def DeepLabV3Plus(input_shape = (256,256,3), num_classes = 3): inputs = Input(shape=input_shape) # Encoder encoder_output, skip_1, skip_2, skip_3 = encoder(inputs) # ASPP (Atrous Spatial Pyramid Pooling) x = conv_block(encoder_output, 256, kernel_size=1) x = conv_block(x, 256, kernel_size=3, strides=1, dilation_rate=6) x = conv_block(x, 256, kernel_size=3, strides=1, dilation_rate=12) x = conv_block(x, 256, kernel_size=3, strides=1, dilation_rate=18) x = Conv2D(256, 1)(x) x = BatchNormalization()(x) # Decoder x = decoder(x, skip_1, skip_2, skip_3) # Output outputs = Conv2D(num_classes, 1, activation='softmax')(x) model = Model(inputs=inputs, outputs=outputs) model.compile(optimizer='adam', loss='categorical_crossentropy', metrics=['accuracy']) return model
时间: 2023-10-01 12:10:03 浏览: 93
这是一个使用DeepLabV3Plus架构的图像分割模型。它由以下几个部分组成:
1. Encoder:从输入图像中提取特征,并生成skip连接以供解码器使用。
2. ASPP(空洞空间金字塔池化):通过使用不同的扩张率(dilation rate)来捕捉不同尺度的上下文信息。
3. Decoder:使用skip连接和上采样操作将特征重新恢复到原始尺寸。
4. 输出层:使用1x1卷积将特征图映射到目标类别数量,并使用softmax激活函数进行分类。
该模型使用adam优化器,并使用分类交叉熵作为损失函数进行训练。你可以根据自己的数据集和要解决的问题调整输入形状和类别数量。
相关问题
tensorflow搭建deeplabv3+
### 使用 TensorFlow 构建 DeepLabV3+ 模型
为了使用 TensorFlow 实现 DeepLabV3+ 网络结构,可以从 GitHub 上获取预定义的配置文件和源码来简化开发过程[^2]。下面提供了一个基于 `tf.keras` 的实现方法。
#### 导入必要的库
```python
import tensorflow as tf
from tensorflow import keras
from tensorflow.keras.layers import Input, Conv2D, BatchNormalization, Activation, DepthwiseConv2D, UpSampling2D, Concatenate
```
#### 定义 ASPP (Atrous Spatial Pyramid Pooling) 层
ASPP 是 DeepLabV3+ 中的关键组件之一,用于捕捉多尺度上下文信息。
```python
def aspp_layer(x, filters=256):
shape_before = tf.shape(x)
# Image pooling branch
pool = tf.reduce_mean(x, axis=[1, 2], keepdims=True)
conv_pool = Conv2D(filters=filters, kernel_size=(1, 1), padding='same')(pool)
bn_pool = BatchNormalization()(conv_pool)
relu_pool = Activation('relu')(bn_pool)
upsampled_pool = UpSampling2D(size=(shape_before[1], shape_before[2]), interpolation="bilinear")(relu_pool)
# Atrous convolution branches with different rates
atrous_rates = [6, 12, 18]
def apply_atrous_conv(rate):
return Conv2D(
filters=filters,
kernel_size=(3, 3),
dilation_rate=rate,
padding='same',
activation=None)(x)
layers = [
Conv2D(filters=filters, kernel_size=(1, 1), padding='same')(x)] + \
list(map(apply_atrous_conv, atrous_rates)) + \
[upsampled_pool]
concatenated = Concatenate(axis=-1)(layers)
output = Conv2D(filters=filters, kernel_size=(1, 1))(concatenated)
return output
```
#### 创建完整的 DeepLabV3+ 结构
此部分展示了如何组合 ResNet50 和 ASPP 来形成最终网络架构。
```python
def deeplab_v3_plus(input_shape=(None, None, 3)):
inputs = Input(shape=input_shape)
base_model = tf.keras.applications.ResNet50(include_top=False, weights='imagenet', input_tensor=inputs)
c5 = base_model.get_layer('conv5_block3_out').output
low_level_features = base_model.get_layer('conv2_block3_out').output
low_level_features = Conv2D(filters=48, kernel_size=(1, 1), padding='same')(low_level_features)
low_level_features = BatchNormalization()(low_level_features)
low_level_features = Activation('relu')(low_level_features)
x_a = aspp_layer(c5)
x_b = UpSampling2D((4, 4), interpolation="bilinear")(x_a)
merged_feature_map = Concatenate()([x_b, low_level_features])
final_output = Conv2D(filters=256, kernel_size=(3, 3), padding='same')(merged_feature_map)
final_output = BatchNormalization()(final_output)
final_output = Activation('relu')(final_output)
final_output = UpSampling2D(interpolation="bilinear", size=(4, 4))(final_output)
num_classes = 21 # For PASCAL VOC dataset
outputs = Conv2D(num_classes, (1, 1), strides=(1, 1), activation='softmax')(final_output)
model = keras.Model(inputs=inputs, outputs=outputs)
return model
```
