yolov7训练后生成的result.png中box、Objectness、Classification、Precision、Recall、val BOX、val Objectness、val classification分别代表什么意思
时间: 2024-05-23 20:11:28 浏览: 13
这些指标代表的意思如下:
- Box:预测出的目标框的位置和大小。
- Objectness:目标框内是否有物体的置信度。
- Classification:目标框内物体类别的置信度。
- Precision:精确率,即正确预测为正样本的比例。
- Recall:召回率,即所有正样本中被正确预测为正样本的比例。
- val BOX:验证集中预测出的目标框的位置和大小。
- val Objectness:验证集中目标框内是否有物体的置信度。
- val classification:验证集中目标框内物体类别的置信度。
这些指标可以帮助我们评估模型的性能和训练过程中的进展。其中,Precision 和 Recall 是评估目标检测模型性能的重要指标,可以用来计算 F1 值,综合考虑 Precision 和 Recall 的表现。val BOX、val Objectness 和 val classification 可以用来评估模型在验证集上的性能,从而选择最佳的模型。
相关问题
分析每一行代码,讲述一下这些代码的流程,并且具体的解释每个函数的作用:from sklearn.neural_network import MLPClassifier from sklearn.metrics import classification_report, confusion_matrix import matplotlib BP = MLPClassifier(solver='adam',activation = 'relu',max_iter = 1000,alpha = 1e-3,hidden_layer_sizes = (64,32, 32),random_state = 1) BP.fit(train_X, train_y) y_pred_after = BP.predict(val_X) scores_BP = [] scores_BP.append(precision_score(val_y, y_pred_after)) scores_BP.append(recall_score(val_y, y_pred_after)) confusion_matrix_BP = confusion_matrix(val_y,y_pred_after) f1_score_BP = f1_score(val_y, y_pred_after,labels=None, pos_label=0, average="binary", sample_weight=None) predictions_BP = BP.predict_proba(val_X) # 每一类的概率 FPR_BP, recall_BP, thresholds = roc_curve(val_y, predictions_log[:,1],pos_label=1) area_BP = auc(FPR_BP,recall_BP) print(area_BP) print('BP模型结果:\n') print(pd.DataFrame(columns=['预测值=1','预测值=0'],index=['真实值=1','真实值=0'],data=confusion_matrix_XGB_after))#混淆矩阵 print("f1值:"+str(f1_score_BP)) print("精确度和召回率:"+str(scores_BP))
这段代码的主要流程是,首先从Scikit-learn库中导入所需的模块和函数,包括多层感知机分类器(MLPClassifier)和评价模型性能的指标(classification_report和confusion_matrix),以及绘制图形的模块matplotlib。然后,创建一个MLP分类器BP,并使用train_X和train_y训练数据来训练模型。接着,使用val_X数据集对模型性能进行评估,计算预测值y_pred_after以及各种分类指标的值,例如准确率、召回率、混淆矩阵和F1得分。最后,使用predict_proba函数预测概率值作为BP分类器的输出。
具体来说,MLP分类器BP的参数包括:
solver:用于优化权重的算法,这里使用Adam算法(一种随机梯度下降优化算法),默认为adam。
activation:激活函数的类型,这里使用ReLU(修正线性单元),默认为relu。
max_iter:最大迭代次数,这里设置为1000。
alpha:L2惩罚项的系数,用于控制模型复杂度和过拟合问题。
hidden_layer_sizes:元组类型,每个元素表示每层神经元的数量,这里设置为(64,32,32),表示三个隐藏层分别有64个、32个和32个神经元。
random_state:随机数发生器的种子值,用于确保结果的可重复性。
此外,还需对数据进行预处理和特征提取等操作,这里未给出具体代码。
以上是对本段代码的简单分析和解释。
Task: - Download the UCM dataset from the official source or any reputable repository. - Preprocess the dataset by splitting it into training, validation, and test sets. Please use an 80-10-10 split, respectively. - Choose a suitable deep-learning algorithm for scene classification. You can use popular classifiers such as Convolutional Neural Networks (CNNs). - Implement the chosen algorithm in Python, using appropriate libraries. - Train the classifier using the training set and tune the hyperparameters using the validation set. - Evaluate the trained classifier on the test set using the following metrics: Accuracy: The overall classification accuracy. Precision: The ability to correctly classify positive samples. Recall: The ability to correctly identify positive samples. F1-score: The harmonic mean of precision and recall. Confusion Matrix: A table showing the number of correct and incorrect predictions for each class. Report: Prepare a one-page report summarizing the results of your scene classification experiment and the architecture of your network. Submit your Python code along with the one-page report summarizing the results. Make sure to organize your code and report in a clear and readable manner.
