没有合适的资源?快使用搜索试试~ 我知道了~
clong1@fb.com, xyzgfu@gmail.com, {zhongyunbao, daquanliu, cxxiao}@whu.edu.cnhttps://github.com/zhongyunbao/Dataset.(a)(b)(c)(d)185420基于深度图像的照明协调0包中云 1 ,龙成江 2* ,傅刚 1 ,刘大全 1 ,李元真 1 ,吴佳明 1 ,肖春霞 1 � †01 武汉大学计算机学院,中国湖北武汉 2 MetaReality实验室,美国加利福尼亚州伯灵格姆0摘要0将前景对象与背景场景进行照明协调是计算机视觉和增强现实社区中的一项重要而具有挑战性的任务。现有的方法主要关注前景和背景外观的一致性或前景对象阴影的生成,很少考虑全局外观和照明协调。在本文中,我们将无缝照明协调形式化为照明交换和聚合问题。具体而言,我们首先应用基于物理的渲染方法构建了一个大规模、高质量的数据集(称为IH),用于我们的任务,该数据集包含具有不同照明条件的各种类型的前景对象和背景场景。然后,我们提出了一种名为DIH-GAN的基于深度图像的照明协调GAN框架,该框架充分利用了多尺度注意机制和照明交换策略,直接推断插入的前景对象与相应背景场景之间的映射关系。同时,我们还使用对抗学习策略进一步改进照明协调结果。我们的方法不仅可以实现前景对象的和谐外观和照明,还可以生成前景对象投射的引人注目的阴影。在我们的IH数据集和真实世界图像上进行的综合实验表明,我们提出的DIH-GAN为基于图像的对象照明协调编辑提供了一种实用和有效的解决方案,并验证了我们的方法优于最先进方法的优越性。我们的IH数据集可在以下网址获取:01. 引言0作为场景编辑的一部分,编辑插入对象的照明以实现场景照明协调。0* 本工作由龙成江和肖春霞共同指导。† 通讯作者。0图1. 单张图像中插入对象的照明编辑。(a)具有照明条件的前景对象图像。(b)具有新照明条件的背景图像。(c) 简单合成图像。(d)照明协调图像。0在计算机视觉[3, 9, 8,39]和增强现实(AR)中,照明协调非常重要,因为不令人满意的照明协调会严重影响用户的真实感。图1中给出了一个例子。即使是经验丰富的专业修图师也很难实现令人满意的照明编辑结果。显然,在没有任何人为干预的情况下自动编辑照明协调是非常具有挑战性的任务。一些先前的工作已经致力于解决这个具有挑战性的任务。特别地,Karsch等人[22]提出了一种支持拖放3D对象插入的图像编辑系统,Liao等人[27,28]提出了一种用于基于图像的对象建模和插入的近似着色模型。尽管这些方法产生了具有感知效果的结果,但它们的性能高度依赖于估计的几何、着色、反照率和材料属性的质量。然而,在某些情况下,任何几何、照明或材料方面的错误或不准确估计都可能导致不吸引人的编辑效果。这个缺点强烈地激发了我们探索一种基于深度学习的方法,直接学习插入的基于图像的前景对象与真实场景之间的映射关系,并实现场景照明协调,而无需任何显式的逆渲染(恢复3D几何、照明、反照率和材料)。显然,一个包含许多训练图像对的数据集,这些图像对包括没有照明协调的合成图像和具有照明协调的对应真实图像,对于训练目的非常有用。185430然而,现有的数据集,如iHarmony4[4]、shadow-AR数据集[30]、HVIDIT数据集[13]等,主要关注的是前景对象的外观或前景对象的阴影,很少考虑全局外观和照明协调。数据集[41]同时考虑了插入前景对象的外观和阴影,但只包含两种类型的前景对象:汽车和人物,这不仅不可用,而且严重限制了照明协调任务的泛化性和鲁棒性。在这项工作中,我们首先构建了一个大规模、高质量的合成数据集,命名为IH数据集,用于对象照明编辑任务。为了构建我们的数据集,我们首先收集了HDR全景图像,从Laval的HDR数据集[11,10]和互联网中捕捉背景图像和照明信息,这些图像在各种室内和室外真实场景中拍摄。因此,我们数据集中的场景是普遍且具有挑战性的。此外,我们还收集了60个具有不同形状和姿势的3D对象模型,用作我们合成图像的前景对象。总的来说,我们的数据集最终包含了89,898个六元组,每个六元组包含一个输入三元组(即一个简单的合成图像,以及相应的对象掩码和背景掩码),以及另一个真实的三元组(即前景对象照明图,背景照明图和最终的照明协调图像)。请参见图2的六元组示例。关于深度学习模型,我们提出了一种新颖的基于场景照明协调GAN框架,名为DIH-GAN,如图3所示,它结合了空间注意力学习[44, 17, 18, 15,36]和对抗学习[12,1],使前景的照明与背景兼容。我们的DIH-GAN以一个没有阴影的简单合成图像以及插入对象掩码作为输入,并充分利用多尺度注意机制和对抗学习来直接推断插入的前景对象与相应背景场景之间的映射关系。此外,我们提出了一种照明交换机制,以编辑对象的照明,并直接实现前景对象与合成图像背景之间的无缝照明融合,使合成图像更加和谐和逼真。值得注意的是,我们提出的多尺度注意机制和特征交换机制起着关键作用,可以避免复杂的逆渲染过程,直接生成合理的照明协调结果。我们的主要贡献总结如下:0•我们构建了第一个大规模、高质量的图像照明协调数据集IH,其中包含89,898个图像六元组,具有多样化的真实背景场景和3D对象模型。0•我们提出了一种新颖的基于深度学习的场景照明协调GAN框架,名为DIH-GAN,它是一个多任务协作网络,可以直接对插入对象进行照明协调编辑,而无需显式的逆渲染。0•大量实验证明,提出的DIH-GAN能够有效实现高质量的图像照明协调,并显著优于现有的最先进方法。02. 相关工作0对象照明编辑。传统的对象照明编辑方法主要集中在估计场景几何、照明和表面反射来编辑对象。以前的方法[7,21]已经表明,对于许多图像编辑任务,对场景几何、反射特性、照明和相机参数的粗略估计效果良好。这些方法需要用户对场景几何和照明进行建模。方法[2]不仅恢复了整个场景的形状、表面反射率和照明,还需要一个粗略的输入深度图,而这种方法不直接适用于照明插入对象。Karsch等人[22]提出了一种完全自动的方法,从单个低动态范围的照片中恢复出全面的3D场景模型(几何、照明、漫反射反照率和相机参数)。