Weakly Supervised Semantic Segmentation
时间: 2023-09-02 13:06:40 浏览: 63
弱监督语义分割是一种语义分割任务的训练方法,其中标注数据的标签信息相对不完整或不精确。通常,标准的语义分割需要每个像素的精确标签,而弱监督语义分割允许使用更高级别的标签信息来训练模型。
在弱监督语义分割中,常见的方法之一是使用图像级别的标签来训练模型。这意味着我们只有整个图像的标签,而没有每个像素的精确标注。一种常见的方法是使用图像级别的标签来生成像素级别的伪标签,然后使用这些伪标签进行训练。这可以通过使用预训练的图像分类模型来实现,将其应用于输入图像,然后根据分类结果生成伪标签。
另一种方法是利用辅助信息来进行弱监督分割。例如,可以使用辅助标注工具来标记一些关键对象或区域,并将其作为辅助信息来训练模型。这种方法可以在模型训练过程中使用辅助信息来引导分割结果的学习。
还有其他一些方法,如使用部分标注数据、多示例学习等,都可以用于弱监督语义分割。这些方法的目标是在缺乏精确标签的情况下,利用可用的标签信息进行有效的模型训练和语义分割任务。
请注意,这些方法都是为了在缺乏精确标注的情况下进行语义分割训练,但由于标签信息的不完整性或不精确性,可能会对分割结果的准确性产生一定的影响。
相关问题
Regional Semantic Contrast and Aggregation for Weakly Supervised Semantic Segmentation
Weakly supervised semantic segmentation is a challenging task where only image-level labels are available for training. In this paper, we propose a novel approach that utilizes regional semantic contrast and aggregation to improve the performance of weakly supervised semantic segmentation.
First, we introduce a regional semantic contrast loss that encourages the model to focus on regions with high semantic contrast, i.e., regions where the difference between the foreground and background classes is high. Specifically, we compute the semantic contrast map by subtracting the predicted probability of the background class from the predicted probability of the foreground class, and then apply a Gaussian filter to smooth the map.
Second, we propose a regional aggregation module that aggregates the features of each region to obtain a more representative feature representation. The module consists of a region proposal network that generates candidate regions based on the semantic contrast map, and a region-based pooling layer that pools the features within each region.
Finally, we combine the regional semantic contrast loss and the regional aggregation module with a standard cross-entropy loss to train the model. Experimental results on the PASCAL VOC 2012 dataset demonstrate that our approach achieves state-of-the-art performance among weakly supervised methods, and even outperforms some fully supervised methods.
railroad is not a train: saliency as pseudo-pixel supervision for weakly supervised semantic segmentation
这篇论文的主要内容是介绍了一种新的弱监督语义分割方法,即使用伪像素监督训练模型。其中,论文拿“railroad is not a train”的例子说明了弱监督下的语义分割问题,给出了具体的解决方法,并取得了较好的效果。
相关推荐
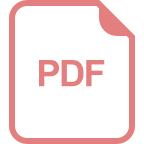
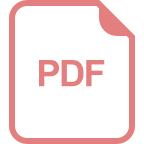
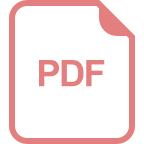












