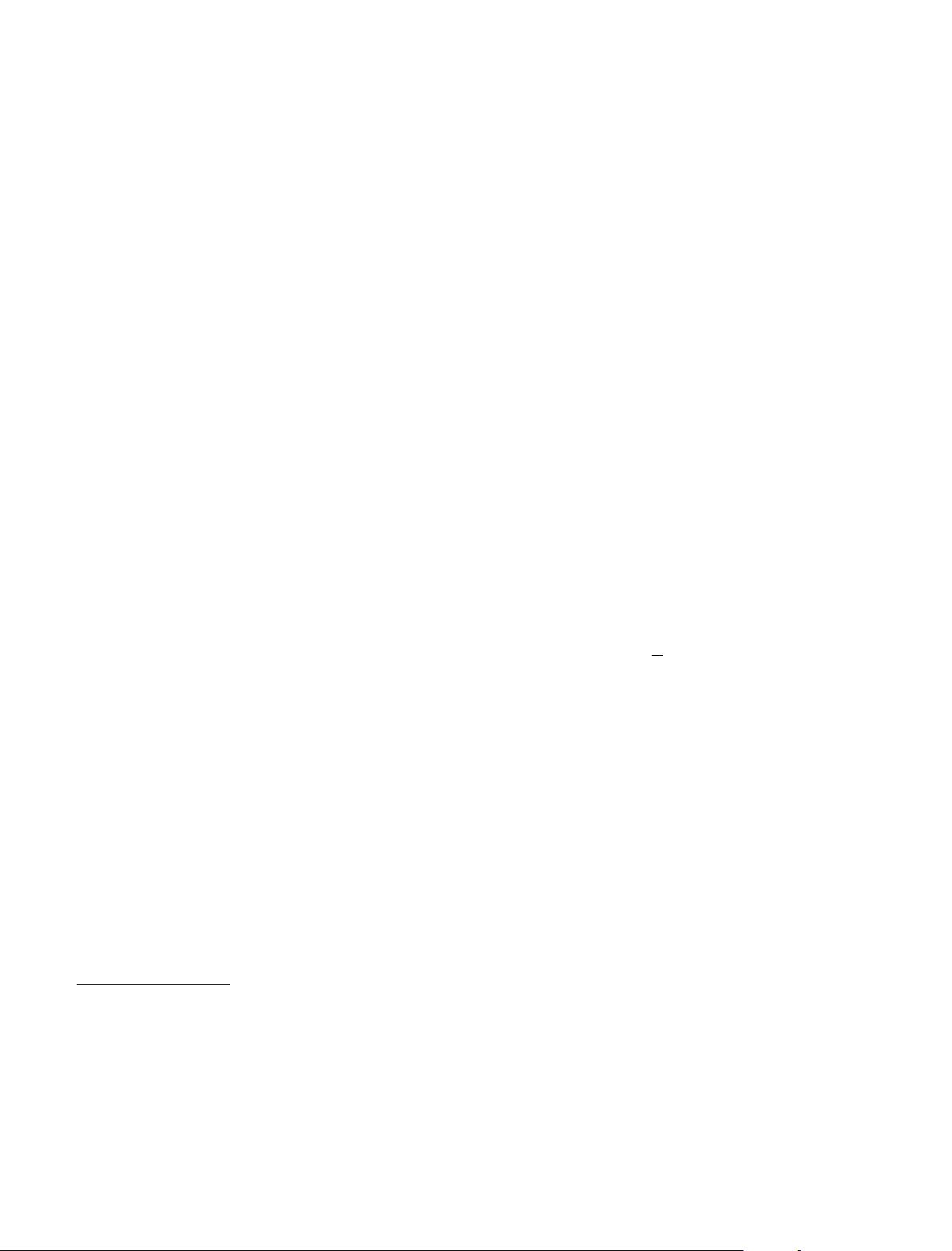
ADAPTIVE CONTROL DESIGN FOR A CLASS OF UNCERTAIN
HIGH-ORDER NONLINEAR SYSTEMS WITH TIME DELAY
Zong-Yao Sun and Yun-Gang Liu
ABSTRACT
This paper is concerned with adaptive stabilization for a class of uncertain high-order nonlinear systems with time delays.
To the authors’ knowledge, there has been no analogous result. Hence during investigation, the conditions on delay effect and the
control design framework should be established for the first time. In this paper, under somewhat necessary restrictions on the
system nonlinearities, by the method of adding a power integrator and the related adaptive technique, a procedure is developed
to design the continuous adaptive state-feedback controller without overparametrization. Moreover, the uniform stability and
convergence of the resulting closed-loop system are rigorously proven, with the aid of a suitable Lyapunov-Krasovskii functional.
