for i = 1: num_of_image
时间: 2024-03-30 08:38:32 浏览: 23
这个代码段是一个 for 循环语句,用于遍历指定范围内的整数变量 i。具体来说,for i = 1: num_of_image 表示从 1 到 num_of_image 依次遍历整数变量 i。在循环体内,可以对 i 进行操作或使用 i 进行计算。当 i 遍历到 num_of_image 时,循环结束。这个代码段的作用是对指定范围内的整数变量进行遍历,以便对每个整数进行相应的操作或计算。
相关问题
for i = 1: num_of_image image = imread(horzcat(pathname,sub_Name{i})); if size(image,3) >1 image = rgb2gray(image); end
这是一段代码,用于读取指定路径下的图像文件,并将其转换为灰度图像。
- num_of_image是一个标量,表示指定路径下的图像文件数量。
- 循环变量i从1到num_of_image进行遍历。
- 在每次循环中,使用imread函数读取指定路径下的第i个图像文件,并将其存储在image变量中。
- 如果图像是RGB格式(即size(image,3)返回值大于1),则使用rgb2gray函数将其转换为灰度图像。
最终,循环结束后,我们将会得到一个大小为num_of_image的图像集合,其中每个图像都是灰度图像。
for cnt=1 :num_of_char % for 循环读取所有文件夹 pathname=horzcat(DATADIR, Name{cnt},'\'); % 把路径和名字融合一起 sub_dirinfo=dir(pathname); % 获取图像目录所有文件信息 sub_Name={sub_dirinfo.name}; % 获取文件名 sub_Name(1:2)=[]; [nouse num_of_image]=size(sub_Name); for i = 1: num_of_image image = imread(horzcat(pathname,sub_Name{i})); if size(image,3) >1 image = rgb2gray(image); end bw = im2bw(image,graythresh(image)); bw1 = double(reshape(bw,288,1)); images = [images,bw1]; labels(count) = cnt; count = count +1; end end
这是一段MATLAB代码,它用于从指定的文件夹中读取图像数据,并将其转换为可用于机器学习算法的格式。具体来说,该代码中的DATADIR是指定图像数据所在的文件夹路径,Name是一个包含所有图像类别名称的向量,num_of_char表示总共有多少个图像类别。在for循环中,首先使用horzcat函数将DATADIR和当前图像类别的名称拼接成完整的文件夹路径pathname。接下来,使用dir函数获取pathname目录下的所有文件信息,并将文件名存储在sub_Name向量中。在for循环的内部,再次使用horzcat函数将pathname和当前图像文件名拼接成完整的文件路径,并使用imread函数读取图像数据。如果图像是彩色图像,则使用rgb2gray函数将其转换为灰度图像。接下来使用im2bw函数将灰度图像二值化,并将其转换为一个288*1的向量bw1。最后,将bw1添加到images矩阵中,并将当前图像类别的编号存储在labels向量中。需要注意的是,该代码中的reshape函数用于将288*1的向量bw1转换为一个288*1的矩阵,以便与其他图像数据形成一个矩阵。
相关推荐
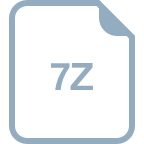
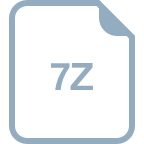
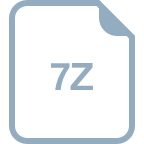













