tf.py_function
时间: 2023-12-08 14:03:49 浏览: 28
`tf.py_function` is a TensorFlow operation that allows you to wrap an arbitrary Python function and use it as a TensorFlow operation. It takes a Python function as input and returns a TensorFlow operation that can be called like any other TensorFlow operation in a TensorFlow graph.
This can be useful for situations where you need to perform some operation that is not natively supported by TensorFlow, or when you need to use a third-party library that is not integrated with TensorFlow.
`tf.py_function` takes care of the conversion between TensorFlow tensors and Python objects, allowing you to use Python functions that take and return standard Python types, such as numpy arrays or Python lists. However, it is important to note that using `tf.py_function` can have performance implications, as it involves the overhead of converting data between TensorFlow and Python.
相关推荐
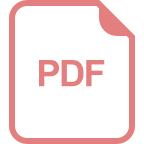
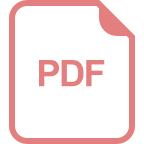
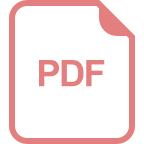















