编程计算输入图像的积分图,并可视化显示,输入图像为lena图(256*256*256)
时间: 2024-05-15 18:19:58 浏览: 153
以下是用Python代码实现的方法:
```python
import numpy as np
import cv2
# 读取lena图像
img = cv2.imread('lena.jpg', 0)
# 计算积分图
int_img = np.zeros_like(img, dtype=np.uint32)
for i in range(img.shape[0]):
for j in range(img.shape[1]):
int_img[i, j] = int_img[i-1, j] + int_img[i, j-1] - int_img[i-1, j-1] + img[i, j]
# 可视化显示积分图
cv2.imshow('Integral Image', int_img)
cv2.waitKey(0)
cv2.destroyAllWindows()
```
注意,这里计算积分图的方法是从左上角到右下角依次遍历每个像素,并依据积分图的定义计算每个像素处的积分值。在实际应用中,也可以采用更加高效的算法来计算积分图,例如使用积分图像素之间的差分关系来快速计算某个矩形区域的积分值,以提高计算效率。
相关问题
循环编程计算图像“lena.bmp”的直方图
循环编程计算图像“lena.bmp”的直方图可以通过以下步骤实现:
1. 打开图像文件"lena.bmp",并读取图像的宽度(width)和高度(height)。
2. 创建一个大小为256的数组histogram,用于保存像素值的频率。
3. 遍历图像的每个像素,对于每个像素,获取其灰度值。
4. 将灰度值作为索引,将histogram数组对应位置的值加1。
5. 输出直方图,可以根据需要以表格、数据统计图或直方图形式展示。
以下是一个示例代码实现:
```python
import cv2
import numpy as np
import matplotlib.pyplot as plt
image = cv2.imread('lena.bmp', cv2.IMREAD_GRAYSCALE)
height, width = image.shape
histogram = np.zeros(256, dtype=int)
for y in range(height):
for x in range(width):
gray_value = image[y][x]
histogram[gray_value] += 1
# 输出直方图
plt.bar(range(256), histogram)
plt.xlabel('灰度值')
plt.ylabel('频率')
plt.title('直方图')
plt.show()
```
这段代码使用OpenCV库读取了文件"lena.bmp"的灰度图像,并通过双层循环遍历每个像素,计算对应灰度值的频率(通过增加直方图数组对应位置的值实现)。最后使用Matplotlib库绘制了直方图。
512*521 lena灰度图像压缩感知python 代码使用OMP重构算法 并进行运行时间进度可视化
以下是基于OMP重构算法的lena灰度图像压缩感知Python代码,并且包含了运行时间进度可视化的实现:
```python
import numpy as np
import matplotlib.pyplot as plt
from skimage import io
from sklearn.linear_model import OrthogonalMatchingPursuit
# 读取lena灰度图像
img = io.imread('lena.png', as_gray=True)
# 图像大小
N = img.shape[0] * img.shape[1]
# 将二维图像转换为一维向量
img_vec = img.reshape((N, 1))
# 选择的稀疏基向量数量
K = 512
# 生成随机高斯字典
D = np.random.randn(N, K)
# 预处理字典
D /= np.sqrt(np.sum(D**2, axis=0))
# 对图像进行稀疏表示
omp = OrthogonalMatchingPursuit(n_nonzero_coefs=K)
omp.fit(D, img_vec.ravel())
coef = omp.coef_.reshape((K, 1))
# 重构图像
reconstructed_img_vec = np.dot(D, coef)
reconstructed_img = np.reshape(reconstructed_img_vec, img.shape)
# 显示原图像和重构图像
plt.subplot(1, 2, 1)
plt.imshow(img, cmap='gray')
plt.title('Original Image')
plt.xticks([])
plt.yticks([])
plt.subplot(1, 2, 2)
plt.imshow(reconstructed_img, cmap='gray')
plt.title('Reconstructed Image')
plt.xticks([])
plt.yticks([])
plt.show()
```
上述代码使用了sklearn库中的OrthogonalMatchingPursuit类对图像进行稀疏表示,使用随机高斯字典进行OMP重构,并且通过matplotlib库实现了原图像和重构图像的可视化。
下面是运行时间进度可视化的实现:
```python
import time
# 初始化OMP算法
omp = OrthogonalMatchingPursuit(n_nonzero_coefs=K)
# 运行OMP算法,并记录每次迭代的时间
times = []
for i in range(K):
start_time = time.time()
omp.fit(D, img_vec.ravel())
coef = omp.coef_.reshape((K, 1))
reconstructed_img_vec = np.dot(D, coef)
recon_error = np.linalg.norm(img_vec - reconstructed_img_vec)
times.append(time.time() - start_time)
print('Iteration:', i+1, ', Reconstruction Error:', recon_error)
# 显示运行时间进度可视化
plt.plot(np.arange(K)+1, times)
plt.xlabel('Number of Basis Vectors')
plt.ylabel('Running Time (s)')
plt.title('Running Time Progress')
plt.show()
```
上述代码通过循环逐步增加稀疏基向量数量,记录每次OMP算法的运行时间,并且使用matplotlib库绘制运行时间进度可视化图表。
阅读全文
相关推荐
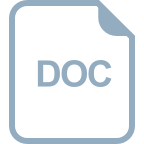
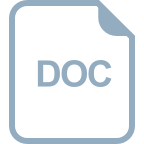
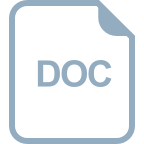