Sure, here's an outline of the steps you'll need to take to complete this task:
1. Download the UCM dataset from the official source or any reputable repository.
2. Preprocess the dataset by splitting it into training, validation, and test sets. Use an 80-10-10 split, respectively. You can use a library like scikit-learn to do this.
3. Choose a suitable deep-learning algorithm for scene classification. Convolutional Neural Networks (CNNs) are a popular choice for image classification tasks like this.
4. Implement the chosen algorithm in Python, using appropriate libraries such as TensorFlow or PyTorch.
5. Train the classifier using the training set and tune the hyperparameters using the validation set.
6. Evaluate the trained classifier on the test set using the following metrics: accuracy, precision, recall, F1-score, and confusion matrix. You can use libraries like scikit-learn to compute these metrics.
7. Prepare a one-page report summarizing the results of your scene classification experiment and the architecture of your network. Include any relevant information such as which hyperparameters you tuned and which ones you used for the final model.
Here's some sample code to get you started:
```
# Step 1: Download UCM dataset
# TODO: Download dataset and extract files
# Step 2: Preprocess dataset
from sklearn.model_selection import train_test_split
# TODO: Load dataset into memory
X_train_val, X_test, y_train_val, y_test = train_test_split(X, y, test_size=0.1, random_state=42)
X_train, X_val, y_train, y_val = train_test_split(X_train_val, y_train_val, test_size=0.1, random_state=42)
# Step 3: Choose deep-learning algorithm
import tensorflow as tf
from tensorflow.keras.layers import Conv2D, MaxPooling2D, Flatten, Dense
model = tf.keras.Sequential([
Conv2D(32, (3, 3), activation='relu', input_shape=(256, 256, 3)),
MaxPooling2D((2, 2)),
Conv2D(64, (3, 3), activation='relu'),
MaxPooling2D((2, 2)),
Conv2D(128, (3, 3), activation='relu'),
MaxPooling2D((2, 2)),
Conv2D(256, (3, 3), activation='relu'),
MaxPooling2D((2, 2)),
Flatten(),
Dense(256, activation='relu'),
Dense(21, activation='softmax')
])
# Step 4: Implement algorithm in Python
model.compile(optimizer='adam',
loss='categorical_crossentropy',
metrics=['accuracy'])
# Step 5: Train classifier
history = model.fit(X_train, y_train, epochs=10, validation_data=(X_val, y_val))
# Step 6: Evaluate trained classifier
from sklearn.metrics import accuracy_score, precision_score, recall_score, f1_score, confusion_matrix
y_pred = model.predict(X_test)
y_pred_classes = np.argmax(y_pred, axis=1)
y_test_classes = np.argmax(y_test, axis=1)
accuracy = accuracy_score(y_test_classes, y_pred_classes)
precision = precision_score(y_test_classes, y_pred_classes, average='macro')
recall = recall_score(y_test_classes, y_pred_classes, average='macro')
f1 = f1_score(y_test_classes, y_pred_classes, average='macro')
confusion_mat = confusion_matrix(y_test_classes, y_pred_classes)
print("Accuracy:", accuracy)
print("Precision:", precision)
print("Recall:", recall)
print("F1-score:", f1)
print("Confusion matrix:\n", confusion_mat)
# Step 7: Prepare report
# TODO: Write report summarizing results and network architecture
```
相关推荐









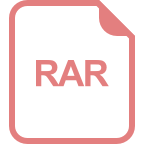