Liao等人[28]提出了一种对象重照系统,支持基于图像的重照,尽管这种方法取得了令人印象深刻的结果,但仍需要对对象进行重新塑造和场景建模。这些方法依赖于对象和场景信息的物理建模,不准确的重建结果会导致效果不佳。相比之下,我们的方法自动编辑对象的照明,直接生成协调的照明结果,无需复杂的逆渲染,从而产生更好的视觉效果。阴影生成。最近,随着对抗学习的突破,生成对抗网络(GAN)[12, 1,32]已成功应用于阴影检测、去除和生成[37, 6, 16, 42,30]。对于阴影生成,Liu等人[30]提出了一种ARShadowGAN模型,能够直接建模前景对象的阴影与相应的真实环境之间的映射关系,基于他们构建的数据集。与这种方法类似,我们的方法也旨在生成对象的阴影,而无需显式估计3D几何信息。除此之外,我们的方法还考虑到了对象本身的阴影。我们不仅实现了与Liu等人[30]方法类似的合理对象阴影生成效果,还编辑了对象的照明,实现了整体场景照明协调。2The author Zhongyun Bao purchased the 3D models for non-commercial research purpose only and produced the experimental resultsin this paper. Meta did not have access to these data.185440图2.合成照明协调图像生成过程的示意图。给定具有纹理的3D对象(a),我们首先应用与之对应的全景照明(b)来渲染3D对象并得到基于图像的对象(c)。然后,我们直接将基于图像的对象粘贴到从(d)裁剪的背景图像(e)中,而不进行照明调整。通过这种方式,我们得到了一个具有对象和其周围环境之间照明和阴影不一致的简单合成图像(f),同时具有对象遮罩(g)和背景遮罩(h)。然后,我们使用背景照明图(d)使用Blender合成照明协调图像(i),并将其作为监督学习的地面真实值。请注意,我们将(f),(g)和(h)视为一个输入三元组,将(b),(d)和(i)视为一个地面真实三元组。输入三元组和相应的地面真实三元组在我们的数据集中被视为一个六元组。在电子版本中查看更好。0图像到图像的转换。图像到图像的转换是将输入图像映射到相应的输出图像。它已被广泛应用于各种任务,包括超分辨率[23, 25],图像质量恢复[31, 38],图像协调[19, 4, 29,13]等。值得一提的是,Cong等人[4]提出了一种新颖的领域验证鉴别器,认识到图像协调需要将前景转换为与背景相同的领域,但忽略了在生成器中明确转换前景特征。最近,Ling等人[29]将图像协调视为一个风格转移问题,从背景中明确地构建视觉风格,并自适应地应用于前景,Guo等人[13]基于内在图像理论对图像协调进行建模。这些方法都专注于前景对象的照明,而不考虑对象阴影生成任务。与所有现有方法不同,我们的任务同时考虑了照明对象和生成对象的投影阴影,并实现了整个场景的照明协调。03. 我们的IH数据集0IH数据集的构建过程包括三个步骤:(1)收集图像和3D模型,(2)过滤背景图像,以及(3)渲染和合成。接下来,我们将详细描述这些步骤。收集图像和3D模型。我们首先从Laval的HDR全景数据集1 [11,10]中收集所有图像,并从互联网上捕获了2,686个具有多样化真实场景的HDR全景图像。对于每个全景图像,我们提取8个有限视场裁剪,以生成复合图像的背景图像,并使用以裁剪为中心的相应全景图像作为照明来渲染地面真实结果。我们最初总共获得了22,256个具有相应照明图的背景图像。此外,我们收集了60个3D模型。01 作者ZhongyunBao签署了许可证并在本文中生成了所有实验结果。Meta无法访问Laval的HDR全景数据集。0从网站(https://laozicloud.com)中插入的对象,其中包含各种类型的对象,例如兔子,人物,露西等。过滤背景图像。为了确保我们的对象照明编辑任务的数据集质量,我们进一步过滤了以下三种类型的图像:(1)没有明显或自然的照明,(2)没有合理的虚拟对象插入位置,以及(3)不显眼或没有阴影。通过这种方式,我们最终获得了12,253个剩余的背景图像。渲染和合成。使用Blender渲染使用收集的3D模型,背景图像和相应的全景地图生成地面真实物体照明图像(参见图2(i))。具体而言,我们首先在插入对象的底部指定一个平面以投射阴影,然后将3D对象嵌入裁剪的背景图像中,最后使用相应的全景地图渲染物体的照明以生成最终结果。请注意,由于相应的背景是真实世界的2D场景图像,我们使用Photoshop手动注释我们数据集中的每个前景对象以获得准确的遮罩。在构建过程中,我们使用了60个具有不同姿势配置的虚拟模型,使用图2中显示的流程基于不同的背景图像构建我们的数据集,并总共生成了169,672个合成的地面真实照明调和图像。此外,为了提高训练效率,我们最终只使用了89,898个六元组来训练我们的网络。每个六元组包含两个三元组。一个作为输入数据的三元组包括一个没有照明调整的简单合成图像,以及相应的对象遮罩和背景遮罩。另一个作为地面真实数据的三元组包括一个合成的照明调和图像,一个对象照明和一个背景照明的地面真实值。图2显示了一个可视化的六元组示例。有关数据集分析的更多详细信息,请参阅补充材料。