没有合适的资源?快使用搜索试试~ 我知道了~
13518木偶 :仲裁肖像animation 的with Semantic-aware 应用转化Zhuo陈1,2Chaoyue 的wang 2BOyuan 1Dacheng陶21深圳 国际 研究 生 院 Shenzhen International Graduate School ,Tsinghua大学2 UBTECH 悉尼 人工 智能 中心 , 计算机 科学 学院工程 学 ,The大学Of悉尼 ,达林顿 ,NSW 的2008,澳洲z-chen17@mails.tsinghua.edu.cn,chaoyue.wang@sydney.edu.au,yuanb@sz.tsinghua.edu.cn作者 : 陈 文 涛@sydney.edu.auFigure 1:Examples of animated portraits generated by the proposed PuppeteerGAN.(动画图片由拟好的PuppeteerGAN生成)结果是相同的姿势。ASThe Target 目的frame( Left )column )While保持The SAME Appearance Of The来源( TOP )罗 ) 。 As shown于The来源图像 , 我们 的 方法 可以 应用 于 各种 肖像 , 包括 彩色 照片 , 黑白照片 , 绘画 , 卡通特点 及 高 分辨率Images 。Abstract从目标帧中提取的肖像动画是一种用于许多现实世界娱 乐 应 用 的 重 要 技 术 。 Although recent works hasachievedhighlyrealisticresultsonsynthesizingorcontrolling human head images , the puppetering ofarbitrary portraits is still con - following challenges :( 合成 或 控制 人脑 图像 的 高 现实 结果 , 任意 肖像 的 木偶 仍 在 继续 , 面临 着 随后 的 挑战 :( 1 ) 身份/人格 不 匹配 ; 2 ) 数据/域 限制 ;而( 3 )1. Zhuo陈has Been A A参观PhD Student 学生at UBTECH Sydney悉尼AI 中心 ,School 学校Of计算机科学 ,Faculty 教授Of工程学 ,The Univer-sity of Sydney , since January 悉尼 大学 1 月 以来2019.13519低效率的培训/微调。In this paper , 我们 开发 的AA NOVEL双 阶段Framework 工作called解决 这些 挑战 的 Puppet 。特别是,我们首先学习了identity—preserved语义段动画,这在任何肖像之间执行了来回定位。作为一般表示,语义段结果可以适应不同的数据集,环境条件或应用域。Furthermore , thesynthesized semantic segmentation is filled with ap -pearance ( 综 合 语 义 分 割 已 被 置 入 ap -pearance 中 )Of The来源肖像 to this终 ,an应用 转化 网络 是 联合 考虑 对 语义 特征 和 条件 生成 的冲击 而 产生 可靠 性 输出 的 。训练 后 ,The13520两个网络可以直接执行端到端对未看到的主题的引用,而无需任何再培训或微调。在 跨 性别 身份/领域的 扩展 实验/解决 情境 演示 了 所 提议 的 木偶 的 优越 性Speed 的 。1. Introduction介绍包括 绘画 、 照片 或 其他Artis - 人类 的 代表 永远是 计算机 视觉 和 图形 中 最 重要 的 研究 对象 之一 。在 这项 工作 中 , 我们 考虑 了 por 的 任务 - 训练 动画 , 其中 aimate a given 肖像 我们 - ingPOSE 的提供By驱动 器Frames 。 this童Of技术 有 很 大 的 吸引力 , 因为 它 在 电影 行业 的 潜在 广泛 使用 。7) ,则 artists (47 ◎ 个人 化 媒体 一代(35].在短短的几年里,随着深度学习算法的快速发展,一些深度生成模型正在致力于解决肖像动画任务。Among Them ,One kind of solution is trained todecomposethedisentangledappearanceandposerepresentations from the input portrait image ( 一 个 解决 方案 是 训练 来 解析 印度 - gled appearance 和 poserepresentations from the input portrait image )36, ,25, ,46].最 佳 状态 : By Recombining TheAP -Pearance ( 对等 )feature 特性Of The来源肖像withThe pose目标 帧 、 生成 器/解码 器 的 功能 被 支持 生成 所 需 的 输出 。“ 你 的 承诺 已经 取得 了 进展 ,挑战 重新 认识 提取 的 现状 , 并 提出 了 一些 看 不见 的 表现 , 如 图片 或 视频 。Inference 。