function [Solution_matrix]=GaussElimination_Pivot(Augmented_matrix) % 2020-5-18 臻orz % inputs: % Augmented_matrix:增广矩阵,为n*(n+1)维矩阵 % outputs: % Solution_matrix:计算的解,为n1维矩阵 %initialize [row,col]=size(Augmented_matrix); Solution_matrix=zeros(row,1); %判定输入矩阵是否符合要求 if row~=col-1 disp('请输入n(n+1)维矩阵'); else for ii = 1:row-1 %找寻主元 max = ii; for jj = ii+1:row if abs(Augmented_matrix(jj,ii)) > abs(Augmented_matrix(max,ii)) max = jj; end end Augmented_matrix([ii,max],:) = Augmented_matrix([max,ii],:); if Augmented_matrix(ii,ii) == 0 disp(['第',num2str(ii),'个主元素为零']); return; end %开始消元 for jj = ii+1:row Augmented_matrix(jj,:) = Augmented_matrix(jj,:)-... Augmented_matrix(jj,ii)/Augmented_matrix(ii,ii)*Augmented_matrix(ii,:); end end %消元完毕,开始回代 if Augmented_matrix(row,row)==0 disp(['第',num2str(row),'个主元素为零']); return; end Solution_matrix(row)=Augmented_matrix(row,col)/Augmented_matrix(row,col-1); for ii=row-1:-1:1 Solution_matrix(ii)=(Augmented_matrix(ii,col)... -Augmented_matrix(ii,1:row)*Solution_matrix)/Augmented_matrix(ii,ii); end end end请问如何使用这个matlab函数,能否举一个例子
时间: 2024-04-28 10:19:48 浏览: 74
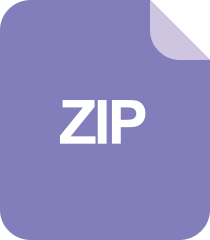
Gauss Jordan 实现:该函数将采用设计为 Gauss-Jordan 算法使用的矩阵并对其进行求解。-matlab开发
这个函数是用来解线性方程组的,输入的增广矩阵是由系数矩阵和常数列构成的。下面是一个例子:
假设有如下线性方程组:
2x + 3y - z = 1
4x - 2y + 3z = -2
-2x + 5y + 2z = 6
则可以将系数矩阵和常数列组成增广矩阵:
Augmented_matrix = [2 3 -1 1; 4 -2 3 -2; -2 5 2 6];
然后调用函数:
Solution_matrix = GaussElimination_Pivot(Augmented_matrix);
最终得到的 Solution_matrix 就是解向量,即 x、y、z 的值。
阅读全文
相关推荐
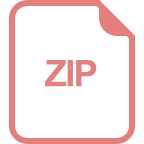
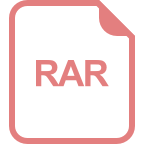
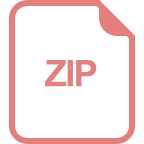
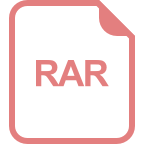
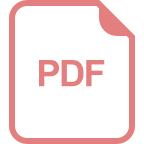
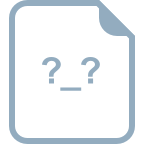
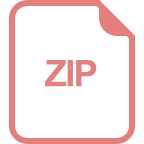
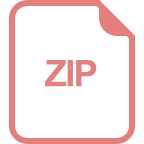
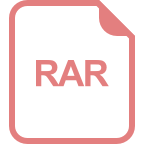
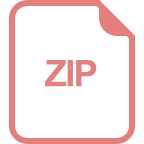
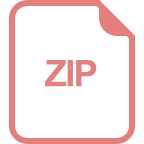
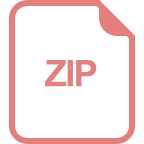
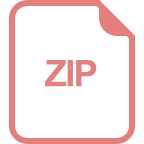
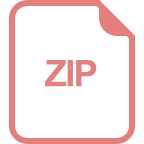
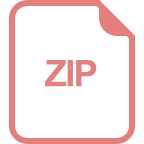