Figure 3. The overview of our proposed DIH-GAN. Given an input image with inserted object and the corresponding object mask andbackground mask, the generator of our DIH-GAN can generate the relighting image (R-Network marked in blue) and predict both objectillumination and background illumination (I-Network marked in black), and the discriminator can distinguish whether the generated re-lighting image is real or fake. The Illumination exchange mechanism between the R-Network and the I-Network realizes the conversion ofillumination information between the scene and the object.4. Proposed MethodOur goal is to train a GAN that takes a naive compos-ite image ˆY with inharmonious illumination, correspond-ing the object mask, background mask and correspondingtarget illumination as input, and directly generate the cor-responding scene illumination harmonized image ¯Y .Toachieve this goal, we propose a novel framework calledDIH-GAN, of which the generator is a multi-task parallelnetwork composed of two networks, i.e., Relighting Net-work (R-Network) and Illumination Network (I-Network)to handle object and illumination separately. See Figure 3.4.1. GeneratorAs shown in Figure 3, the generator of our DIH-GANcontains two parallel branch networks, i.e., R-Network andI-Network. R-Network learns the overall features of theinput image and I-Network predicts the object and back-ground illumination. They work collaboratively to completethe task by illumination exchange mechanism.Relighting Encoder. For the U-Net [33] like R-Network,there are five down-sampling blocks in encoder and eachdown-sampling block consists of a residual block with3 consecutive convolutions, instance normalization andReLU operation and halves the feature map with an averagepooling operation. Each down-sampling block is followedby a multi-scale attention block which guides the networkto infer the object shadow and generates the refinement fea-ture maps. Note that we design such a multi-scale attentionmechanism for two purposes: (1) to adaptively extract re-Figure 4. Illustration of our multi-scale attention mechanism.liable multi-scale features and overcome the scale-variationacross the image to assign larger weights to areas of interestfor refinement; and (2) to guide the generation of shadowsof the inserted objects by paying attention to real shadowsand corresponding occluders in the scene.As shown in Figure 4, the multi-scale attention block hasthree types convolution layers with three different kernelsizes, 1 × 1, 3 × 3, 5 × 5, to extract features in differentscales. Specially, for the input feature map, the multi-scaleattention block first extracts features using two 1 × 1 con-volution layers with crossing channels and squeezing fea-tures, two 3 × 3 convolution layers and two 5 × 5 convo-lution layers to generate feature maps. Note that for 3 × 3and 5 × 5 convolution, the feature map size of each channelhas been changed and therefore we apply an up-samplinglayer to recover the original size