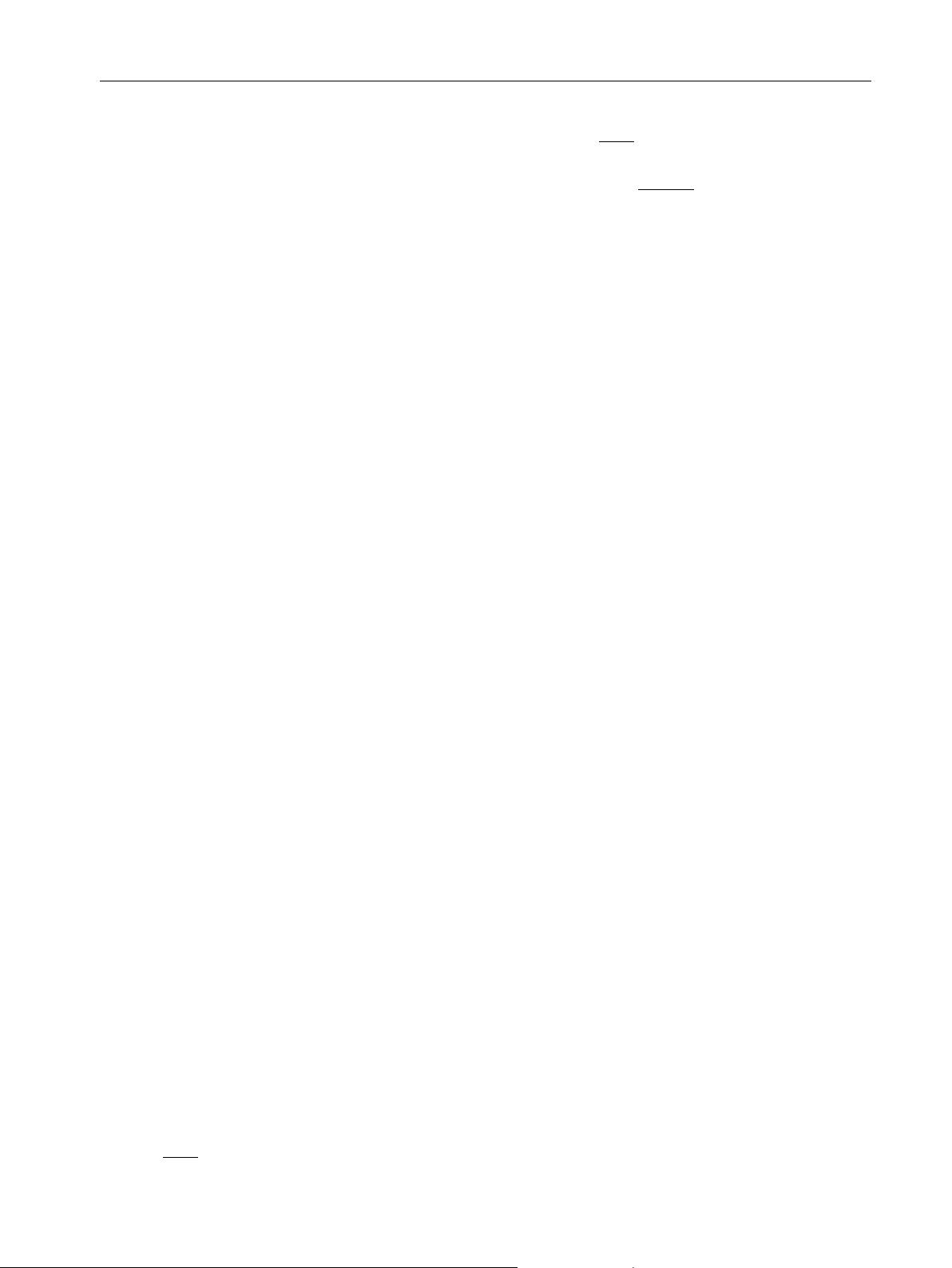
in which
E;N ;U
represents misalignment angle of mathe-
matical platform in local East-North-Upward (ENU) geo-
graphical frame; dV
E;N ;U
is the velocity errors of three axes
(ENU); dL,dl, and dh represent position errors; r
x;y;z
is the
accelerometer biases of three axes; e
x;y;z
is the gyro drifts of
three axes.
