numpy实现图像循环平移
时间: 2023-06-27 09:04:45 浏览: 85
可以使用numpy中的roll函数实现图像的循环平移。具体步骤如下:
1. 将图像转换为numpy数组。
2. 使用numpy的roll函数对图像数组进行平移操作。
3. 将平移后的图像数组转换回图像格式。
以下是代码示例:
```python
import numpy as np
from PIL import Image
# 读取图像
im = Image.open('test.jpg')
im.show()
# 将图像转换为numpy数组
im_arr = np.asarray(im)
# 图像循环平移
shifted_arr = np.roll(im_arr, 100, axis=1)
# 将平移后的数组转换为图像格式
shifted_im = Image.fromarray(shifted_arr)
shifted_im.show()
```
在上面的示例中,我们将图像沿着水平方向平移了100个像素。可以根据需要调整平移的距离和方向。
相关问题
for循环图像持续平移
您可以使用循环来实现图像的持续平移。下面是一个示例代码,演示了如何使用for循环在图像上进行平移:
```python
import cv2
import numpy as np
# 读取图像
img = cv2.imread('image.jpg')
# 设置平移距离和方向
shift_x = 10 # 水平方向平移的像素数
shift_y = 10 # 垂直方向平移的像素数
# 获取图像尺寸
height, width = img.shape[:2]
# 创建一个空白画布,用于存储平移后的图像
shifted_img = np.zeros_like(img)
# 循环遍历图像的每个像素
for y in range(height):
for x in range(width):
# 计算平移后的像素位置
new_x = (x + shift_x) % width
new_y = (y + shift_y) % height
# 将像素从原始图像复制到平移后的图像
shifted_img[new_y, new_x] = img[y, x]
# 显示原始图像和平移后的图像
cv2.imshow('Original Image', img)
cv2.imshow('Shifted Image', shifted_img)
cv2.waitKey(0)
cv2.destroyAllWindows()
```
在这个例子中,我们使用了OpenCV库来读取和显示图像。首先,我们读取了一个名为'image.jpg'的图像。然后,我们定义了一个平移距离(shift_x和shift_y),用于指定水平和垂直方向的像素平移量。然后,我们遍历图像的每个像素,并根据平移距离计算新的像素位置。最后,我们将原始图像中的像素复制到平移后的图像中。最后,我们使用cv2.imshow()函数显示原始图像和平移后的图像。
请注意,这个例子中的平移是循环的,也就是说图像在平移后会从另一侧重新出现。如果您想要实现其他类型的平移效果,可以根据具体需求进行修改。
for循环实现图像持续平移的代码
Sure! Here's an example code snippet to implement continuous image translation using a for loop in Python:
```python
import cv2
import numpy as np
def translate_image(image, shift_x, shift_y):
rows, cols = image.shape[:2]
M = np.float32([[1, 0, shift_x], [0, 1, shift_y]])
translated_image = cv2.warpAffine(image, M, (cols, rows))
return translated_image
# Load the image
image = cv2.imread('input_image.jpg')
# Define the translation amount
shift_x = 10 # shift in the x-direction
shift_y = 20 # shift in the y-direction
# Create a loop for continuous translation
while True:
# Translate the image
translated_image = translate_image(image, shift_x, shift_y)
# Display the translated image
cv2.imshow('Translated Image', translated_image)
# Break the loop if 'q' is pressed
if cv2.waitKey(1) & 0xFF == ord('q'):
break
# Release the video capture and close all windows
cv2.destroyAllWindows()
```
In this code, we use the `cv2` library to read and manipulate the image. The `translate_image` function takes an input image and shifts it by a given amount in the x and y directions using the `warpAffine` function. We then create a loop that continuously translates the image in a while loop until the user presses the 'q' key. The translated image is displayed using `imshow` function. Finally, we release the resources and close all windows when the loop is terminated.
Note that you need to have OpenCV installed (`pip install opencv-python`) and provide the path to your input image.
相关推荐
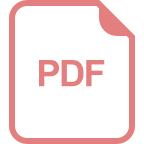
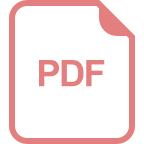
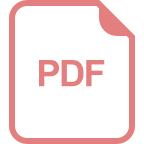