before feeding the featuremap into the Sigmoid function to produce attention map.We separately conduct an element-wise multiplication on18545the input feature and the attention map at each scale to pro-duce attended feature maps, which are then concatenatedat channel-wise together and fed into a 1 × 1 convolutionlayer to recover the same channel number with the origininput feature. We apply a residual structure [14] to combineit with the origin input feature map together as final output.This residual mechanism not only accelerates the conver-gence speed but also correct image details such as borderartifacts.The final output features of the encoder include theillumination features Fillu and non-illumination featuresFnoillu of global image. This feature separation is enforcedby the no-illumination loss Lnoillu (see Eq. (6)).Illumination Encoder. For the I-Network, the encoder hasa similar structure to the one of the R-Network and takes theresult of multiplying the input image and background maskas input to extract the illumination feature of background.The background illumination feature is then exchanged withthe object illumination feature of R-Network by the illu-mination exchange mechanism.Note that the output ofillumination encoder have two functions: the backgroundillumination features are used in combination with objectnon-illumination features Fnoillu of R-Network encoder toproduce the illumination harmonized image in the decoderof R-Network; the background illumination features arealso fed to the decoder of I-Network to predict the corre-sponding background illumination information through su-pervised learning.Illumination Exchange Mechanism. After obtaining thefeatures extracted by the two encoders, inspired by [29], weuse the background illumination features in the I-Networkto guide the foreground object illumination features in theR-Network, and exchange them for the input of the de-coders.To specify, the two sub-networks work together throughthe illumination exchange mechanism. It is worth mention-ing that at the bottleneck feature of the R-Network, we per-form multiplication operation on it with the object mask ofthe corresponding size, and get the normalized foregroundobject features F obj by using IN [35]. This treatment isable to better realize the exchange of object illuminationand background illumination, and achieve the illuminationharmonization task.The normalized foreground object feature F obj can bedivided into two parts: non-illumination features F objnoilluwhich is independent of illumination feature F objillu. The il-lumination feature F objillu have two