leave-one-out cross-validation and served as a classifier, is
employed for six classification profiles to calculate the classification accuracy. Experimental results show that the proposed
method can reduce the redundant features effectively and achieve superior performance. The classification accuracy obtained
by our method is higher in five out of the six gene expression problems as compared with that of other classification methods.
Keywords: Feature selection, neighborhood mutual information, particle swarm optimization, support vector machine
1. Introduction
The goal of microarray data classification is to build an efficient and effective model that can differen-
tiate the gene expressions of samples. The challenges posed in cancer recognition, available training data
sets are generally of a fairly small sample size compared to the number of genes involved, along with
experimental variations in measured gene expression levels. In general, only a relatively small number
of gene expression data show a strong correlation with a certain phenotype compared to the whole in-
vestigated. Thus, in order to analyze the gene expression profile correctly, feature (gene) selection is a
crucial step in gene array-based cancer recognition. Recently, many gene expression analysis and gene
selection techniques have been introduced. Sharma et al. [1] proposed a top-r feature selection method
to select optimal feature subsets and improve feature selection. Shon et al. [2] constructed a feature se-
lection method using a technique which combined filter method with wavelet transform to improve the
classification performance. Hu et al. [3] presented the basic concepts on neighborhood rough set model
and designed a novel forward feature selection method to select a minimal reduct, which avoided the
preprocess of data discretization and hence decreased the information lost in pretreatment. But the reduc-
t which satisfies criterions of higher classification performance and fewer gene numbers is not unique
*
Corresponding author. E-mail: jiuchengxu@gmail.com.
0959-2989/14/$27.50 © 2014 – IOS Press and the authors. All rights reserved
DOI 10.3233/BME-130897
IOS Press
Bio-Medical Materials and Engineering 24 (2014) 1001–1008 1001