Finally, a numerical example is provided to illustrate the effectiveness of the theoretical result.
Key Words: High-order time-delay nonlinear systems, adding a power integrator, adaptive stabilization, Lyapunov-Krasovskii
functional.
I. INTRODUCTION
Time delay systems have received considerable atten-
tion continuously over the past decades, since time delay
phenomena exist in many physical systems, such as mechani-
cal, biological and economical systems, and are often the
primary cause of instability and poor performance. So far,
great progress has been made for time delay linear systems
[1–3]. Nevertheless for time delay nonlinear systems, there
are many rather challenging control problems that remain
unsolved and need further investigation.
This paper considers adaptive stabilization for the high-
order time delay uncertain nonlinear system
x t x t f t xt xt dt
xt u t ft
i
i
p
i
n
p
n
i
n
() () (, (), ( ), ()),
() () (
=+ −
=+
+1
τ
,, ( ), ( ) ( )),xt xt dt−
⎧
⎨
⎩
τ
(1)
where i = 1,...,n −1,x(t) = [x
1
(t),...,x
n
(t)]
T
∈ R
n
is the
system state vector and u(t) ∈ R is the control input; d:R→R
r
is an unknown vector denoting the uncertainties or
unknowns; τ ≥ 0 denotes the time delay of the system state;
the system initial time is t
0
and the system initial condition is
x(θ) = ξ
0
(θ), θ ∈ [t
0
− τ, t
0
], with ξ
0
being a specified
continuous function; for i = 1,...,n ,
p
i
∈
≥
odd
1
is called the
powers of the system where
odd
≥1
denotes the set of all of the
numbers in the form of
p
q
with p and q being positive odd
integers and p ≥ q; and f
i
, named by system nonlinearity, is an
unknown continuous function and satisfies f
i
(t,0,0,d(t)) = 0.
When p
i
= 1, System (1) is known as the strict-feedback
nonlinear system, which has been extensively studied since
the early 1990s [4–6]. When p
i
> 1, the most remarkable
feature of the system is that its Jacobian linearization at the
origin is neither controllable nor observable, and this makes
the traditional design tools, such as feedback linearization [6]
or the backstepping method [5], difficult to apply to System
(1). Mainly due to the addition of a power integrator method,
a series of research results have been achieved over the past
decade for the case of p
i
> 1, see [7–12] and [13–14] for
stabilization and tracking, respectively.
When τ > 0, for System (1), which is actually retarded
functional differential equations (FDEs), we have solved two
special cases with weak uncertainties [15,16]. Specifically,
[15] attempted to construct a continuous controller by impos-
ing a strong condition on nonlinearities f
i
’s, and [16] relaxed
the restriction on f
i
’s in [15] and studied the case of multiple
state time delays. To the best of our knowledge, no adaptive
control results on such a class of systems with serious uncer-
tainties have ever been reported. Nevertheless there are plen-
tiful research results for the special case of p
i
= 1, for example
[17–22], where backstepping is the main method to control
design. Specifically, merged with a wavelet neural network,
[17–19] constructed neural network controllers for serval
Manuscript received March 15, 2013; revised July 18, 2013; accepted September
19, 2013.
Zong-Yao Sun (corresponding author, e-mail: sunzongyao@sohu.com) is with
institute of Automation, Qufu Normal University, Qufu, Shandong, 273165, China.
Yun-Gang Liu is with School of Control Science and Engineering, Shandong
University, Jinan, Shandong, 250061, China (e-mail: lygfr@sdu.edu.cn).
This work was supported by National Natural Science Foundation of China
(61004013, 61203013, 61273084, 61233014), the Shandong Provincial Natural
Science Foundation of China (ZR2010FQ003), the Independent Innovation Founda-
tion of Shandong University (2012JC014), the Specialized Research Fund for the
Doctoral Program of Higher Education (2011370 5120003), the Project of Taishan
Scholar of Shandong Province and the Doctoral Scientific Research Start-Up Foun-
dation of Qufu Normal University.
© 2014 Chinese Automatic Control Society and Wiley Publishing Asia Pty Ltd
Asian Journal of Control, Vol. 17, No. 2, pp. –
, March 2015
Published online 8 May 2014 in Wiley Online Library (wileyonlinelibrary.com) DOI: 10.1002/asjc.895
535
543