acc = (logits.argmax(dim=-1) == labels).float().mean()什么意思
时间: 2024-05-29 15:11:34 浏览: 157
这行代码计算了模型的准确率(accuracy),即预测正确的样本数占总样本数的比例。具体来说,它首先通过 `logits.argmax(dim=-1)` 获取模型在每个样本上预测的类别(即概率最大的类别),然后与标签进行比较,得到一个布尔值张量,其中预测正确的位置为 True,错误的位置为 False。最后,将这个张量转化为浮点数并取平均值,即可得到准确率。
相关问题
Structure-Aware Transformer for Graph Representation Learning 论文代码的 dgl实现
"Structure-Aware Transformer for Graph Representation Learning"是一篇使用Transformer模型进行图表示学习的论文。这篇论文提出了一种名为SAT(Structure-Aware Transformer)的模型,它利用了图中节点之间的结构信息,以及节点自身的特征信息。SAT模型在多个图数据集上都取得了非常好的结果。
以下是SAT模型的dgl实现代码,代码中使用了Cora数据集进行示例:
```
import dgl
import numpy as np
import torch
import torch.nn as nn
import torch.nn.functional as F
class GraphAttentionLayer(nn.Module):
def __init__(self, in_dim, out_dim, num_heads):
super(GraphAttentionLayer, self).__init__()
self.num_heads = num_heads
self.out_dim = out_dim
self.W = nn.Linear(in_dim, out_dim*num_heads, bias=False)
nn.init.xavier_uniform_(self.W.weight)
self.a = nn.Parameter(torch.zeros(size=(2*out_dim, 1)))
nn.init.xavier_uniform_(self.a.data)
def forward(self, g, h):
h = self.W(h).view(-1, self.num_heads, self.out_dim)
# Compute attention scores
with g.local_scope():
g.ndata['h'] = h
g.apply_edges(fn.u_dot_v('h', 'h', 'e'))
e = F.leaky_relu(g.edata.pop('e'), negative_slope=0.2)
g.edata['a'] = torch.cat([e, e], dim=1)
g.edata['a'] = torch.matmul(g.edata['a'], self.a).squeeze()
g.edata['a'] = F.leaky_relu(g.edata['a'], negative_slope=0.2)
g.apply_edges(fn.e_softmax('a', 'w'))
# Compute output features
g.ndata['h'] = h
g.update_all(fn.u_mul_e('h', 'w', 'm'), fn.sum('m', 'h'))
h = g.ndata['h']
return h.view(-1, self.num_heads*self.out_dim)
class SATLayer(nn.Module):
def __init__(self, in_dim, out_dim, num_heads):
super(SATLayer, self).__init__()
self.attention = GraphAttentionLayer(in_dim, out_dim, num_heads)
self.dropout = nn.Dropout(0.5)
self.norm = nn.LayerNorm(out_dim*num_heads)
def forward(self, g, h):
h = self.attention(g, h)
h = self.norm(h)
h = F.relu(h)
h = self.dropout(h)
return h
class SAT(nn.Module):
def __init__(self, in_dim, hidden_dim, out_dim, num_heads):
super(SAT, self).__init__()
self.layer1 = SATLayer(in_dim, hidden_dim, num_heads)
self.layer2 = SATLayer(hidden_dim*num_heads, out_dim, 1)
def forward(self, g, h):
h = self.layer1(g, h)
h = self.layer2(g, h)
return h.mean(0)
# Load Cora dataset
from dgl.data import citation_graph as citegrh
data = citegrh.load_cora()
g = data.graph
features = torch.FloatTensor(data.features)
labels = torch.LongTensor(data.labels)
train_mask = torch.BoolTensor(data.train_mask)
val_mask = torch.BoolTensor(data.val_mask)
test_mask = torch.BoolTensor(data.test_mask)
# Add self loop
g = dgl.remove_self_loop(g)
g = dgl.add_self_loop(g)
# Define model and optimizer
model = SAT(features.shape[1], 64, data.num_classes, 8)
optimizer = torch.optim.Adam(model.parameters(), lr=0.005, weight_decay=5e-4)
# Train model
for epoch in range(200):
model.train()
logits = model(g, features)
loss = F.cross_entropy(logits[train_mask], labels[train_mask])
optimizer.zero_grad()
loss.backward()
optimizer.step()
acc = (logits[val_mask].argmax(1) == labels[val_mask]).float().mean()
if epoch % 10 == 0:
print('Epoch {:03d} | Loss {:.4f} | Accuracy {:.4f}'.format(epoch, loss.item(), acc.item()))
# Test model
model.eval()
logits = model(g, features)
acc = (logits[test_mask].argmax(1) == labels[test_mask]).float().mean()
print('Test accuracy {:.4f}'.format(acc.item()))
```
在这个示例中,我们首先加载了Cora数据集,并将其转换为一个DGL图。然后,我们定义了一个包含两个SAT层的模型,以及Adam优化器。在训练过程中,我们使用交叉熵损失函数和验证集上的准确率来监控模型的性能。在测试阶段,我们计算测试集上的准确率。
阅读全文
相关推荐
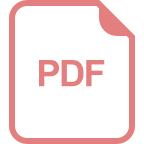
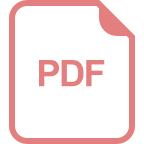
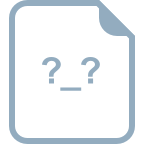
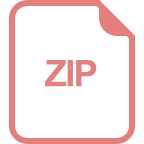
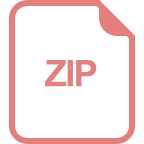
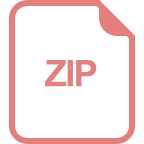
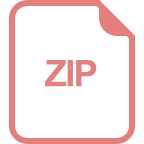
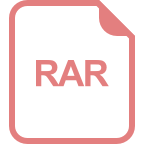
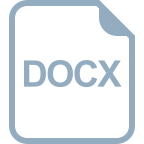
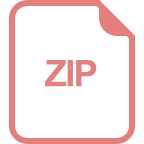
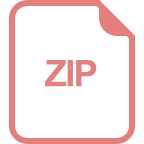
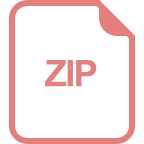
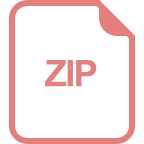
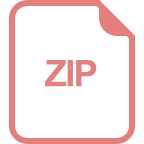
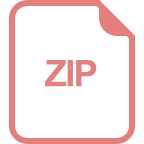
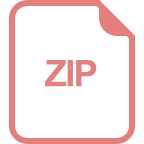