高斯过程回归预测模型python
时间: 2024-08-20 18:03:16 浏览: 57
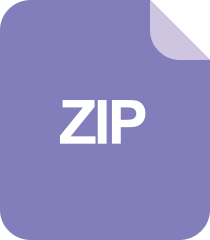
GPR.zip_Gpr python_高斯过程_高斯过程回归

高斯过程回归(Gaussian Process Regression, GPR)是一种基于概率的机器学习方法,用于处理非线性和非参数的预测问题。在Python中,你可以使用Scikit-learn库中的`GaussianProcessRegressor`类来实现。以下是一个简单的步骤:
1. 导入必要的库:
```python
from sklearn.gaussian_process import GaussianProcessRegressor
from sklearn.gaussian_process.kernels import RBF, WhiteKernel
import numpy as np
```
2. 准备数据集(X是输入特征,y是目标值):
```python
X = np.random.rand(100, 2) # 100个样本,每个样本有两个特征
y = np.sin(X).sum(axis=1) + 0.1 * np.random.randn(len(X)) # 目标值为正弦函数加上噪声
```
3. 创建并训练高斯过程回归模型:
```python
kernel = RBF(length_scale=1.0, length_scale_bounds=(1e-2, 1e3)) + WhiteKernel(noise_level=1e-10)
gp = GaussianProcessRegressor(kernel=kernel)
gp.fit(X, y)
```
4. 进行预测:
```python
X_new = np.linspace(0, 1, 1000).reshape(-1, 2)
y_pred, sigma = gp.predict(X_new, return_std=True)
```
阅读全文
相关推荐
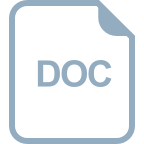
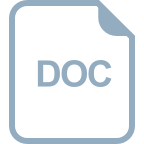










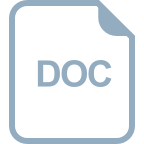
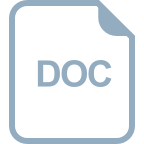
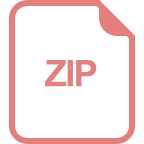
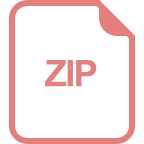
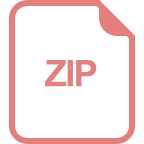
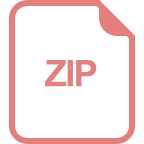