pytorch写一个vit
时间: 2023-05-14 08:04:44 浏览: 161
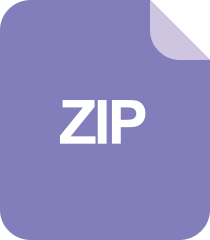
ViT:实现Vi(sion)T(transformer)
非常感谢您的提问。以下是使用 PyTorch 实现 ViT 的代码示例:
```python
import torch
import torch.nn as nn
import torch.nn.functional as F
class PatchEmbedding(nn.Module):
def __init__(self, img_size=224, patch_size=16, in_chans=3, embed_dim=768):
super().__init__()
img_size = (img_size, img_size) if isinstance(img_size, int) else img_size
patch_size = (patch_size, patch_size) if isinstance(patch_size, int) else patch_size
num_patches = (img_size[1] // patch_size[1]) * (img_size[0] // patch_size[0])
self.img_size = img_size
self.patch_size = patch_size
self.num_patches = num_patches
self.projection = nn.Conv2d(in_chans, embed_dim, kernel_size=patch_size, stride=patch_size)
def forward(self, x):
B, C, H, W = x.shape
assert H == self.img_size[0] and W == self.img_size[1], \
f"Input image size ({H}*{W}) doesn't match model ({self.img_size[0]}*{self.img_size[1]})."
x = self.projection(x).flatten(2).transpose(1, 2)
return x
class MLP(nn.Module):
def __init__(self, in_features, hidden_features=None, out_features=None, dropout=0.):
super().__init__()
out_features = out_features or in_features
hidden_features = hidden_features or in_features
self.fc1 = nn.Linear(in_features, hidden_features)
self.act = nn.GELU()
self.fc2 = nn.Linear(hidden_features, out_features)
self.dropout = nn.Dropout(dropout)
def forward(self, x):
x = self.fc1(x)
x = self.act(x)
x = self.dropout(x)
x = self.fc2(x)
x = self.dropout(x)
return x
class Attention(nn.Module):
def __init__(self, dim, num_heads=8, qkv_bias=False, attn_drop=0., proj_drop=0.):
super().__init__()
self.num_heads = num_heads
head_dim = dim // num_heads
self.scale = head_dim ** -0.5
self.qkv = nn.Linear(dim, dim * 3, bias=qkv_bias)
self.attn_drop = nn.Dropout(attn_drop)
self.proj = nn.Linear(dim, dim)
self.proj_drop = nn.Dropout(proj_drop)
def forward(self, x):
B, N, C = x.shape
qkv = self.qkv(x).reshape(B, N, 3, self.num_heads, C // self.num_heads).permute(2, 0, 3, 1, 4)
q, k, v = qkv[0], qkv[1], qkv[2]
attn = (q @ k.transpose(-2, -1)) * self.scale
attn = attn.softmax(dim=-1)
attn = self.attn_drop(attn)
x = (attn @ v).transpose(1, 2).reshape(B, N, C)
x = self.proj(x)
x = self.proj_drop(x)
return x
class Block(nn.Module):
def __init__(self, dim, num_heads, mlp_ratio=4., qkv_bias=False, drop=0., attn_drop=0., drop_path=0.):
super().__init__()
self.norm1 = nn.LayerNorm(dim)
self.attn = Attention(dim, num_heads=num_heads, qkv_bias=qkv_bias, attn_drop=attn_drop, proj_drop=drop)
self.drop_path = nn.Dropout(drop_path) if drop_path > 0. else nn.Identity()
self.norm2 = nn.LayerNorm(dim)
mlp_hidden_dim = int(dim * mlp_ratio)
self.mlp = MLP(in_features=dim, hidden_features=mlp_hidden_dim, out_features=dim, dropout=drop)
def forward(self, x):
x = x + self.drop_path(self.attn(self.norm1(x)))
x = x + self.drop_path(self.mlp(self.norm2(x)))
return x
class VisionTransformer(nn.Module):
def __init__(self, img_size=224, patch_size=16, in_chans=3, num_classes=1000,
embed_dim=768, depth=12, num_heads=12, mlp_ratio=4., qkv_bias=True,
drop_rate=0., attn_drop_rate=0., drop_path_rate=0.):
super().__init__()
self.num_classes = num_classes
self.num_features = self.embed_dim = embed_dim
self.patch_embed = PatchEmbedding(img_size=img_size, patch_size=patch_size, in_chans=in_chans, embed_dim=embed_dim)
num_patches = self.patch_embed.num_patches
self.cls_token = nn.Parameter(torch.zeros(1, 1, embed_dim))
self.pos_embed = nn.Parameter(torch.zeros(1, num_patches + 1, embed_dim))
self.pos_drop = nn.Dropout(p=drop_rate)
dpr = [x.item() for x in torch.linspace(0, drop_path_rate, depth)] # stochastic depth decay rule
self.blocks = nn.ModuleList([
Block(
dim=embed_dim, num_heads=num_heads, mlp_ratio=mlp_ratio, qkv_bias=qkv_bias,
drop=drop_rate, attn_drop=attn_drop_rate, drop_path=dpr[i])
for i in range(depth)])
self.norm = nn.LayerNorm(embed_dim)
self.head = nn.Linear(embed_dim, num_classes) if num_classes > 0 else nn.Identity()
def forward(self, x):
x = self.patch_embed(x)
cls_token = self.cls_token.expand(x.shape[0], -1, -1)
x = torch.cat((cls_token, x), dim=1)
x = x + self.pos_embed
x = self.pos_drop(x)
for blk in self.blocks:
x = blk(x)
x = self.norm(x[:, 0])
x = self.head(x)
return x
```
希望这个代码示例能够帮助您实现 ViT。如果您有任何问题,请随时问我。
阅读全文
相关推荐
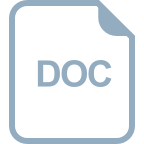
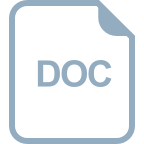
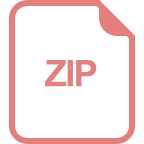
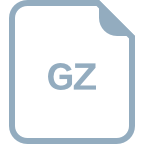










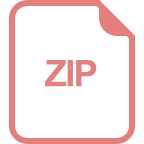