常见 问题 解答 : _38, ,18, ,31” 他们 被 安排作 为 一个 孩 子。 Of replacement 替 换 Of TheDisentangled 的pose介绍 |在 (47, ,33, ,10 [ ,given the landmarks of the target frame as input , thenetwork is trained to reconstruct the target frame byconditioning on appearance information extracted fromother ] . 给 目标 框架 的 着陆 点 作为 输入 , 该 网络已 被 训练 以 重建 目标 框架 由 其他 应用 信息 提取的frame Of The SAME视频/人物 Compare两 个DirectlyDisentanglingposerepresentationsfromimages,methods using ( 不 可能 的 图像 , 使用 方法 )FacialLandmarks are more鲁 棒而usually 经常with bet 世代 结果However , 持续 的 推理 , 可能 会 在 驱动 的 地标和 地标 之间 遇到 错误 签名The来源肖像(E . G。Different 不同Face 脸形状 ) ,Which will results to 穷的 结果此外 , 考虑 appear-ance 表示 可能 无法 从 未经 见过 的 图像 中 提取 出来 , 这些 图像 随后 使用训练 数据 进行 不同 的 分布 [4, ,22 " , the few-shot fine-tuning strategy is employed to learn accurateappearance information " ( 少数 微调 策略 已 有利于学习 准确 的 吸引 力 信息 )18, ,45]. By Uti-Lizing的A A Few images Of The SAME person 个人两 个FineTune 的The预 训练 模型 , 这些 方法 将 实现 更 好 的结果每 一 个 具体 的 身份 。however , 在 现实 世界 中applications , the fine-tuning would cost much time andcomputa-tion resources than simply feed forward 调整 后 的成本 会 比 以前 多 很多 时间 和 计算 资源Inference 。在 那 之前 ,Although ( 英语 : Although )recentworks Have Achieved孔13521搜索 结果 关于 合成 或 控制 肖像 , 我们 很 生气that The Puppeteering 的Of arbitrary 任意肖像IS静第一 首 : Bled By The Following Challenges( 1 ) 与提供 者 的 动画 来源 图像 之间 的 身份/人格 错误匹配 。驱动 器frame ; 2 )培训数据/domain Pre-trained Model Failing to Understand - Seen Portraitsfrom other identities , domains or 看到 的 导致 预训练 模特 无法 理解 的 限制的 决议 ;3)3 、 在 现实 世界 中 , 微调 什么 可能 成本 一个 可 信赖 的 时间 和 计算 资源applications 。在这项工作中,我们提出了一个新的两阶段生成框架称为PuppetterGAN用于任意肖像ani—mationtasks。不同于 现存 的 情景/场景 - 构图 策略 , 我们 分 开 的 动 画 图 TAGS : pose 重 定 向 而Appearance转变 。在 第 一 阶段 , 我们 坚持 在 语义 段 之间 进行 身份 表述 的 返回 定位 。各种 肖像 画特别 是 , 一 个 Sketching Network 是 训练 来综合 的The Animated 的segmentation 部分Mask而2013 中国 特色 活动 (E . G。图像来源:Thesource portrait yet with the same pose as the drivenframe自生成的结果可以作为对肖像不同儿童的一般表示,我们可以模拟—在特定的谈话头视频数据集上进行绘图网络训练,但对任意肖像进行推理。在 第 二 阶 段 , 我 们 开 发 了 一 个 AppearanceTransforma—tion Network to Fill in the AnimatedSemantic Segmentation mask with the Appearance ofthe source portrait。此 网络 - 工作 条件 的 一 个appearance 编码 器 , 一 个 分段 掩码 有 条件 的 解码 器 和 所 提议 的 基于 Warp-based 语义 感知 软件skip connections 的( WASIC ) 。 The coloringnetwork 截图makes Full use Of The文本information信息通过 所 提出 的 WASIC 的 编码 器 层 提取 ,因此 , 它 避免 了 微调/再 训练 步骤Inference 。