theta = np.zeros((in_features, 1)) best_theta = np.zeros((in_features, 1)) best_loss = np.inf
时间: 2024-06-02 08:08:36 浏览: 165
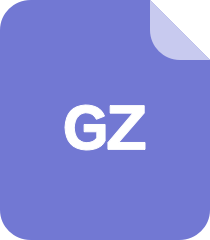
gradient_demo.m.tar.gz_梯度matlab_梯度下降_梯度下降 MATLAB_梯度下降算法_梯度算法
这段代码是在初始化模型参数,其中`in_features`表示输入特征的数量,`theta`是一个形状为`(in_features, 1)`的全零向量,`best_theta`也是一个形状为`(in_features, 1)`的全零向量,`best_loss`是一个初始值为正无穷的标量,用于保存最优模型参数时的损失值。
阅读全文
相关推荐
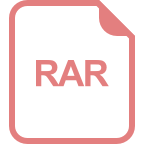
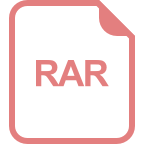
