functions: one is to becropped by the resized object mask, rescaled to a larger sizeand then fed into the object illumination decoder of the I-Network to predict the object illumination. The other is tobe affined by learned scale and bias from the backgroundillumination features F bgillu extracted by the I-Network en-coder, and then the affined features are concatenated withaffine = αcF objillu + θc,(1)θc = Nbg h,wF bgillu,(2)αc =�1Nbg�h,w(F bgillu − θc)2,(3)Ltotal =β1Lillu + β2Lnoillu + β3Lper+ β4Ladv + LRecons,(4)185460F obj noillu 并输入到 R-Network解码器中生成逼真的照明调和图像。仿射结果计算如下:0其中 θ c 和 α c 是通道 c中背景照明特征的激活的均值和标准差:0其中 N bg 是背景照明的像素总数,h, w分别表示特征的高度和宽度。在整个照明调和任务中,我们对照明图像、物体的非照明和照明特征以及背景照明特征分别进行了监督约束,从而提高了照明调和图像的准确性。照明调和解码器。R-Network中的解码器由五个上采样层组成。每个上采样层通过最近邻插值、连续的扩张卷积、实例归一化和ReLU操作将特征图扩大两倍。最后一个特征图通过sigmoid函数激活。R-Network通过跳跃连接连接下采样和上采样层。物体/背景照明解码器。根据[40],I-Network的解码器用于预测照明。本文中,我们使用相同的结构来进行物体照明和背景照明解码器的预测。04.2. 判别器0DIH-GAN的判别器旨在帮助R-Network加速收敛并生成一个合理的调和图像。根据Patch-GAN[19],我们的判别器由六个连续的卷积层组成。每个卷积层包含卷积、实例归一化和ReLU操作。我们使用Sigmoid函数激活由卷积产生的最后一个特征图,并在激活的特征图上执行全局平均池化操作,以获得判别器的最终输出。04.3. 损失函数0总损失 L total 由照明损失 L illu 、非照明损失 L noillu、感知损失 L per 、对抗损失 L adv 和经典的 L 1-正则化重构损失 L Recons 组成,如下所示:Lillu = ∥YOI − ¯YOI∥22 + ∥YBI − ¯YBI∥22,(5)Lnonillu = (F 1nonillu − F 2nonillu)2/Nnonillu,(6)Lper =MSE(VYF I, V ¯YF I) + MSE(VYBI, V ¯YBI)+ MSE(VYR, V ¯YR),(7)where MSE is the mean squared error, and Vi = VGG(i) isthe extracted feature map.Adversarial loss Ladv is utilized to describe the compe-tition between the generator and the discriminator as:Ladv = log(D(x, m, Y )) + log(1 − D(x, m, ¯Y )),(8)where D(·) is the probability that the image is “real”. x isthe input image and m is the corresponding mask, ¯Y is theoutput of the generator of DIH-GAN, and Y is the ground-truth. The discriminator tries to maximize Ladv while thegenerator tries to minimize it.4.4. Implementation detailsOur DIH-GAN model is implemented by Tensorflow andruns with NVIDIA GeForce GTX 1080Ti GPU. We splitthe 89,898 six-tuples into 71,918 six-tuples for training and17,980 six-tuples for testing. Note that, there is no crossoverbetween the foreground objects in our training dataset andtesting dataset. Our network is trained for 80 epochs, andthe resolution of all images for training and testing is 256 ×256. The initial learning rate is 10−4. We set β1 = 25.0,β2 = 6.0, β3 = 0.04, β4 = 0.5 and adopt Adam optimizer tooptimize the DIH-GAN and discriminator.5. Experiments5.1. Evaluation Metrics and Experimental SettingsEvaluation metrics. To evaluate the performance of ourDIH-GAN, we adopt two commonly-used evaluation met-rics including RMSE and SSIM. In