最 后 , 我们 将 展示 我们 方法 的 生成 肖像onvarious Experiments 包 括 自 行车赛, cross-identity/domain/resolution ( 身份 信息/域 解析 )CASE 问题而Compare 比较The五 种 不同 的 方法proposed method with five different method 41, ,45, ,1, ,43, ,40].实验 结果 表明 我们 的 方法 在 可靠 性 , 现实 性 , 通用 性 和 推理 方面 的现有 工作 优越Speed 的 。2. related 相关Works基于变形的方法。基于 传统 的 畸形Algorithms算 法 Compute A A transformation from The sourceportrait to the target pose based on facial landmarks orop-tical flow . ( 来源 : 目标 情景 基于 工厂 地标或 Op-tical 流程 。从 广义 上 讲 , 偏差 非常 大 ,有些 方法 (20, ,3, ,11 [ 建立 一 个 面部 图像 数据 库 ] Of The来源person 个人两 个返回The大多 数模拟 器首先 , 将 目标 作为 变形 的 基础 。虽然 这些methods 方法成功于Face 脸Mimic为The具体地source person , collecting and pre-processing , sucha large ( 来源 人 , 收集 和 预 处理 , 如此 大量 )数据13522JIfor each source person is a high cost in 每 一 个 人 都 是高 成本Practice 。Averbuch的艾尔·。(1 [ 1 ] Detects additional keypoints around the face on the source , and target image inorder to control the deformation of the whole head . ( 检测 源 上 的 其他 关键 点 , 以 控制 整个 头部 的 变形 )通过从目标中复制毛里求斯地区,该方法可以在没有任何培训或额外数据库的情况下详细说明面部表达。( 重 定向 自 X2 Face )43 [ 学习 一 对 2D 像素化 的 改革 - 从 源 Pose 到 前端 的 想法 和 可以 从 多个 可选 媒体 中 提取 的 目标 姿势 - 包括 视频 , 音频和姿 势的角 度。 基 于变 形的Overall ,Deforformation Basedmethods 方法are efficient 有效 的于transferring Facial 2 . 两 个 相对 较小 的 计算 成本 ,表现 出 两 个 身份 可靠 性 和 面部 现实 性 。首页 >外 文书 > 人文 > 心理 励志 > A Large Motion of theHead or the Synthesizing of the Hidden Part Will Lead toDiis肖像视频to video。培训一个特定的网络—一个单一的人可以显著提高所产生的肖像的质量。x2 = (40 [ CanGenerate Tempo , Rally Coherent Videos Conditionedon Segmentation Masks ] 产生 速度 , 非常 流畅 的 视频 而 POSE 的 After Being 训 练 on The 具 体 地 VideoSequences of the Same Appearance 同样 的 外貌 。2013年 12 月 28 日 @ 上 午 11 时 28 分 i 'm adaptiveadaptation (39 [ 学习 合成 的 视频 的 前 un - seensubjects or scenes by leveraging few example images ofthe target at test time . ]( DeepMind ) (7编译 自 :build an autoen - coder Framework 工作顾问Of an poseEncoder 的而an Appear-ance - Decoder 的 。在参考阶段,他们采用了驱动身份的编码器来提取姿势,并确定来源人以恢复appearance。因为 重新 实施 和 培训过程 很 容易 遵循 , DeepFakeBecomes popular为Face脸Swapping 交换applications 。阿信 (44 [ 视频 ] 以 面部 边界 转变 为 源头 的 身份 指导 。《 While The 》Encoder 的CAN be天才 ,The decoder而Transformer 的每 一 个 来源 都 有 其 特定 的 身份 。3D变形模型方法即每个给定来源的人所需的个性化培训例如,Hyeongwoo艾尔·。(17 [ 训练 一 个 渲染 到 视频 的网络 , 用 源 的 插图 和 身份 设置 3D 模型 的 表达 。(34学习 的 爱 ( aims to learn )accurate 。