选择一个神经网络模型,实现MNIST手写体识别数据库的训练与测试。开发环境为python
时间: 2024-03-23 21:38:51 浏览: 9
好的,我建议使用PyTorch框架来实现MNIST手写数字识别。下面是一个简单的代码示例:
首先,需要导入必要的库和模块:
```python
import torch
import torch.nn as nn
import torch.optim as optim
from torchvision import datasets, transforms
```
然后,定义一个神经网络模型。在这个示例中,我们使用两个卷积层和两个全连接层来构建一个简单的卷积神经网络:
```python
class Net(nn.Module):
def __init__(self):
super(Net, self).__init__()
self.conv1 = nn.Conv2d(1, 10, kernel_size=5)
self.conv2 = nn.Conv2d(10, 20, kernel_size=5)
self.fc1 = nn.Linear(320, 50)
self.fc2 = nn.Linear(50, 10)
def forward(self, x):
x = nn.functional.relu(nn.functional.max_pool2d(self.conv1(x), 2))
x = nn.functional.relu(nn.functional.max_pool2d(self.conv2(x), 2))
x = x.view(-1, 320)
x = nn.functional.relu(self.fc1(x))
x = self.fc2(x)
return nn.functional.log_softmax(x, dim=1)
```
接下来,定义训练和测试函数:
```python
def train(model, device, train_loader, optimizer, epoch):
model.train()
for batch_idx, (data, target) in enumerate(train_loader):
data, target = data.to(device), target.to(device)
optimizer.zero_grad()
output = model(data)
loss = nn.functional.nll_loss(output, target)
loss.backward()
optimizer.step()
def test(model, device, test_loader):
model.eval()
test_loss = 0
correct = 0
with torch.no_grad():
for data, target in test_loader:
data, target = data.to(device), target.to(device)
output = model(data)
test_loss += nn.functional.nll_loss(output, target, reduction='sum').item()
pred = output.argmax(dim=1, keepdim=True)
correct += pred.eq(target.view_as(pred)).sum().item()
test_loss /= len(test_loader.dataset)
print('\nTest set: Average loss: {:.4f}, Accuracy: {}/{} ({:.0f}%)\n'.format(
test_loss, correct, len(test_loader.dataset),
100. * correct / len(test_loader.dataset)))
```
最后,加载数据集并开始训练和测试:
```python
batch_size = 64
test_batch_size = 1000
epochs = 10
learning_rate = 0.01
device = torch.device("cuda" if torch.cuda.is_available() else "cpu")
train_loader = torch.utils.data.DataLoader(
datasets.MNIST('data', train=True, download=True,
transform=transforms.Compose([
transforms.ToTensor(),
transforms.Normalize((0.1307,), (0.3081,))
])),
batch_size=batch_size, shuffle=True)
test_loader = torch.utils.data.DataLoader(
datasets.MNIST('data', train=False, transform=transforms.Compose([
transforms.ToTensor(),
transforms.Normalize((0.1307,), (0.3081,))
])),
batch_size=test_batch_size, shuffle=True)
model = Net().to(device)
optimizer = optim.SGD(model.parameters(), lr=learning_rate)
for epoch in range(1, epochs + 1):
train(model, device, train_loader, optimizer, epoch)
test(model, device, test_loader)
```
这里我们使用了SGD优化器和交叉熵损失函数。通过运行上面的代码,你应该可以得到一个大约98%的测试准确率。
相关推荐
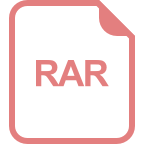
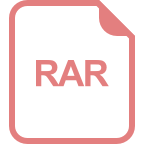
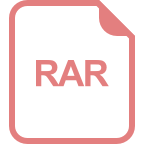
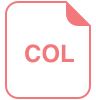