addition, we also in-troduce other two evaluation metrics including fMSE andfSSIM to evaluate the performance on foreground regions.These two metrics are to compute MSE and SSIM val-ues between foreground regions of input and correspond-ing ground truth. Overall, smaller fMSE, RMSE, and largerfSSIM, SSIM indicate better results.Compared methods. We choose one traditional relightingmethod ASI3D [22] with the similar task as ours, and otherthree deep learning-based methods from the related fields:one shadow generation method ARShadowGAN [30], andtwo image harmonization methods including DoveNet [4]and Intrinsic-Net [13]. For fair comparison, we re-train AR-ShadowGan, DoveNet and Intrinsic-Net on our training set,and test them on our testing set for our illumination harmo-nization task.5.2. Comparison with Start-of-the-Art MethodsQuantitative comparison. Table 1 reports the quantita-tive comparison results on our testing set. As can be seen,our DIH-GAN achieves the best quantitative results on allthese four evaluation metrics. This is mainly because thetraditional methods ASI3D rely on the estimation accuracyof 3D information of objects and scenes. Inaccurate esti-mation of 3D information often leads to poor results. Asa deep learning based method, our DIH-GAN does not re-quire complicated 3D information estimation and insteadit uses the attention mechanism to enhance the beneficialfeatures for a better result. The best performance of DIH-GAN is mainly attributed to the multi-scale attention mech-anism, feature exchange mechanism and adversarial learn-ing, which can better guide the illumination editing of in-serted object, refine the features and bridge the illuminationgap between inserted object and background environmentto obtain results closer to the ground truth.Visual comparison.We provide some visual compari-son results in Figure 5. As we
下载后可阅读完整内容,剩余1页未读,立即下载
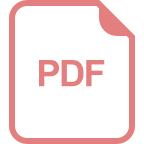


















cpongm
- 粉丝: 4
- 资源: 2万+
上传资源 快速赚钱
我的内容管理 收起
我的资源 快来上传第一个资源
我的收益
登录查看自己的收益我的积分 登录查看自己的积分
我的C币 登录后查看C币余额
我的收藏
我的下载
下载帮助

会员权益专享
最新资源
- VMP技术解析:Handle块优化与壳模板初始化
- C++ Primer 第四版更新:现代编程风格与标准库
- 计算机系统基础实验:缓冲区溢出攻击(Lab3)
- 中国结算网上业务平台:证券登记操作详解与常见问题
- FPGA驱动的五子棋博弈系统:加速与创新娱乐体验
- 多旋翼飞行器定点位置控制器设计实验
- 基于流量预测与潮汐效应的动态载频优化策略
- SQL练习:查询分析与高级操作
- 海底数据中心散热优化:从MATLAB到动态模拟
- 移动应用作业:MyDiaryBook - Google Material Design 日记APP
- Linux提权技术详解:从内核漏洞到Sudo配置错误
- 93分钟快速入门 LaTeX:从入门到实践
- 5G测试新挑战与罗德与施瓦茨解决方案
- EAS系统性能优化与故障诊断指南
- Java并发编程:JUC核心概念解析与应用
- 数据结构实验报告:基于不同存储结构的线性表和树实现
资源上传下载、课程学习等过程中有任何疑问或建议,欢迎提出宝贵意见哦~我们会及时处理!
点击此处反馈


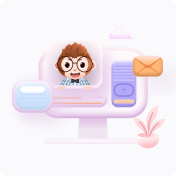
安全验证
文档复制为VIP权益,开通VIP直接复制