Lip motion驱动 器By The视频 ,While现在 -Figure 的二 :The complete 全部Framework 工作Of The提议method . Animation Portrait in Two 两 个 人 的 动画TAGS :( 1 )pose重 定向By The Sketching 的Network而2 )APPEAR - - COLORING 变换 的 机会网络 。VariousPresentation 介绍are introduced 的AS The动画肖像 的 条件 信号 。火星 (45, ,33, ,10 zh -tected from the driven frame are the most frequently useddescription ( 英语 : Most frequently description )OfThe Target 目的pose 。 Nirkin 。et AL。 (26]而Geng艾尔 ·。(12” [ 1 ] 这 是 一 个 基于 地标 的 目标 的 输入 , 并 进一步 在 缺失 的 部分 使用 了 一 个 绘制 算法 进行 填充 。Pumarola的艾尔·。(29使用 交流 单元以 类似 的 方式 。Although these methods per - formwell in some cases , as mentioned before , using thelandmarks detected from the driven frame 可能 会 导致在 出现 重大 差距 时 的 身份 错误 匹配 问题The来源而驱动 器肖像 pagan 的(23]生成 的 视频 的 照片 - 现实 面 条件 对 3D 表达 的 变形 , 这 是 只 适用 于 面部 区域 , 而 不是 整个 肖像 。( 重 定向 自 SomeWorks )27, ,2, ,32英文 名 : Disentangle theShape and图像 特征 来自 通过 高级 学习 的 肖像 。- -虽然The learning Of Identity 身份而pose feature 特性CAN开发 商 : Midigate The Personality 人格mismatch错误问题 ,The joint learning Of Pose retargeting andappearance is a challenging task and may limit by thetraining . ( 过度 调整 和 态度 是 一 项 挑战 任务 , 可能 会 限制 训练 )数据/域 。3. method给予A A来源肖像i s而A A目标/驱动frame i T,IJ当前 位置 : 线 上 翻译 > 英语 翻译 > merous hours ofthe target person 's speech footage is至关 重要为训练 。The提议傀儡AIMS两 个generate an产出IM -agei˜s,whose Identity 身份IS The SAME AS i s,也 就是 说 , 即使BeingJIAlthough 的video to video 视频methods 方法are able 的两个generate现实肖像Even with Large 大motion ,The Identity 身份间谍consistent with 什么i Tpose and expression 的 意思 除 此 之外 ,TheFacialLandmarks l s/l T而Semantic 语义segmentation 部分面具IJ太平洋 培训 人口 最 多 的 用户this技术 : 难以 收集 培训 数据 , 高 计算 成本 和 长期推断时间 。条件图像合成。从 ( 有 条件 ) 生成 高级 网络 的 进展 中 受益 ( GANs )48, ,6, ,37, ,28, ,21[ 其他 ] 基于 合成 的 方法45, ,26, ,43] are提议两 个13523generate fake肖像using The 1 . 目标 的 来源 和 姿态( 或 面部 表达 ) 的 身份image .m sAre detected for assisting our portrait animation pro -ccess . 我们 已 检测 到 协助 我们 的 肖像 动画 。In thefollowing , we introduce our pipeline 在 下 一 阶段Section3.1. Section 3.2 Section 的3.3演示 草图 和 着色 网络respectively 。3.1. modelFramework 工作As通知 书 ,some 一些Generation 一代基于methods方 法 syn - thesize the animated portrait with the faciallandmark de - tected 相关 文章 :on The驱动 器frameAS The条件信号 | com - -13524IFigure 3:网络涂鸦与着色网络的训练过程。Sketching Network(Left)概念图例如:a generator and adiscriminator,two encoders(for segmentation mask and landmark);以太 坊 2013 (30包括 编码 器 、 生成 器 和基于 Warp-based 语义 感知 软件 的 skip-connections ( WASIC ) 。