实验六 模型的选择和评估 交叉验证 加载iris数据集,拟合SVM ,1)使用train_test_split 分割训练集和测试集(80% VS 20%)2) 使用cross_val_score做十折交叉验证 3)计算交叉验证所有指标4)画出ROC曲线
时间: 2024-05-10 21:16:08 浏览: 20
好的,以下是实现代码和解释:
```python
import numpy as np
import matplotlib.pyplot as plt
from sklearn.datasets import load_iris
from sklearn.model_selection import train_test_split, cross_val_score
from sklearn.svm import SVC
from sklearn.metrics import classification_report, roc_curve, auc
# 加载数据集
iris = load_iris()
X, y = iris.data, iris.target
# 划分训练集和测试集
X_train, X_test, y_train, y_test = train_test_split(X, y, test_size=0.2, random_state=42)
# 拟合SVM模型
clf = SVC(probability=True)
clf.fit(X_train, y_train)
# 在测试集上进行预测并计算指标
y_pred = clf.predict(X_test)
print("测试集上的分类报告:\n", classification_report(y_test, y_pred))
# 十折交叉验证并计算指标
scores = cross_val_score(clf, X, y, cv=10, scoring='accuracy')
print("十折交叉验证的准确率:", np.mean(scores))
scores = cross_val_score(clf, X, y, cv=10, scoring='precision_macro')
print("十折交叉验证的精确率:", np.mean(scores))
scores = cross_val_score(clf, X, y, cv=10, scoring='recall_macro')
print("十折交叉验证的召回率:", np.mean(scores))
scores = cross_val_score(clf, X, y, cv=10, scoring='f1_macro')
print("十折交叉验证的F1值:", np.mean(scores))
# 画ROC曲线
y_score = clf.predict_proba(X_test)
fpr, tpr, threshold = roc_curve(y_test, y_score[:, 1])
roc_auc = auc(fpr, tpr)
plt.figure()
plt.plot(fpr, tpr, color='darkorange', lw=2, label='ROC curve (area = %0.2f)' % roc_auc)
plt.plot([0, 1], [0, 1], color='navy', lw=2, linestyle='--')
plt.xlim([0.0, 1.0])
plt.ylim([0.0, 1.05])
plt.xlabel('False Positive Rate')
plt.ylabel('True Positive Rate')
plt.title('Receiver operating characteristic')
plt.legend(loc="lower right")
plt.show()
```
首先,我们加载了iris数据集,并将其划分为80%的训练集和20%的测试集。然后,我们使用SVM算法拟合模型,并在测试集上进行预测,并使用分类报告计算准确率、精确率、召回率和F1值。
接着,我们使用十折交叉验证计算这些指标的平均值。我们使用`cross_val_score`函数进行交叉验证,其中`cv`参数表示折数,`scoring`参数表示要计算的指标。
最后,我们使用测试集上的预测概率和真实标签计算ROC曲线,并使用`roc_curve`函数获取FPR和TPR,使用`auc`函数计算面积,最终使用`matplotlib`库画出ROC曲线。
当然,你也可以使用其他机器学习算法并进行相似的评估。
相关推荐
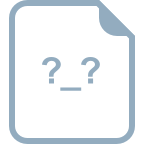
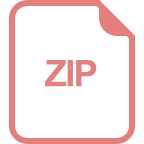