上述代码片段提供了创建一个基本版本的 DeepLabV3+ 模型所需的全部要素。对于特定应用案例,可能还需要调整输入尺寸、类别数量以及其他超参数设置以适应具体的数据集需求。
请详细介绍deeplabv3+的网络结构并给出deeplabv3+图像分割的代码
DeepLabv3+是Google于2018年提出的图像语义分割算法,它是基于DeepLabv3的改进版,主要针对于语义分割中存在的细节和边缘信息不够准确的问题进行了改进。相比于DeepLabv3,DeepLabv3+在特征融合和上采样方面进行了优化,使得分割结果更加精确。
DeepLabv3+的网络结构主要由三个部分组成:骨干网络、ASPP(Atrous Spatial Pyramid Pooling)模块和Decoder模块。
骨干网络使用的是Xception模型,它是一种深度可分离卷积的扩展版本,能够更好地提取图像特征。ASPP模块通过使用不同的采样率对特征图进行空间金字塔池化,能够有效地捕捉不同尺度的特征。Decoder模块主要通过上采样和跨层连接来恢复分辨率和细节信息。
以下是使用Python和Tensorflow2.0实现的DeepLabv3+图像分割代码:
```python
import tensorflow as tf
from tensorflow.keras import layers
# 定义ASPP模块
def ASPP(inputs, output_stride):
# 定义空洞卷积的采样率
rates = [1, 6, 12, 18]
# 使用不同的采样率对特征图进行空间金字塔池化
branches = []
for rate in rates:
branch = layers.Conv2D(256, 3, padding='same', dilation_rate=rate, activation='relu')(inputs)
branches.append(branch)
# 使用全局池化对特征图进行降维
x = layers.GlobalAveragePooling2D()(inputs)
x = layers.Reshape((1, 1, 2048))(x)
x = layers.Conv2D(256, 1, padding='same', activation='relu')(x)
x = layers.UpSampling2D(size=(output_stride // 4, output_stride // 4), interpolation='bilinear')(x)
# 将ASPP分支和全局池化的结果进行拼接
x = layers.concatenate([x] + branches, axis=3)
x = layers.Conv2D(256, 1, padding='same', activation='relu')(x)
x = layers.Dropout(0.5)(x)
return x
# 定义Decoder模块
def Decoder(inputs, skip_connection):
# 使用跨层连接将浅层特征图与深层特征图进行融合
x = layers.Conv2D(48, 1, padding='same', activation='relu')(inputs)
x = layers.UpSampling2D(size=(4, 4), interpolation='bilinear')(x)
x = layers.concatenate([x, skip_connection], axis=3)
x = layers.Conv2D(256, 3, padding='same', activation='relu')(x)
x = layers.Dropout(0.5)(x)
x = layers.Conv2D(256, 3, padding='same', activation='relu')(x)
x = layers.Dropout(0.1)(x)
return x
# 定义DeepLabv3+模型
def DeepLabv3Plus(input_shape, num_classes, output_stride):
# 定义输入层
inputs = layers.Input(shape=input_shape)
# 定义骨干网络
x = layers.Conv2D(32, 3, strides=2, padding='same', activation='relu')(inputs)
x = layers.Conv2D(64, 3, padding='same', activation='relu')(x)
x = layers.Conv2D(64, 3, strides=2, padding='same', activation='relu')(x)
x = layers.Conv2D(128, 3, padding='same', activation='relu')(x)
x = layers.Conv2D(128, 3, strides=2, padding='same', activation='relu')(x)
x = layers.Conv2D(256, 3, padding='same', activation='relu')(x)
x = layers.Conv2D(256, 3, padding='same', activation='relu')(x)
skip_connection = x
# 定义ASPP模块
x = ASPP(x, output_stride)
# 定义Decoder模块
x = Decoder(x, skip_connection)
# 使用双线性插值对特征图进行上采样
x = layers.UpSampling2D(size=(output_stride // 4, output_stride // 4), interpolation='bilinear')(x)
# 输出层
x = layers.Conv2D(num_classes, 1, padding='same')(x)
outputs = layers.Activation('softmax')(x)
# 定义模型
model = tf.keras.Model(inputs=inputs, outputs=outputs)
return model
# 定义输入参数
input_shape = (512, 512, 3)
num_classes = 21
output_stride = 16
# 构建DeepLabv3+模型
model = DeepLabv3Plus(input_shape, num_classes, output_stride)
# 输出模型的结构信息
model.summary()
```
在上述代码中,我们首先定义了ASPP模块和Decoder模块,然后通过这两个模块构建了DeepLabv3+模型。最后使用输入参数调用DeepLabv3Plus函数,即可得到完整的DeepLabv3+模型。
阅读全文
相关推荐
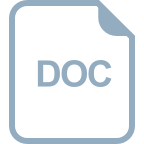
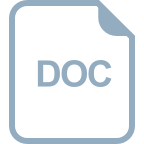
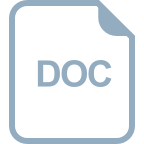
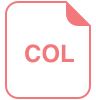
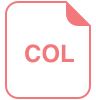
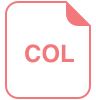
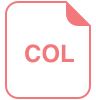
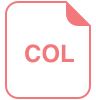
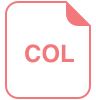
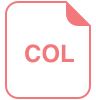
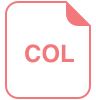
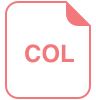
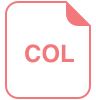
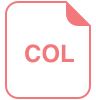


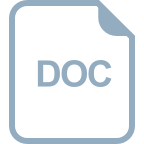