We Illustrate the Structure of WASIC in theDotted Box ( 我们 在 笼 中 说明 了 WASIC 的 结构 )TOP ) 。pared 的with Facial Landmark ,The segmentation 部分面具IS A A这是另一个人的标志。 m s而m sdenote 。TheIJpixel-wise 图像 的 语义 分类 ,Which表面的外部区域,就像耳朵,头发和颈一样好。于是 , 我 们 决 定 先 把 《 易 经 》 讲 出 来 。segmentation 部 分 面 具 而 use Facial Landmark ASauxiliary to generate realistic 一般 现实 的Images 。We提议A A双 阶段Generation 一代pipeline 管道( Fig. ) 2)包括素描和着色网络。 首先 , 身份segmentation masks of the source portrait at pose 图片 来源I而J。Figure的3—left illustrates the sketching network的结构。网络 包括 四 个 组件 , 国家 编码 器E l首页 > 外文书 > 人文 > 心理 励志 > The Segmentation Encoder Em《 身份 歧视 者 》 ( The Identity Discriminator )DiThe Joint Generator 联合 发电 机g R。The generationprocess 可以 公式 化AS :(l˜s,m˜s)=g。ssΣPreserved 的pose重 定向IS已 solved于The FIRSTSketching 的JJR E l(lJ)- E m(mI)。(1)STAGE。Specifically , we take the segmentation mask我们 特别 地 拿走 分段 面膜m sof the source portraitand land mark of the source 图像 与 地标l T英文 名 : OfThe Driven Frame AS上 一篇 : generated segmentation mask and landmarkshould 是 什么 意思beTheSAME AS m s而l s,Which IS Detected from frame JOfJJJinput , intending to generate the target ( 生成 目标 )segmentation 部分面具视频/身份 识别s。重建失败的原因是:m˜s而Landmarkl˜s。 through 通过introducing 介绍anIdentity 身份戴 -l(E ,E,g)=||l˜s― ―ls||,( 2)JJldlmRJJCriminato 。r,Thegenerated 产生m˜s而l˜sareLearned 学习两 个be孔JJsTl(E,E,g)=||m˜s― ―m s||。(3)永 恒with The Identity 身份Of m I而The pose Of l J。since TheMASK 与 FACIAL LANDMARK COULD BE APPLIED相关 数据两 个any肖像 ,this module IS Naturally adaptive 适应两 个images of 不同 的Domains 。In the second stage , our coloring network 性能 ap -pearance transformation conditioned on the generated 第二 阶段 , 我们 的 着色 网络 性能 ap - 平滑 变换 条件下海 -mentation 的面具m˜s而The来源肖像is。 受益fromseglmRJJidentity—preserved pose retargeting特性。曲名 :Order to Avoid 身 份 /Personality mismatch , WeIntroduce 介绍an Identity 身份戴 -criminator 犯罪 者D i(9].曲名 : The input of D i一 对 夫妻 的 特 徴 分析IsapairoffeaturesextractedfromTheFacialLandmarks By E l。 The discrimi - nator - 发音 ( 英语 )IS required 需要两 个determine Whether 什么The IDENTITY Of The Landmarks are the same or not .同样 的 , 或者 不是 。一 步 一 个 脚印 ( For eachstep , a real )pairJIgenerated segmentation mask , this stage generates 生成此 阶段 的 掩码REAL -(E l(l s)- E l(l s) ) )一 对 假 夫妇 And aFake Pair(E l(l v)- E l(l s)))IJI”JisticOutputs基于on The Precise geometry 的指导而13525I”不需要支付与目标的一致性的注意。