以8数码问题和15数码问题为例实现A*算法的求解程序(编程语言不限,如Python等),要求设计两种不同的估价函数
时间: 2024-12-16 22:16:17 浏览: 7
A*搜索算法是一种启发式搜索方法,它结合了宽度优先搜索(广度优先)和最佳优先搜索(深度优先)的特点,通过评估节点的“费用”和“代价”来进行决策。在8数码问题(也称滑动拼图)和15数码问题中,目标是将数字按照特定顺序排列。
这里我们提供一个基本的Python代码框架,用于解决这两种问题,并使用两种不同的估价函数:
**1. 朴素估价函数(曼哈顿距离)**
```python
def heuristic(state, goal):
return sum(abs(state[i] - goal[i]) for i in range(len(state)))
# A*函数
def a_star(state, start, goal, heuristic_func=heuristic):
open_set = [(0, start)]
came_from = {}
cost_so_far = {start: 0}
while open_set:
_, current = min(open_set, key=lambda x: (cost_so_far[x[1]], heuristic_func(x[1], goal)))
if current == goal:
break
open_set.remove((cost_so_far[current], current))
for neighbor in expand(current):
new_cost = cost_so_far[current] + 1
if neighbor not in cost_so_far or new_cost < cost_so_far[neighbor]:
cost_so_far[neighbor] = new_cost
priority = new_cost + heuristic_func(neighbor, goal)
open_set.append((priority, neighbor))
came_from[neighbor] = current
path = []
while current in came_from:
path.append(current)
current = came_from[current]
path.append(start) # 添加起始位置
return path[::-1] # 返回路径从终点到起点
# 扩展函数(这里假设是简单的上下左右移动)
def expand(node):
possible_neighbors = [
(node[0] + 1, node[1]), # 上
(node[0] - 1, node[1]), # 下
(node[0], node[1] + 1), # 右
(node[0], node[1] - 1) # 左
]
return [n for n in possible_neighbors if is_valid(n)] # 根据实际规则判断是否有效
# ...其他游戏规则的实现...
```
**2. 模型启发式函数(欧几里得距离或汉明距离)**
替换`heuristic_func`为另一种更复杂的估价函数,比如欧几里得距离(适用于15数码问题,因为状态空间较小,可以计算完整的距离):
```python
def euclidean_heuristic(state, goal):
return sqrt(sum((state[i] - goal[i])**2 for i in range(len(state))))
a_star(..., heuristic_func=euclidean_heuristic)
```
**相关问题--:**
1. A*算法的核心思想是什么?
2. 如何评估一个估价函数的好坏?
3. 这两种估价函数在性能上会有怎样的差异?
阅读全文
相关推荐
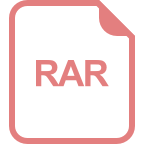
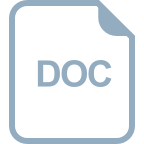
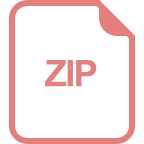





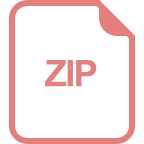
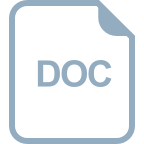
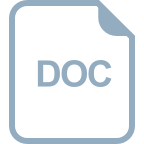
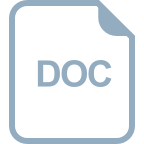
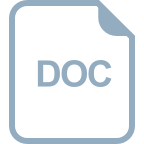
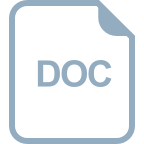
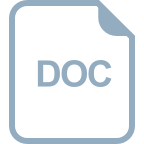
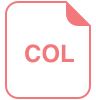
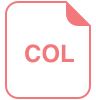
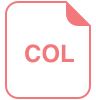
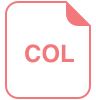