Measurement vector Z is defined as follows
Z ¼
P
sins
P
gps
V
sins
V
gps
"#
¼
ðP þ dP
sins
ÞðP þ dP
gps
Þ
ðV þ dV
sins
ÞðV þ dV
gps
Þ
"#
¼
dP
sins
dP
gps
dV
sins
dV
gps
"#
ð3Þ
where P
sins
and P
gps
represent the position of SINS and
GPS, respectively; V
sins
and V
gps
represent the velocity of
SINS and GPS, respectively; P and V represent the real
position and velocity of vehicle; dP
sins
and dP
gps
represent
the position errors of SINS and GPS, respectively; dV
sins
and dV
gps
represent the velocity errors of SINS and GPS,
respectively.
The commonly data fusion method is the optimum esti-
mation based on Kalman filter. KF algorithm is a linear
minimum variance estimation method, which essence is
to obtain the optimal solution of Bayesian estimation by
using recursive algorithm. KF is the optimal estimation
method of linear problem, but it is limited to the state
estimation of linear system.
10,11
The properties of SINS/
GPS integrated navigation system is nonlinearity. How-
ever, Kalman filter directly treated the system model as a
linear model, which will bring large errors, affecting the
performance of the whole system. Furthermore, Kalman
filter will appear divergence phenomenon with the increase
of filtering time. The UKF algorithm was adopted in this
article to solve the nonlinear and filter volatilization
problems.
The basic principle of UKF is based on unscented trans-
formation (UT), which uses a set of deterministic sampling
points to approximate the posterior probability density.
12
The transform process is given as follows:
13,14
Suppose the nonlinear transformation is y ¼ f ðxÞ state
vector x is n-dimensional random vector, the mean value
and variance of x is
x and P
x
, respectively. The nonlinear
transformation points of x are called Sigma points , which
consists of 2n þ 1 vectors
i
(corresponding weight-value
is w
i
). UT is defined as follows
0
¼
xi¼ 0 ð4Þ
i
¼
x þ
ffiffiffiffiffiffiffiffiffiffiffiffiffiffiffiffiffiffiffiffi
ðn þ lÞP
x
p
i
i ¼ 1; :::; n ð5Þ
i
¼
x
ffiffiffiffiffiffiffiffiffiffiffiffiffiffiffiffiffiffiffiffi
ðn þ lÞP
x
p
in
i ¼ n þ 1; :::; 2n ð6Þ
w
m
0
¼
l
n þ l
ð7Þ
w
c
0
¼
l
n þ l
þð1 a
2
þ bÞð8Þ
w
c
i
¼ w
m
i
¼
1
2ðn þ lÞ
i ¼ 1; 2; :::; 2n ð9Þ
in which l ¼ a
2
ðn þ cÞn represents the distribution
of scaling function; a is used to set the distance between
i
and
x; b is used to adjust the accuracy of variance. After
much experimenting, c is set to 0, a is set to 1, b is set to 2.
The mean value and variance of y can be acquired by the
following formulas
y
i
¼ f ð
i
Þ i ¼ 0; :::; 2n ð10Þ
y ¼
X
2n
i¼0
w
m
i
y
i
ð11Þ
P
y
¼
X
2n
i¼0
w
c
i
ðy
i
yÞðy
i
yÞ
T
ð12Þ
The calculation process of UKF includes two stages:
prediction and update. The prediction process is depended
on dynamic error model and past information, and update
process is accomplished by external measurement data.
UKF outputs the differen ces of between GPS and SINS
measurements, and used the output results to revise the INS
errors. UKF outputs the differences between GPS and SINS
measurements, which is used to revise the INS errors.
RBF neural network
There are many different neural network applied to predict
system state, such as wavelet neural network, RBFNN,
back propagation neural network, and adaptive resonance
theory neural network.
15–17
Compared with the other neural
networks previously mentioned, RBF model has faster
learning speed than the other models, and it is simple to
design, furthermore, it doesn’t have the local minima prob-
lems and do not suffer from paralysis pathologies. There-
fore, RBFNN has been extensive used in prediction.
RBFNN operation principle is use the distance between
the input vector and a reference vector RBF is a function to
activate the corresponding hidden u nit by using the dis-
tance between the input vector and a reference vector to
activate the corresponding hidden unit. RBFNN get the
radial basis functions by the previous input a nd output
message and calculate the current output by the current
input and the radial basis functions.
18–20
The characteristic
of RBF is that its response increases or decreases mono-
tonically with the distance between the center reference
vector and the input sample vector.
RBFNN is a three-layer fo rward neural network, the
basic components include (1) input layer; (2) hidden layer;
and (3) output layer. The structure of RBFNN is shown in
Figure 1. The function of the input layer is to transfer the
Zhao et al. 3