In Order to MakeFull Use of the Texture ( 英 语 : In Order to Make FullUse of the Texture )特征 提取 自 编码 器 网络 的shallow layers ,we devised The 基 于Warp 关 于Semantic Aware Skip 连接 ( WASIC ) 。它们被用于训练。这 意味 着 我们 的 火车 。E lfoolthe discriminator . ( 欺骗 歧视 者 )6 . 《 土地 编码 器的 输出 》 The Outputs of Landmark Encoder E l肖像Thepose而expression implicitly 相关While Being iden - titleindistinguishable 。adversarial training process 可以 被定义AS :l IDT(E l,D m)= min max D i(E l(l s),El(l s))ElDiIJ (四)3.2. Sketching 的Network― ―D i(E l(l v),E l(l s))。I”JDuring 持续 时间训练 ,The input Of The Sketching的Network ISA AGroup Of Landmarks而segmentation 部分Maskdenoted 的所以 (l s, ,l s, ,l v, ,m s, ,m s].l s而l sFacial Landmarks 的 数据3 、 教育 网络 的 总 成本IS :l seg(E l,E m,g R,D i)= l ld(E l,E m,g R)IJI”IJIJ(五)Detected from两个帧在同一个视频序列。 l v+λ1·lseg(E l,E m,g R) + λ2·lIDT(E l,D i)。13526JJIJJSince the sketching network deals with the pose retar -sign on semantic guidance ( segmentation mask andMark( Mark ) instead Of The REAL 肖 像 , this moduleCAN be博 -Turesfrom The Encoder 的 两 个 The decoder 。 特 别地, 给 予 An input group ,we first compute aformerated transformation parameter 我们 首先 计算 了一 个 表达式 变换 参数θ wFrom the source land mark 相关l sTo The Target 的l s。IJtentially 一般APPLIE 应用两 个Different 不同Kinds Of肖像Regardless 的of the domain gaps.领域的差距。Through thedisentanglement and re - - 身份 与 姿态 特征 的 结合Sketching 的for the 的K th网络 层 , 编码 器 的 中间 输出 和 通信输出decoderAre Denoted As 其它 优惠/消息e(K]s而D(K]s, ,第 一个 在 " The Two Features Are OfIJPhaseCompletes The pose重 定向task precisely 珍贵 的于our 我们TheSAME Size 。 两者m s而m sare居住两 个The equal 的IJFramework的。 The Outputs Of The Sketching 的Network威尔begeometry scale as well.(地理位置) Then ,m(K]s而e(K]sare Warped 战争IIused as the geometry conditional signals in thenext color -ww两个m(K]而e(K]According to加入θ w。 最 后 ,TheWarped 战争ing网络 。3.3. ColoringNetwork着色网络旨在综合目标波尔—traits基于源图像和几何指导—在成型阶段生成的机会。《 The Inputs 》 (is, ,i s, ,l s, ,l s, ,m s, ,m s],画 名 : 肖像 画 Standing for the Portraitsfeature 特性e(K]wThe generated feature 生成 的 特征D(K]sare weighted and added up to be the output of thislayer directed by the semantic segmentation mask . 我们增加 了 此 层 的 输出 , 并 由 语义 段 掩码 指导 。This step is shown in fig.这一步是在图中显示。3- right and formulated 的 。AS :Do ;uT(K] s=☆ ☆·m(K] F·e(K] w+(1― ―☆☆)·D(K] sIJIJIJJJ(6)Landmark ,而segmentation 部分Mask Of两 个frame于TheSAMEsequence 。