# 将调整后的数据转换为NumPy数组并进行预测 resized_test_data = np.array(resized_test_data) predictions = model.predict(resized_test_data) for i, pred in enumerate(predictions): filename = os.listdir(test_images)[i] new_filename = os.path.splitext(filename)[0] + '_prediction.png' # 构造新文件名 imageio.imwrite(new_filename, pred)怎么改
时间: 2024-02-11 08:10:06 浏览: 91
你可以使用OpenCV库来写入图像文件,可以将代码改为以下形式:
```python
import cv2
# 将调整后的数据转换为NumPy数组并进行预测
resized_test_data = np.array(resized_test_data)
predictions = model.predict(resized_test_data)
for i, pred in enumerate(predictions):
filename = os.listdir(test_images)[i]
new_filename = os.path.splitext(filename)[0] + '_prediction.png' # 构造新文件名
# 将预测结果转换为图像
pred = np.uint8(pred * 255) # 将像素值转换为0-255范围内的整数
pred = np.squeeze(pred) # 去除通道维度
pred = cv2.cvtColor(pred, cv2.COLOR_GRAY2BGR) # 将灰度图像转换为RGB图像
# 写入图像文件
cv2.imwrite(new_filename, pred)
```
这样可以将预测结果转换为RGB图像并使用OpenCV库写入图像文件。
相关问题
import cv2 import numpy as np import torch as torch from torchvision.models import densenet121 # Load the DenseNet model model = densenet121(pretrained=True) # Read the image image = cv2.imread('C:/Users/23594/Desktop/888.jpg') # Convert the image to grayscale grayscale_image = cv2.cvtColor(image, cv2.COLOR_BGR2GRAY) # Resize the image to the size of the model input resized_image = cv2.resize(grayscale_image, (224, 224)) # Normalize the image normalized_image = resized_image / 255.0 # Convert the image to a tensor image_tensor = torch.from_numpy(normalized_image).float() # Predict the key points of the person predictions = model(image_tensor) # Convert the predictions to a list of points points = [] for i in range(len(predictions[0])): points.append((predictions[0][i][0], predictions[0][i][1])) # Draw the key points on the image cv2.drawKeypoints(image, points, np.array([]), (0, 255, 0), flags=cv2.DRAW_MATCHES_FLAGS_DRAW_RICH_KEYPOINTS) # Display the image cv2.imshow('Image', image) cv2.waitKey(0)import cv2 import numpy as np import torch as torch from torchvision.models import densenet121 # Load the DenseNet model model = densenet121(pretrained=True) # Read the image image = cv2.imread('C:/Users/23594/Desktop/888.jpg') # Convert the image to grayscale grayscale_image = cv2.cvtColor(image, cv2.COLOR_BGR2GRAY) # Resize the image to the size of the model input resized_image = cv2.resize(grayscale_image, (224, 224)) # Normalize the image normalized_image = resized_image / 255.0 # Convert the image to a tensor image_tensor = torch.from_numpy(normalized_image).float() # Predict the key points of the person predictions = model(image_tensor) # Convert the predictions to a list of points points = [] for i in range(len(predictions[0])): points.append((predictions[0][i][0], predictions[0][i][1])) # Draw the key points on the image cv2.drawKeypoints(image, points, np.array([]), (0, 255, 0), flags=cv2.DRAW_MATCHES_FLAGS_DRAW_RICH_KEYPOINTS) # Display the image cv2.imshow('Image', image) cv2.waitKey(0)
这段代码是使用预训练的DenseNet模型对一张人物图片进行关键点检测,并在图片上标出关键点。它使用了OpenCV和PyTorch库。具体流程包括:
1. 导入必要的库,包括OpenCV、NumPy和PyTorch等。
2. 加载预训练的DenseNet模型。
3. 读取待检测的人物图片,并将其转为灰度图像。
4. 调整图片大小为与模型输入大小相同。
5. 将像素值归一化到0到1之间,并将其转为PyTorch的张量。
6. 对张量进行预测,得到关键点的坐标。
7. 将预测结果转为关键点的列表。
8. 在原始图片上标出关键点。
9. 显示标注后的图片。
需要注意的是,这段代码没有进行任何异常处理和参数调优,仅仅是演示了一个基本的关键点检测流程。
predictions = model.predict(test_data) # 预测结果为什么出现Input 0 of layer "model" is incompatible with the layer: expected shape=(None, 256, 256, 1), found shape=(None, 512, 512)怎么将数据调整为256*256
你可以使用OpenCV库中的resize()函数来调整图像大小。具体操作如下:
```python
import cv2
# 将图像调整为256x256大小
resized_test_data = []
for img in test_data:
resized_img = cv2.resize(img, (256, 256))
resized_test_data.append(resized_img)
# 将调整后的数据转换为NumPy数组并进行预测
resized_test_data = np.array(resized_test_data)
predictions = model.predict(resized_test_data)
```
这样就可以将测试数据调整为256x256大小,然后进行预测。请注意,如果你的测试数据是彩色图像,需要在resize()函数中指定插值方法,例如:
```python
resized_img = cv2.resize(img, (256, 256), interpolation=cv2.INTER_AREA)
```
其中,INTER_AREA表示使用像素区域关系进行像素重采样,适合缩小图像。
阅读全文
相关推荐
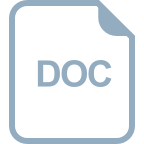
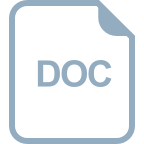
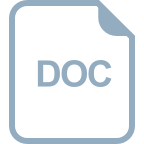
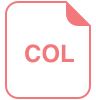
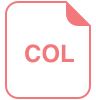
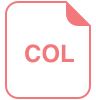
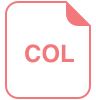
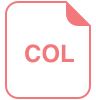
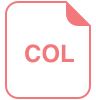
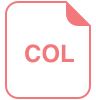
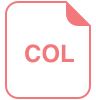
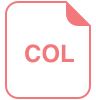
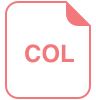
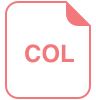
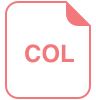

