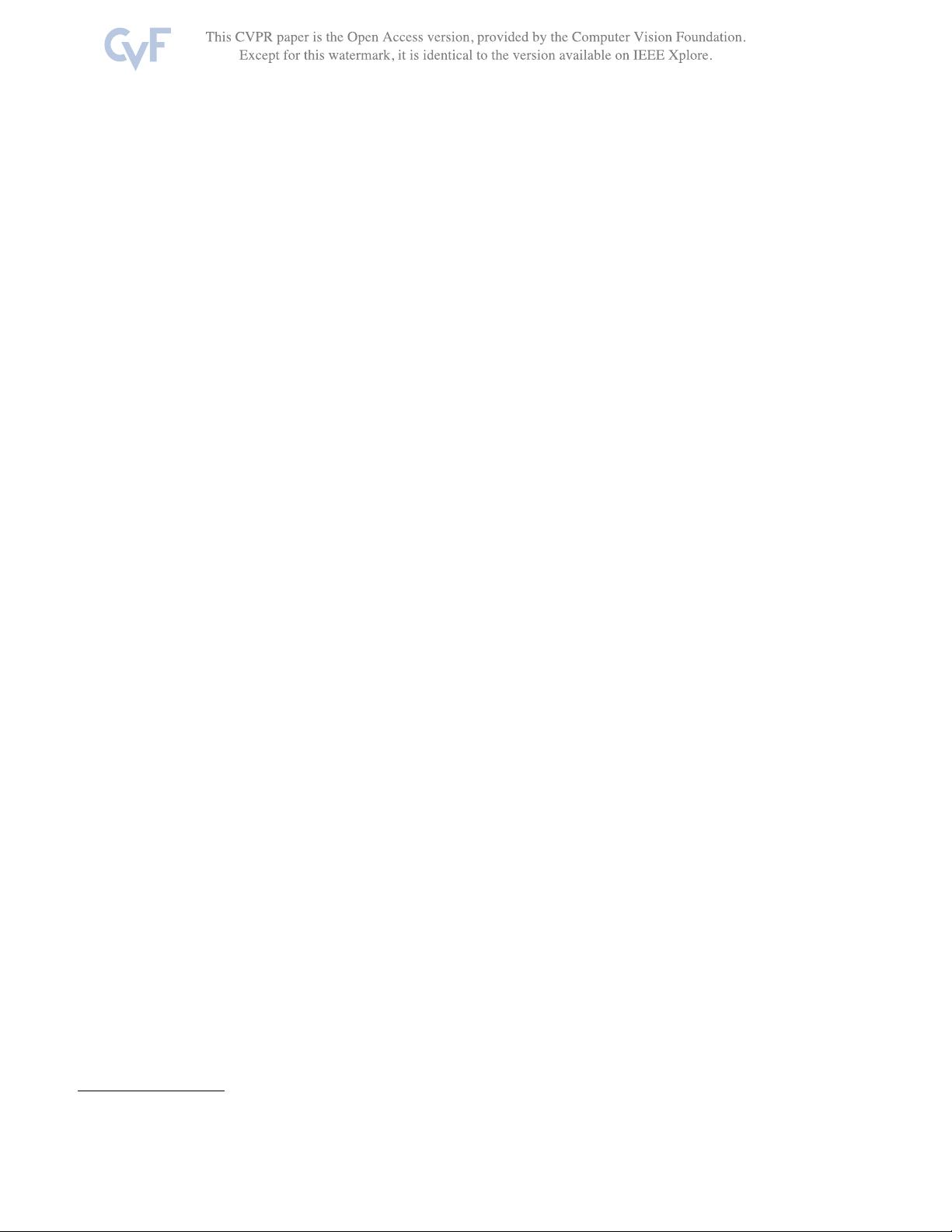
3D Human Sensing, Action and Emotion Recognition in
Robot Assisted Therapy of Children with Autism
Elisabeta Marinoiu
2∗
Mihai Zanfir
2∗
Vlad Olaru
2
Cristian Sminchisescu
1,2
{elisabeta.marinoiu, mihai.zanfir, vlad.olaru}@imar.ro cristian.sminchisescu@math.lth.se
1
Department of Mathematics, Faculty of Engineering, Lund University
2
Institute of Mathematics of the Romanian Academy
Abstract
We introduce new, fine-grained action and emotion
recognition tasks defined on non-staged videos, recorded
during robot-assisted therapy sessions of children with
autism. The tasks present several challenges: a large
dataset with long videos, a large number of highly vari-
able actions, children that are only partially visible, have
different ages and may show unpredictable behaviour, as
well as non-standard camera viewpoints. We investigate
how state-of-the-art 3d human pose reconstruction methods
perform on the newly introduced tasks and propose exten-
sions to adapt them to deal with these challenges. We also
analyze multiple approaches in action and emotion recogni-
tion from 3d human pose data, establish several baselines,
and discuss results and their implications in the context of
child-robot interaction.
1. Introduction
Autism affects the lives of millions of people around the
world. It is estimated that 1 out 100 people in Europe suf-
fers from autism [
1], whereas the Centers for Disease Con-
trol and Prevention estimates that 1 in 68 children in the US
has autism, with a prevalence of male cases over female that
amounts to a factor of 4.5 times higher [
2]. The challenges
the people with autism face when interacting with others re-
volve around confusion, fear or basic misunderstanding of
emotions and affects. They have difficulties using and un-
derstanding verbal and non-verbal communication, recog-
nizing and properly reacting to other people’s feelings, and
fail to respond, either verbally or non-verbally, to social and
emotional signs coming from others.
In contrast, persons with autism cope well with rule-
based, predictable systems such as computers [
12, 29, 24].
Recent developments have shown the advantages of using
humanoid robots for psycho-educational therapy, as chil-
∗
Authors contributed equally
dren with autism feel more comfortable around such robots
than in the presence of humans, who may be perceived as
hard to understand and sometimes even frightening. While
humanoid robots capable of facial expressions could help
improve the ability of children with autism to recognize
other people’s emotions, most studies are based on remote
controlled human-robot interaction (HRI). Less work has
been done to automatically track and detect children’ fa-
cial expressions, body pose and gestures, or vocal behav-
ior in order to properly assess and react to the their behav-
ior, as recorded by robot cameras in unconstrained scenes.
Thus, robot-assisted therapy cannot yet be used for emo-
tion recognition and, subsequently, to enable appropriate
responses to such emotions.
In this paper, we introduce fine-grained action classifi-
cation and emotion prediction tasks defined on non-staged
videos, recorded during robot-assisted therapy sessions of
children with autism. The data is designed to support ro-
bust, context-sensitive, multi-modal and naturalistic HRI
solutions for enhancing the social imagination skills of such
children. Our contributions can be summarized as follows:
• We analyze a large scale video dataset containing
child-therapist interactions and subtle behavioral anno-
tations. The dataset is challenging for its long videos,
large number of action and emotion (valence-arousal)
annotations, difficult viewpoints, partial views, and oc-
clusions between child and therapist.
• We adapt state-of-the-art 3d human pose estimation
models to this setting, making it possible to reliably
track and reconstruct both the child and the thera-
pist, from RGB data, at comparable performance levels
with an industrial-grade Kinect system. This is desir-
able as our proposed models offer not just 3d human
pose reconstructions, but additionally detailed human
body part segmentation information which can be ef-
fective, in the long run, in precisely capturing complex
interactions or subtle behavior.
2158