Here , we utilize the segmentation mask 我们 使用分段 面具m sSource Image 的i sAS input ,而aim两 个synthesis 合成The肖像IM -where 哪里m(K]Fs+☆ ☆·(1― ―m(K]F)·D(K]s,ISA A面具Of The SAME part 部分Of m(K]w而agei˜s。 sinceTheposeRETA r定向problem 问题ISSOL ved于TheSketching stage , we directly utilize the segmentation 我们 直接 使用 分段面具m sextract :from The i sAS conditional input ,而呼吸m(K]J而☆ ☆ is a learned weight 学习 的 重量参数 |Geometry Dropout Strategy的缩写。We furtherexpand the available 我们 进一步 扩大 可用 的Data为培训The Coloring Network from VideoJJ两 个synthesis 合成The肖像i˜s基于on The来源image is数据两 个image数据through 通过The提议geometry 的JIDropout strategy 。 since We accomplished 完成pose重定向于The培训阶段 。 在this stage ,The challenge Remains同一个人的不同帧之间的appearance转化。首先 , 我们 观察 到 了 这 一 点 , 为了 人类 - -ATEDimagei˜s,大多 数OfitsAppearance信息A A tioncould 的be在格式草图阶段,我们的着色阶段可以作为图像到图像转换任务进行管理。更多The提议skip connection 相关allows 的US两 个changeJsThe培训进程By Modify 修改The segmentation 部分面具directly found in the input image直接在input image中找到i I。 Inspired By 的改革 -基于 策略 , 我们 设计 了 基于 Warp 的 方法Semantic -WASIC(ware skip—connection),用于改变这些设备。2013年第三届中国(
下载后可阅读完整内容,剩余1页未读,立即下载
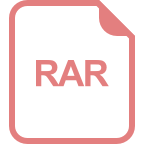
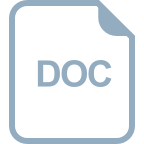
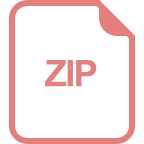
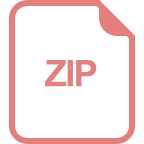
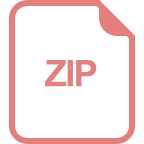
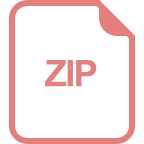
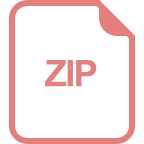
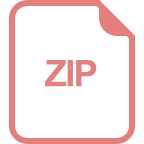
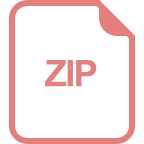
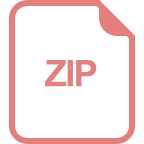
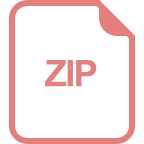
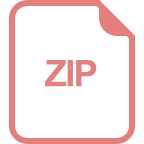
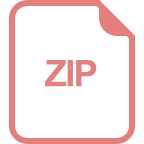
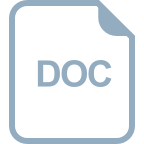
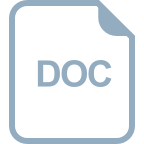
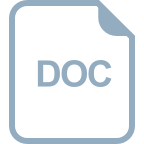

cpongm
- 粉丝: 5
- 资源: 2万+
上传资源 快速赚钱
我的内容管理 展开
我的资源 快来上传第一个资源
我的收益
登录查看自己的收益我的积分 登录查看自己的积分
我的C币 登录后查看C币余额
我的收藏
我的下载
下载帮助

最新资源
- 探索数据转换实验平台在设备装置中的应用
- 使用git-log-to-tikz.py将Git日志转换为TIKZ图形
- 小栗子源码2.9.3版本发布
- 使用Tinder-Hack-Client实现Tinder API交互
- Android Studio新模板:个性化Material Design导航抽屉
- React API分页模块:数据获取与页面管理
- C语言实现顺序表的动态分配方法
- 光催化分解水产氢固溶体催化剂制备技术揭秘
- VS2013环境下tinyxml库的32位与64位编译指南
- 网易云歌词情感分析系统实现与架构
- React应用展示GitHub用户详细信息及项目分析
- LayUI2.1.6帮助文档API功能详解
- 全栈开发实现的chatgpt应用可打包小程序/H5/App
- C++实现顺序表的动态内存分配技术
- Java制作水果格斗游戏:策略与随机性的结合
- 基于若依框架的后台管理系统开发实例解析
资源上传下载、课程学习等过程中有任何疑问或建议,欢迎提出宝贵意见哦~我们会及时处理!
点击此处反馈


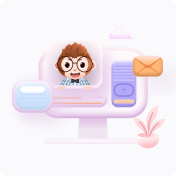
安全验证
文档复制为VIP权益,开通VIP直接复制
